eBook
Why Metadata Management is an Essential Element of Data Governance
Lingering data usage problems
We constantly hear about the explosion of big data and how important data is to any business across any vertical. However, so many business users simply aren’t using their data because they don’t know what they have (do you have an up-to-the-minute inventory of your enterprise data?), they can’t find it (does any individual in your organization know where all of your important data resides?) or they just don’t trust it (we found it, but where did it come from and what does it mean?). If you can’t answer all of these questions definitively, then, surprise, you’re not alone!
In large organizations, data inevitably spans many systems and thus, IT has the challenging task of integrating data from various processes and systems. For example, many organizations maintain traditional and legacy systems and are expanding their data capabilities with cloud storage, ‘big-data’ Hadoop clusters, and third-party vendor data. Each of these data repositories has its own rules and requirements. The modern organization’s Data Supply Chain is massive, complex and scattered. All it takes is one change, such as a 3rd party feed switch, to impact an array of business processes. It’s no wonder organizations struggle to pinpoint the right data when they need it. Yet, these same organizations require real-time insights at the speed of business to make informed business decisions to achieve or sustain a competitive advantage.
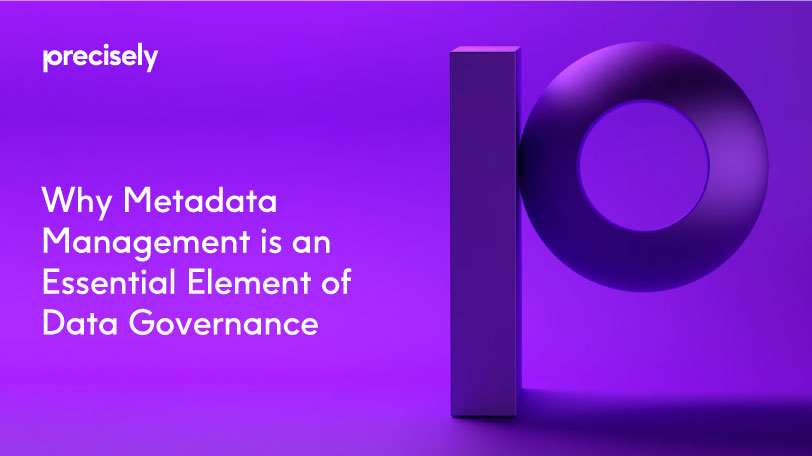
Metadata management 101
Organizations are now looking toward metadata to help solve this problem. In short, metadata is simply data about data. It can tell us various data attributes including where data resides and how to find it. Remember the days of finding a book by identifying information (aka metadata) such as an author, title, subject or date of publication? The card catalog is the first place you would go to search for something in the library. It would tell us where to find the book and how the library was organized. If a card catalog tells us how books are organized, we can think of a centralized metadata portal as a card catalog for data. Just as books reside across a library, on different floors, in different sections, etc., data is scattered across disparate systems in different formats in an enterprise. Metadata is (or at least should be) stored in a central location and used to help organizations standardize how data is located. However, before you can organize the metadata by type and understand how it functions, you need to go back and understand where metadata starts, and define your data.
Organizing and defining data
Before metadata can be used to create a glossary that tells organizations exactly where the data is located and how it should be used, we must understand the purpose and value of metadata to the business. To fully understand data, it must be triangulated—viewed from three different perspectives. Gathering metadata from these different perspectives is the only way to achieve a comprehensive understanding of how and where data lives in the business:
Physical Data Perspective: Organizations have multiple databases and each one has a code specifying where exactly each set of data lives. The metadata in this model should include information about where each system resides and where certain data sets are located within each system. Typically, this type of metadata can be automatically derived from the software that runs the physical hardware.
Logical Data Perspective: This category should contain metadata about how data travels from point A to point B. Essentially it is a map that tells organizations the data’s origins, what happens to it and where it has moved over time. The logical data model shows how data should flow through an organization and gives us a picture of where the metadata comes from and how it is transformed.
