White Paper
Why Legacy and Traditional Data Is a Goldmine for AI and Analytics
The benefits of incorporating legacy data in your analytics, AI and machine learning initiatives.
Most organizations involved in advanced analytics are using big data to feed their AI projects. Many analytics teams are familiar with data in Hadoop and Spark, but are often much less fluent in legacy data sources. These legacy sources include data from relational databases, enterprise data warehouses and applications running on mainframes and hi-res server platforms.
Tools for AI and Machine Learning are targeted toward data in widely accessible modern formats, and legacy data structures are often arcane, created in an era when storage and memory were at a premium. Exacerbating the challenge of using legacy data, mainframe ops and skillsets are often specialized and siloed, and there are few ways to bridge these siloes.
This paper explains why you need to incorporate legacy data in your analytics, AI, and ML initiatives, describes steps for creating a data supply chain for legacy data, and delivers recommendations based on successful use cases.
What Is Legacy Data?
Legacy data sources include mainframes and systems like IBM i, as well as platforms running custom applications and industryspecific applications such as data historians. The category also includes relational databases and enterprise data warehouses.
There’s a popular notion that legacy platforms are outdated. Quite the opposite is the case; these platforms run much of the world’s business. Mainframes host about 70 percent of the world’s transactional data. Ninety-six of the world’s top 100 banks, 23 of the top 25 US retailers and 9 of the world’s 10 largest insurance companies run on mainframes.
Download this white paper to learn why you need to incorporate legacy data in your analytics, AI and ML initiatives and more about the steps you’ll need to take to create a data supply chain for legacy data.
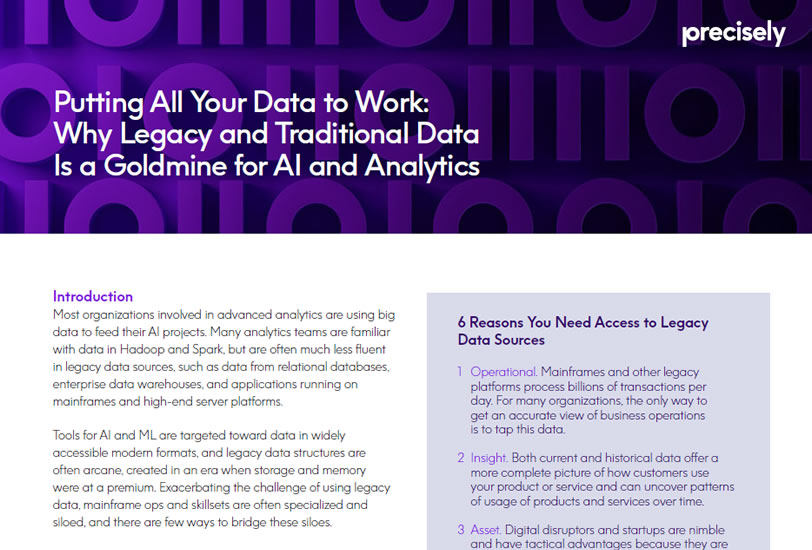