White Paper
Six Steps to Overcoming Data Pitfalls Impacting Your AI and Machine Learning Success
Data quality processing is essential to debugging data that underlies AI and machine learning predictions
In most applications we use today, data is retrieved by the source code of the application and is then used to make decisions. The application is ultimately affected by the data, but source code determines how the application performs, how it does its work and how the data is used. Today, in a world of AI and machine learning, data has a new role – becoming essentially the source code for machine-driven insight. With AI and machine learning, the data is the core of what fuels the algorithm and drives results. Without a significant quantity of good quality data related to the problem, it’s impossible to create a useful model.
The algorithms find signals in the data that are then used to make predictions and take actions. If the model is trained on different data, the predictions and actions will be different. In addition, unlocking the secrets of complex data. Frequently, AI and machine learning techniques find signals that are hidden inside variations and patterns that no human could ever detect on his or her own.
When you consider the role of data a thorny problem emerges. On the one hand, it is clear that having as much data as possible that is of high quality will make AI and machine learning algorithms work better. But it is also clear that because the signal is hidden deep inside the data and can only be revealed by algorithms, it is not always straightforward to see how we can clean such data to improve its quality without obscuring the signal.
Download this white paper to learn why the process of identifying biases present in the data, is an essential step towards debugging the data that underlies machine learning predictions and most importantly, improves data quality.
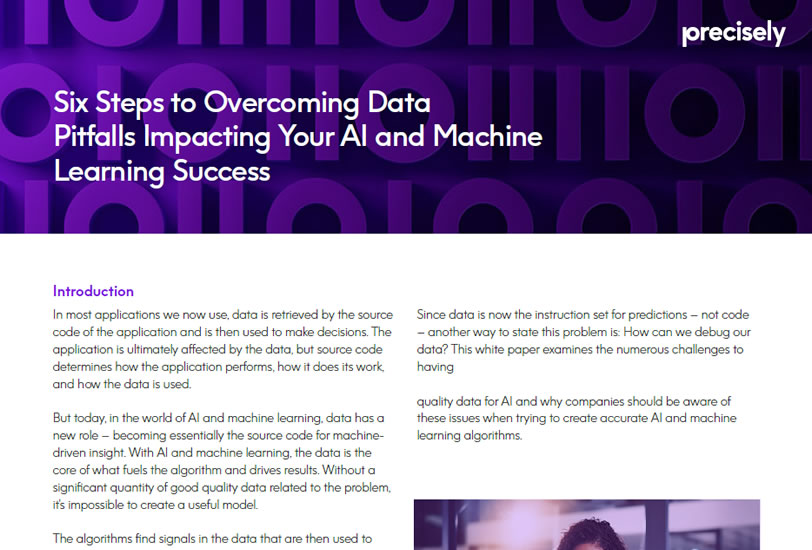