Conceptual Data Perspective: Conceptual metadata should convey the meaning and purpose of a data set from a business standpoint. It should tell users what the data means, for what purpose is it typically used, when it was created, if it is up-to-date and if it is confidential or not, etc. The conceptual data model requires human input to define the data. It also requires users to continuously update this metadata because it changes over time. For example, a data scientist might find a new use for ‘old’ data. In this situation, the use-case metadata should be updated to reflect this new purpose for data.
To effectively manage this much metadata, users must be able to go in and suggest updates with a process in place for certification or approval.
Think Wikipedia, where anyone can go in and add their two cents, but there are also controls for editing to ensure that sensitive or controversial topics are not corrupted with bad information. An effective metadata implementation will have similar controlled crowdsourcing functionality.
Once an organization views their data from these perspectives, the metadata model will start to take shape. The next step is to implement an appropriate data governance solution to organize their metadata and place it in a centralized repository.
Data governance platform
To create a comprehensive data glossary that offers business professionals transparency into the ownership dimension, organizations need to invest in a data governance platform. The platform should promote fluid communications between the data owners and the data consumers. In addition, it should have extensive collaboration capabilities for users to gain expertise on their data.
The platform should deliver an all-inclusive view of an organization’s data landscape. By delivering transparency into all aspects of an organization’s data assets, business users can gain valuable insights into not only the details of their data assets, but also quality of their data and the attendant risks associated with its use across business applications.
Like with any corporate asset, you need a current inventory and value assessment to even begin the process of tracking and securing data. Comprehensive, current metadata is essential for data protection and security.
Finally, the right platform should have automatic discovery capabilities, enabling the capture and monitoring of changes to metadata. Once changes are discovered, the technical metadata relationships are the raw material upon which a business lineage is built in order to deliver meaningful insights on data in a business context. With the proper data governance strategy, enterprises can successfully create a comprehensive data governance glossary of business-term definitions that appear in data artifacts such as reports and applications. At the heart of data governance is more than just standardized terminology, but people that oversee the data and answer questions. This is accomplished by assigning data owners and data stewards that are responsible for organizing and maintaining data definitions, usage rights and data quality parameters so that business professionals can consume data in a business context.
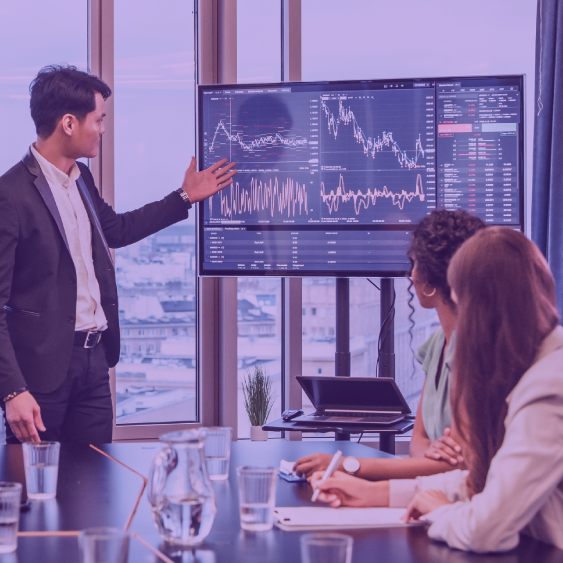
The building blocks for a metadata management foundation
Deep in the information age, trends develop and mature at lightning speed. As big data has become the norm and organizations grapple with volumes and velocity of data never before seen, the latest buzzword among data management professionals is “metadata management,” and for good reason. Organizations can use metadata to classify, manage and organize the massive amounts of diverse data collected across their enterprise. This information is crucial to both understanding and effectively deploying resources to support enterprise departments. And metadata provides crucial information to enable true predictive analytic insights.
