White Paper
Quantifying the Risk of Landslides: The Datasets and Approach Needed
Add precision to predictions to identify areas susceptible to landslides
Landslides are costly. Every year in the United States, the economic loss runs from $2–$4 billion. The 1998 El Niño event in the San Francisco Bay Area alone triggered over $200 million in damages.
While it’s not possible to predict the exact time and date of a landslide, you can identify which areas are susceptible to landslides. Susceptibility studies provide insights into the potential for structural damage and the loss of life.
Catastrophe models and susceptibility maps cannot solve all the problems associated with hazards, but can help in mitigating the hazards to a great extent. This paper describes a new approach developed by a team of geomatics specialists from Precisely that adds accuracy and precision to this analysis.
Knowledge of geographic and geologic conditions is necessary to understand the instability factors responsible for landslides. Slope failure is often caused by seismic activities and torrential rains. Man made activities like cut slopes, terrain farming and the scoring of river banks can also lead to slope failures. The Pacific Northwest is a particular hotspot for landslides, marked by the recent Oso landslide in Washington and constant landslides in Oregon.
A recent Precisely study focused on Oregon. This study was designed to evaluate the relative contribution of instability factors including the location of faults, folds, geology/lithology, stream proximity, land use, slope, aspect, slope roughness, elevation, terrain roughness and precipitation, plus peak ground acceleration and peak ground velocity from a Cascadia scenario event1. Landslide inventory maps including the Statewide Landslide Inventory Database for Oregon2 (SLIDO) and the Oregon Department of Transportation (ODOT) unstable slopes databases were incorporated as both training and validation datasets.
The study utilized a multivariate regression analysis, using the available parameters for regional mapping to analyze the relative contributions of various factors. These factors were analyzed as predictor variables of previously mapped landslides from existing landslide inventory maps. Afterward, an empirical model was developed using the best predictor variables.
Using these variables, we could then predict and map the probability of a landslide in susceptible areas, classifying these probabilities as very low, medium, high and very high.
Read this whitepaper to learn more.
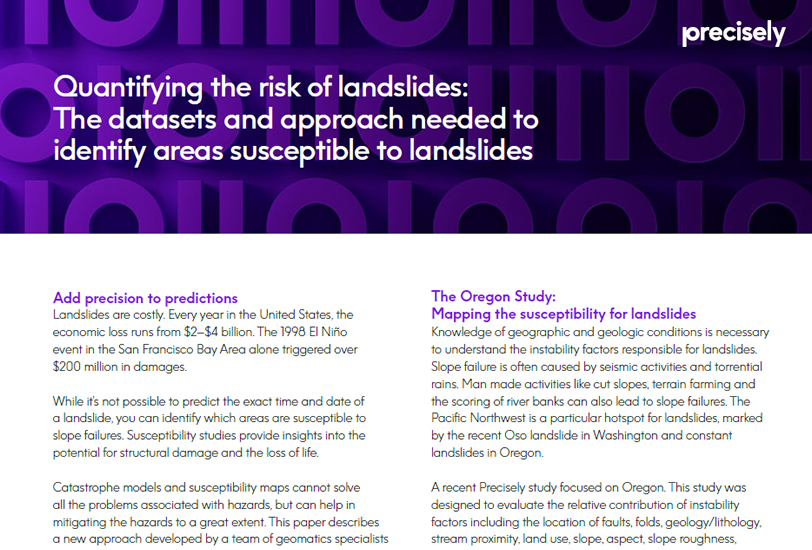