eBook
Preparing Your Data for AI with Data Integration
Introduction
Data is the fuel that powers artificial intelligence (AI). However, if data isn’t high quality, accessible, and integrated, it can introduce biases and inaccuracies that can harm the business.
Data integration is a critical step in preparing your data for AI success. By bringing together data from various sources and formats into one unified view, organizations can easily access and analyze all their data, no matter where it comes from or how it’s organized. Data integration also ensures that data is accurate, complete, and reliable, which are essential qualities for successful AI initiatives.
This checklist provides a quick overview of the key steps and considerations for data integration.
Data Integration for AI
Define your data integration goals and scope
Assess your current data landscape and identify data sources
Choose the right data integration approach, partners and tools
Design and implement a data integration architecture and pipeline
Ensure data quality and governance throughout the process
Monitor and optimize data integration performance and outcomes
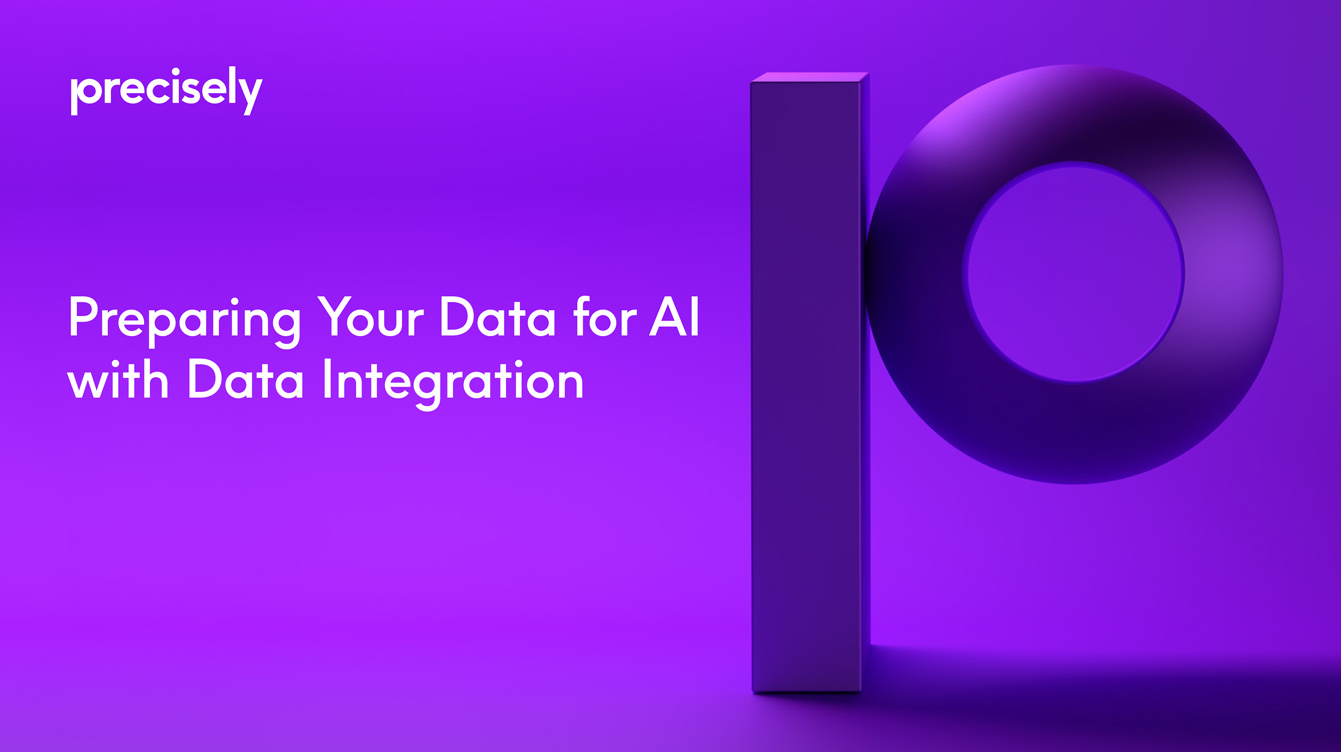
Step 1: Define data integration goals and scope
There’s no one solution for data integration; different AI use cases may require different approaches and techniques. Defining your data integration goals and scope based on your specific AI objectives will also define the scope and boundaries of your project, allowing you to share expected deliverables, timelines, goals and expectations with stakeholders and team members.
For step one, ask these questions:
- What are the business problems or opportunities we want to address with AI?
- What are the expected outcomes and benefits of our AI project?
- What are the data sources and types that we need to integrate?
- What are the challenges and risks?
- What are the success criteria and metrics?
- What are our resources and budget?
- What tools and partners will we work with?
Step 2: Assess your current data landscape and identify data sources
Assessing your current data landscape and identifying the data sources to integrate will help you understand the availability, accessibility, and quality of your data, as well as find and prioritize any gaps and issues that need to be addressed.