However, managing metadata requires far more than a data governance tool. It requires a proper foundation, clear processes and the right people to execute the work. The building blocks of metadata management, then, consist of both the tools and technology that comprise a strategy, as well as the combination of people and process to create a culture of support and accountability. As with any major business initiative, there will be minds to change and challenges to overcome right from the start, which is why you can’t afford to wait.
Meeting challenges from the beginning
A typical challenge confronting businesses today as they seek to implement data initiatives is getting buy-in from upper management. Another common, and related issue, is the amount of time it can take to realize or demonstrate ROI. When investing in metadata management, there will be immediate impacts, though the full ROI will be realized over a longer span. Properly managing metadata requires persistence that few organizations will sustain unless the processes are built into the fabric of the work. But when fully implemented, the pay-off is substantial, providing organizations with increased profits and improved operational efficiencies, optimized data utilization and maximum value from data assets.
Organizations rarely have sufficient resources to staff multiple projects concurrently, and those that require specialized expertise such as a data scientist are in high demand, causing bottlenecks. Not only are people competing for resources or are spread thin across various projects, but projects that require broad participation and collaboration among divergent lines of business further undermine progress. Operations is consumed with the day-to-day business, marketing is busy creating campaigns and content, IT is juggling myriad requests and priorities from data to environments to end user support, and finance is buried in balancing the books. Getting everyone in the same room at the same time to discuss, organize and define data is a near impossibility, not to mention a low priority for most stakeholders.
It’s high time to get your team involved
So how do you get each department not only involved with, but invested in, metadata management? Each department needs to realize that proper metadata management will help them find the “gold nuggets” buried in organizational data that can lead to better business decisions and greater profitability. If finding the right patterns, trends, insights and actionable information isn’t enough incentive to get employees involved with metadata management, then upper management will have to leverage every resource they can to instigate change. This can include creating visibility for metadata work, carving out career paths, and being creative to build short term and long term incentives that are consistent with the organization’s culture and policies.
Once an organization has both budget and buy-in from upper management, and has laid the groundwork for what metadata management can achieve with employees and departments across the enterprise, they can begin building a metadata management foundation.
Laying a metadata management foundation
When starting a metadata management program, there are three fundamental steps that must be handled by people within or outside the organization. They are:
- Design of the model and implementation of the tool: every business is different, and each one needs to ensure that their model is customized to fit their specific needs. The architect of the metadata model must guarantee that organizations are collecting the right inventory of metadata to solve their individual business problems. In addition, the tool must also be configured to meet ongoing business needs. This step should be handled by an internal specialist or an outside consultant with experience working with all types of metadata. Ideally, the architect reports directly to the chief data officer (CDO).
- Oversight and management of the metadata: as with any project, there needs to be an assigned project manager to ensure everything is going as planned. In this case, organizations need a manager who understands the metadata model to guarantee that once the tool is designed, that the right information is being collected and properly maintained and that the work is done correctly and on schedule. The manager role is not highly technical, but can see to it that that the metadata model is networked together to promote usability in design through implementation and beyond.
- Acquisition of metadata: there are three types of metadata that need to be collected. The first two types are physical and logical metadata. Physical metadata deals with the location of the data and logical deals with the flow of data through an enterprise. The data lineage of logical metadata provides critical information on where data is coming from and going to, and both of these types are technical in nature. For this reason, collection of these types of metadata requires an analyst with technical skills. Much of the physical and logical metadata can be refreshed automatically once it has been initially collected. Conceptual metadata, on the other hand, deals with the meaning and purpose of data as understood from a business perspective. It is the “data” in people’s heads, and must be collected from actual people within each line of business. The metadata architect can oversee this effort, but it needs to be a strategic and collaborative effort. Whoever is tasked with collecting conceptual metadata will have to prioritize and plan for what metadata to target first, to target quick acquisition of high value information. It involves a process of one-on-one, small group, or larger workshops with people from across different lines of business, and the collection and documentation of their business definitions to make sure that discrepancies are known and documented so that bad assumptions about data do not lead to bad decisions.
