eBook
Seven Metrics to Assess Data Quality within your Data Governance Framework
Read this ebook to explore 7 metrics for data quality assessments within your data governance framework and avoid exposing your organization to unnecessary risk.
Introduction
Your business depends on accurate data. Inaccurate, incomplete, inconsistent data diminishes the quality of customer experiences, hinders operational efficiency, and threatens regulatory compliance, ultimately exposing your organization to unnecessary risk, instead of giving you the information you need.
Data governance initiatives seek to solve these problems, and to provide the business with trusted, high quality data that will boost marketing effectiveness, customer satisfaction, and ultimately revenue. Data governance tools like the Data Governance service in the Precisely Data Integrity suite provide a broad set of capabilities to identify and manage datasets. Sustainable data governance requires a solid foundation of quality data. It requires the right people, the right processes, and the right technology to turn raw, untamed data into valuable business insights.
Data quality refers to the ability of a set of data to serve an intended purpose. Low-quality data cannot be used effectively to do the thing with it that you wish to do. Data observability enables a big picture understanding of the health of an organization’s data through continuous AI/ML-enabled monitoring, detecting anomalies throughout the data pipeline and preventing data downtime. Data quality, data observability and data governance share a ‘symbiotic relationship’. Data governance needs appropriate data quality and data observability tools to not only clean the raw data, but to illustrate data errors, peculiarities and issues, in order to help compile the best standards and monitor the data quality against policies for critical data elements over time. Precisely’s industry leading data validation and data quality monitoring capabilities are an integrated component of the Data Governance service in the Precisely Data Integrity suite.
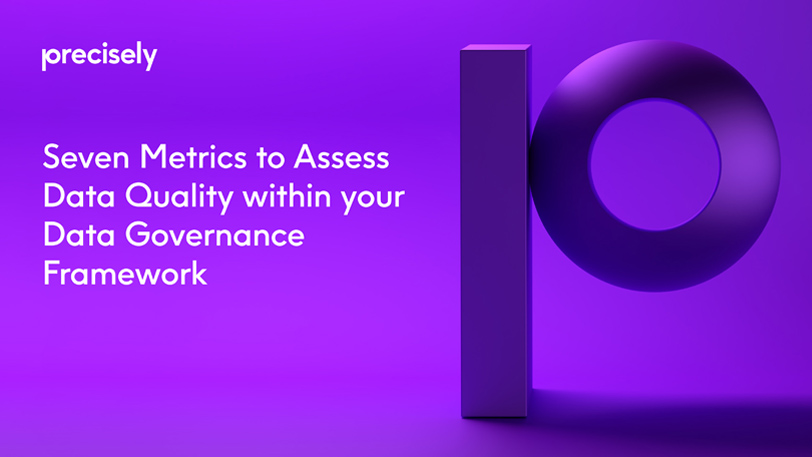
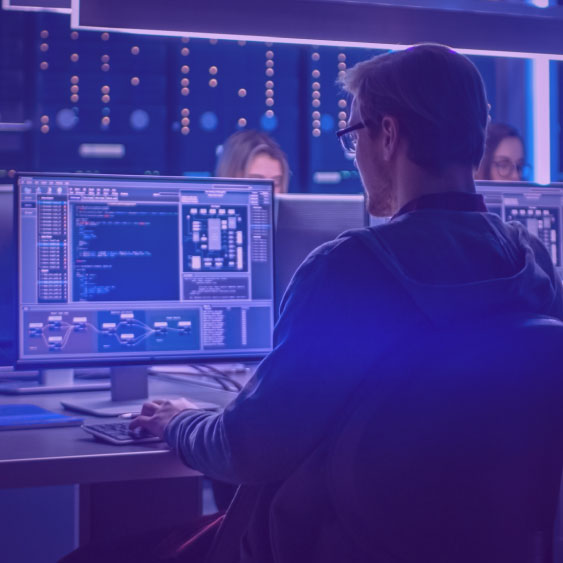
Assessing data quality
There are lots of good strategies that you can use to improve the quality of your data and build data best practices into your company’s DNA. Although the technical dimensions of data quality control are usually addressed by engineers, there should be a plan for enforcing best practices related to data quality throughout the organization.
After all, virtually every employee comes into contact with data in one form or another these days. Data quality is everyone’s responsibility. Assessing data quality on an ongoing basis is necessary to know how well the organization is doing at maximizing data quality. Otherwise, you’ll be investing time and money in a data quality strategy that may or may not be paying off.
To measure data quality – and track the effectiveness of data quality improvement efforts – you need, well, data. What does data quality assessment look like in practice? There are a variety of data and metrics that organizations can use to measure data quality. We’ll review a few of them here.
7 metrics to measure data quality
The most obvious and direct measure of data quality is the rate at which your data analytics processes are successful. Success can be measured both in terms of technical errors during analytics operations, as well as in the more general sense of failure to achieve meaningful insight from a dataset even if there were no technical hiccups during analysis.
The fewer data quality problems you have to start with, the faster you can turn your data into value. The main purpose of a data quality plan is to enable effective data analytics, so fewer analytics failures mean you are doing a good job on the data quality front.
Below are seven metrics to help you get started on your data quality plan:
1. The ratio of data to errors
This is the most obvious type of data quality metric. It allows you to track how the number of known errors – such as missing, incomplete or redundant entries – within a data set corresponds to the size of the data set. If you find fewer errors while the size of your data stays the same or grows, you know that your data quality is improving.
2. Number of empty values
Empty values – which usually indicate that information was missing or recorded in the wrong field — within a data set are an easy way to track this type of data quality problem. You can quantify how many empty fields you have within a data set, then monitor how the number changes over time.
3. Data transformation error rates
Problems with data transformation – that is, the process of taking data that is stored in one format and converting it to a different format – are often a sign of data quality problems. Your data transformation tools will struggle to work effectively with data that they encounter in unexpected formats, or that they cannot interpret because it lacks a consistent structure. By measuring the number of data transformation operations that fail (or take unacceptably long to complete) you can gain insight into the overall quality of your data.
4. Amounts of dark data
Dark data is data that can’t be used effectively, often because of data quality problems. The more dark data you have, the more data quality problems you probably have.
5. Email bounce rates
If you’re running a marketing campaign, poor data quality is one of the most common causes of email bounces. They happen because errors, missing data or outdated data cause you to send emails to the wrong addresses.
6. Data downtime
Are your users frequently running into a situation where they cannot access accurate data they need, when they need it, for timely decision making? When issues such as data drift, anomalies and outliers occur then users are often unable to use the data for important business decisions.
7. Data time-to-value
Calculating how long it takes your team to derive results from a given data set is another way to measure data quality. While a number of factors (such as how automated your data transformation tools are) affect data time-to-value, data quality problems are one common hiccup that slows efforts to derive valuable information from data.
Summary
The metrics that make the most sense for you to measure will depend upon the specific needs of your organization, of course. These are just guidelines for measuring data quality. Precisely offers data quality products that seamlessly integrate with the Precisely Data Integrity Suite to enable users to understand the quality of their data.”
The importance of data quality, and the amount of data you have to process will only increase with time at most organizations. Continually improving your ability to maintain data quality will help keep you prepared for the data analytics requirements of the future.