Once an organization has an established a metadata management foundation, business and technical users will be able to quickly find data repositories and details on its lineage and reliability – in other words, where the data came from, how it got there, which transformations it has undergone, its level of quality and its relationship to other data and reports.
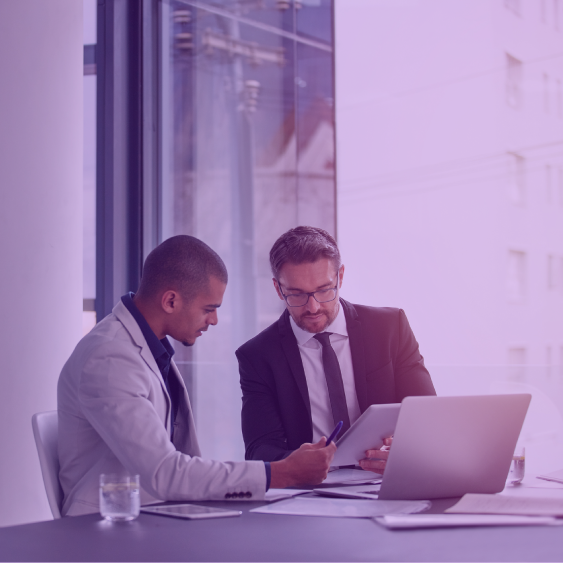
Building a metadata business case successfully
Metadata is quickly becoming the next big sensation in data management, and will be crucial for the success of big data projects this and beyond. Metadata contains all of the information that is vital to both understanding and effectively deploying data across organizations – information such as meaning and purpose, lineage, utilization and more. It is critical in enterprise data environments to support effective data governance, ensure regulatory compliance and meet a growing inventory of data management demands.
We discussed how to extract value from metadata and how organizations can leverage their metadata to optimize data initiatives. However, it is unlikely that upper management will invest in metadata management technologies unless they are certain that they will see a significant return on investment (ROI). To help demonstrate that much needed ROI, this post will discuss how to make a business case for metadata and demonstrate value.
Building a metadata business case
Businesses have one fundamental concern—their bottom line. What executive leadership may not realize is that investing in metadata can improve their bottom line by increasing operational efficiency, optimizing data security and maximizing the value of their data. Below are 4 key capabilities that demonstrate the importance of metadata and help build a metadata business case.
Discovery: Finding individual data sets in a big data environment can be a challenging proposition, but a robust central repository of metadata enables organizations to quickly and easily search and discover the data they need. By defining and tagging data sets with metadata, it becomes easier to find and to confirm the data is valid for a specific use. This improves operational efficiency, allowing organizations to more effectively leverage their data.
Impact Analysis: Being able to locate and understand the quality of your data is critical, but metadata can take this a step further. By using metadata, organizations can see how data is related and the impact that changing that data may have on other data sets. For example, if a user is searching for a business term such as “customer identification number,” an impact analysis can tell the user what other datasets, use cases and subject areas are related to that term. Furthermore, they can determine the impact to the organization if that data element should be deleted, moved, or changed in some meaningful way. For example, if you changed the data type of a telephone number from a string to numeric, it might save some storage space, but it might break 10 algorithms across the organization. Having this information at your fingertips gives you a real view into the reach and criticality of data, and will improve operational efficiency, ultimately saving time and money.
Inventory and Assessment: Data breaches are a virtual inevitability in business today, but metadata can help assess the damage and prepare organizations to respond immediately and appropriately. Metadata can be used to classify and rank data sets based on their security risk, to ensure the potential impact of a breach is quickly understood and duly addressed. For example, Social Security numbers comprise highly sensitive data, however, without an associated name, they are just a sequence of 9 digits. If a hacker attains access only to social security numbers, it requires a different response than if they accessed full customer records with Social Security numbers as well as associated names and dates of birth. Having an up to date inventory of data before a hack or a leak can save a company from unnecessary customer mistrust and damage to the brand – and ultimately, bottom line impact.
Certification: Every organization needs high quality data for big data projects, so it’s important to have an effective and transparent certification process. If an organization is selling data to a third party and wants to receive maximum reimbursement, it will need to ensure the quality and reliability of the data.
Metadata can be used to create data quality scores, so the quality of data can be quantitatively verified. Not only can organizations get maximum return on their data, but they can also feel confident in the quality of the data they are using internally.
A sound metadata management strategy can pay dividends, but to effectively leverage metadata, organizations will need to implement a strong, robust data governance platform.
Data governance
To leverage metadata, organizations will need a comprehensive data governance solution that delivers a complete view of an organization’s data landscape. The platform should include interactive data visualization and lineage capabilities, and deliver transparency into all aspects of an organization’s data assets. It should also have automatic discovery capabilities, enabling the capture and monitoring of changes to metadata. Once changes are discovered, the technical metadata relationships may be investigated to deliver meaningful insights on data for better business decisions.
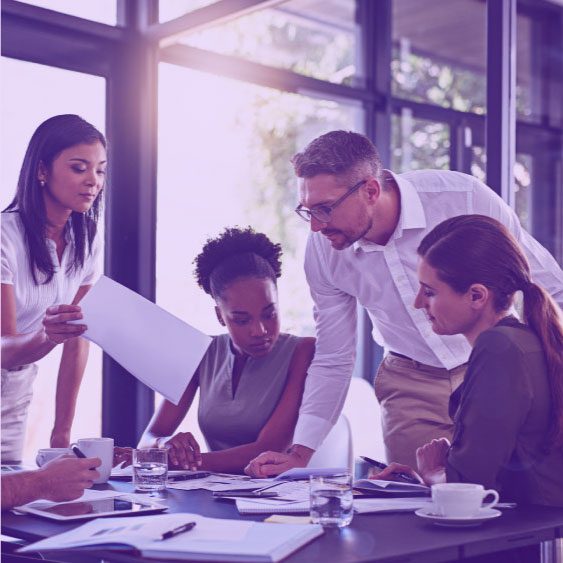
Extracting metadata value through data governance
Big data is no longer a new phenomenon; it is now just the norm, with millions of organizations across the world having started a big data implementation. However, not all of them are successful. Today, many organizations struggle to generate value from the huge amount of data they ingest, making it difficult to access, use and analyze the data that might best meet their needs. In fact, a recent survey by NewVantage Partners found that “more than 85% of respondents report that their firms have started programs to create data-driven cultures, but only 37% report success thus far.”
Organizations are now realizing that they must rely on metadata to classify, manage and organize the massive amounts of diverse enterprise data found across the organization. Metadata shows business and technical users where to find information in data repositories while providing details on where the data came from, how it got there, which transformations it has undergone, its level of quality and its relationship to other data or reports.
Best practices with metadata starts at the point where the data enters the organization. With so much differing information on the internet, we’ve highlighted the most common types and functions of metadata. By understanding what these mean, organizations can begin to build systems that allow advanced data management with a demonstrable ROI.
Types of metadata
Metadata allows organizations to describe and document the data, but most organizations struggle to implement metadata solutions. In order for metadata to help an organization to better understand, track and retrieve data, they must first learn how metadata itself can be effectively structured. This begins with understanding the three different types of metadata.
Physical Metadata: This is the type of metadata that deals most directly with the physical location and storage of data. It consists of technical metadata that can be automatically populated by the database or by any technical application that moves, changes or stores data.
Logical Metadata: This type of metadata concerns the design of data flows through the system. This could include schema or other design documents that map out how data is supposed to go from intake to end consumption by the data analyst or other data consumer. This metadata can be captured from data modeling software or from systems architects.
Conceptual Metadata: This type of metadata deals with the meaning and purpose of data as understood from the business perspective. It can include the typical uses of data, and how particular datasets are used in business processes. Most of this information must be captured from the minds of business users.
These three types of metadata represent three vantage points from which to view and understand data. It is necessary to acquire and keep refreshed all three types of metadata in order to take full advantage of your data assets. Now that we understand how metadata needs to be managed, we need to look at how metadata is used.
Functions of metadata
Metadata can be used to summarize basic information to advanced functionality. Some of the most common examples of how metadata is used includes search, browse, syndication, access permissions and more. More advanced usage includes:
- Resource discovery: using relevant criteria metadata can be used to find data resources, bring similar resources together, distinguish unrelated resources and give the location of certain data sets.
- Organizing data resources: metadata can be used to organize data files and links from a wide variety of data sources based on their topic.
- Promote and simplify software exchange: data resources across any database or network can be searched seamlessly using metadata.
- Digital identification: using a file name or URL, metadata can be used to find the location of any digital object.
- Archiving and preservation: metadata is crucial for ensuring that data resources will continue to be accessible and usable. Metadata or information about a data set’s lineage is a key element in archiving and preservation.
Metadata management is no easy task with plenty to consider. Organizations need to ensure metadata is stored where it can be accessed and indexed so it can easily be found. The quality of the metadata needs to be consistent so all users can trust it and the same data needs to be kept up-to-date over time. So how do you create, maintain, update, store, publish and handle metadata? That’s where a strong data governance strategy can help.
Data governance
To leverage metadata, organizations will need to operationalize data governance by employing comprehensive data governance that delivers a complete view of an organization’s data landscape. The solution should include interactive data visualization and lineage capabilities and deliver transparency into all aspects of an organizations data assets.
In addition, the solution should have automatic discovery capabilities, enabling the capture and monitoring of changes to metadata. Once changes are discovered, the technical metadata relationships may be investigated to deliver meaningful insights on data. With the proper data governance platform, enterprises can empower their data community to use metadata to help jump start their big data initiatives.
The real value comes to light when you can take the technical lineage and translate it into a business lineage – this is one of many ways to capitalize on metadata value by pairing it with data governance.
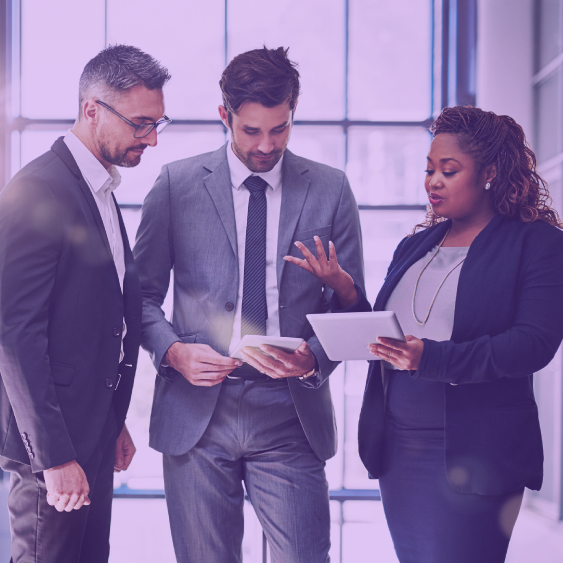
Building the framework for successful data management
Data-driven organizations know how to leverage their data as a strategic asset to optimize business processes, improve decision making, enhance the customer experience and increase revenue. But leveraging these assets is about far more than just managing data, it’s about building a culture committed to maximizing data value, where stakeholders are engaged and business users are empowered to seek out data to augment business strategies and objectives.
A comprehensive data management strategy should include a foundation of data governance and metadata management to promote data understanding, accessibility, usability and utilization. The underlying culture that makes such a strategy successful requires a partnership between business and IT, accountability among data owners and stewards and cooperation and collaboration across an enterprise to gather and maintain critical information such as conceptual metadata. All of this should enable business users to easily locate and apply data to business problems, turning that raw data into actionable insights.
However, building this culture and becoming a data-driven organization doesn’t happen overnight. There are many steps business leaders must take to implement metadata management. In a previous blog, we focused on the building blocks of metadata management. In this blog, we’ll examine how organizations can cultivate a data-driven culture.
Solid data management must begin with the right tools, but it is the combination of technology, people and processes that enable enterprise-level excellence. The world’s finest hammer may as well be a paperweight without a skilled craftsman to wield it, which is why data management success starts with the right team.
Creating a data-driven culture starts at the top
As anyone who has tried to implement a data management solution will tell you, without executive buy-in and budget, the project is dead in the water. Senior management needs to understand and promote the value of data assets and the importance of building a data-driven culture, and demonstrate that commitment as a data management team is built. Leadership needs to hire the right expert who can evangelize and oversee the data strategy for the entire enterprise. Many organizations today tap a Chief Data Officer (CDO), while others leverage data consultants to act as a de facto CDO.
Immediately under the CDO should be a senior director to act as the head of enterprise adoption. Their responsibilities include integration, adoption and compliance for new data policies, standards, analytical methods and various capabilities recommended by the CDO. Success in this position requires extensive experience in change management, and a deep understanding of technology development lifecycles.
Next, organizations should enlist a technically savvy senior manager to take on the role of data engineering manager. This role is responsible for leading teams that build high performance, scalable data solutions to meet the needs of data creators, managers and consumers. This person may also manage the data platform and work with a variety of teams and individuals, including product engineers, product managers, designers, analysts and data scientists to understand their data supply chain needs and develop innovative solutions for data ingestion, preparation and delivery.
To round out the team is the data evangelist. This position can be filled by a data analyst experienced in publicizing and energizing the work of others. This job is essential in driving data knowledge participation to discuss, organize and define data from diverging lines of business. There are a variety of approaches the data evangelist can take, but the main goal is to spread broad data knowledge and encourage participation from and collaboration among different departments.
Facilitating Data Knowledge Participation
Among the data team, the data evangelist is essential to creating a data-driven organization, because they are likely spear-heading organizational efforts to gather conceptual metadata. The data evangelist, or their designated team members, is tasked with gathering that data which resides within the minds of employees across varied departments and lines of business. To gather this conceptual metadata across business functions, they must interview subject matter experts and work cross-functionally with various business and technology teams.
There are, however, many barriers to gathering this knowledge. First, there is simply a matter of logistics. Gathering experts from various departments together in the same room at the same time, in order to share their expertise, is no simple task. Then there is the general level of skepticism that comes with the introduction of any new process or technology. There will be change averse employees, and those who doubt the efficacy of the new procedures. It can take time to create converts among employees. Lastly, there are those employees who feel threatened by shared knowledge. They guard the knowledge they have as a sort of shield protecting them and their position. These employees will not readily give up what they perceive as a source of their power.
However, knowing these obstacles exist is the first step to overcoming them. The data team needs to find innovative ways to engage users and encourage their involvement. One approach a data evangelist may utilize to motivate participation is by implementing an internal marketing campaign to get people excited about data and gain their buy-in. For example, the data evangelist can craft various marketing materials to connect people with data insights to show how data specifically benefits their team. They can also take a more entertaining approach and use “gamification” or competitions to increase participation and interest, or train the team using real-world examples to demonstrate how data knowledge is relevant to their work.
If internal marketing doesn’t do the trick, data evangelists can work with the human resources (HR) department to further engage employees. HR can provide special recognition for data engagement, like acknowledgements on ID tags and email signatures, prestige giveaways, or exclusive perks like time off, bonuses, free lunches, etc. to help spur participation.
In a data-driven culture where participation is encouraged, rewarded and respected, teams are more likely to take an active role in data analysis to make smarter business decisions, enhance strategies and fine-tune objectives to gain a competitive advantage and increase revenue.