Tasks to perform at this stage are:
- Conduct a data inventory and audit to identify and document all data sources and types that are relevant for your AI project
- Assess the data quality and completeness of each data source, and identify any data quality issues, such as missing, inaccurate, inconsistent, or duplicate data
- Assess the data accessibility and security of each data source, and identify any data access issues, such as data silos, data fragmentation, data privacy, or data protection
- Assess the data compatibility and interoperability of each data source, and identify any data integration issues, such as data format, data structure, data schema, or data semantics
- Prioritize the data sources and types that are most critical and valuable for your project, and determine the data integration order and frequency
Step 3: Consider your data integration approach and tools
Data integration is a combination of methods and technologies that can change based on your data’s sources, types, formats, and environment. In this step, you’ll choose the best way to integrate the data generated and leveraged by your business. Identify the methods, tools, and partners that match your project’s complexity and volume. You’ll also compare and select the solutions that offer the best functionality, performance, and cost-effectiveness.
Some of the factors to consider are the:
- Architecture and design, such as centralized, decentralized, or hybrid
- Technique and method, such as extract-transform-load (ETL), extract-load-transform (ELT), change data capture (CDC), or data virtualization
- Mode and frequency, such as batch, real-time, or streaming
- Platform and tool environment, such as cloud-based (public, private, or multi-cloud), on-premise, or hybrid
- Functionality and capability, such as data ingestion, data transformation, data cleansing, data enrichment, data mapping, data validation, data delivery, or data monitoring
- Scalability and performance, such as data volume, data velocity, data variety, data latency, data throughput, or data reliability
- Requirements that must be met for governance, stakeholder data access, and compliance
- Partnerships required for proper implementation of data integration patterns and application of data
Step 4: Design and implement a data integration architecture and patterns
The next step is to design and implement a data integration architecture and patterns that can execute and deliver your data integration goals. A data integration architecture is the blueprint that defines how your data sources are connected, transformed, and delivered to your AI applications. A data integration pattern is the workflow that implements the data integration architecture and executes the data integration tasks and processes. With these steps, you’ll also be able to ensure that your data integration pattern is functional, reliable, and secure, and has the data quality necessary for AI success.
Steps for this stage are:
- Define the data integration inputs and outputs, such as data sources, types, formats, and destinations
- Define the data integration transformations and rules, such as data cleansing, enrichment, mapping, and validation
- Define the data integration flows and sequences, such as data ingestion, delivery, and synchronization
- Define the data integration controls and standards, such as data quality, governance, and security
- Implement the data integration pattern using the selected data integration tools and platforms
- Test and validate the data integration pattern using sample data and scenarios
Step 5: Ensure data quality and governance throughout the data integration process
Data quality and governance are essential for data integration success, especially for AI applications that rely on accurate, complete, and trustworthy data. Data quality refers to the degree to which your data meets the expectations and requirements of your AI project. Data governance refers to the policies and procedures that ensure the proper management and usage of your data. It’s essential to ensure data quality and governance throughout the data integration process, from data source to data destination to enhance the reliability, usability, and value of your data. This process also improves compliance with data regulations and standards that apply to your data and industry.
Best practices to follow at this stage are:
- Establish data quality and governance roles and responsibilities, such as data owners, data stewards, data analysts, or data consumers
- Determine data quality and governance metrics and indicators, such as data accuracy, completeness, consistency, timeliness, or relevance
- Set up data quality and governance rules and standards, such as data definitions, data formats, data schemas, data values, or data lineage
- Implement data quality and governance tools and techniques, such as data profiling, data cleansing, data enrichment, data validation, data auditing, or data monitoring
- Monitor and measure data quality and governance performance and outcomes, such as data quality reports, data quality dashboards, data quality alerts, or data quality feedback
- Improve and optimize data quality and governance processes and practices, such as data quality improvement plans, data quality remediation actions, data quality best practices, or data quality lessons learned
Step 6: Monitor and optimize data integration performance and outcomes
Data integration is an ongoing process that requires continuous monitoring and optimization. As your data sources, types, and volumes change over time, so do your data integration needs and challenges. Monitoring and optimizing your data integration performance and outcomes ensures your data integration pipeline is delivering the expected value and benefits. This process also allows you to identify and leverage the data integration opportunities and innovations that can enhance your AI capabilities and competitiveness.
Steps for this stage are:
- Monitor and measure your data integration performance and outcomes, such as data integration speed, efficiency, reliability, or quality
- Identify and analyze your data integration issues and bottlenecks, such as data integration errors, failures, delays, or anomalies
- Implement and test your data integration improvements and optimizations, such as data integration enhancements, upgrades, or fixes
- Review and evaluate your data integration results and feedback, such as data integration reports, dashboards, alerts, or surveys
- Update and refine your data integration goals and scope, such as data integration objectives, deliverables, or timelines
- Document and communicate your data integration learnings and best practices, such as data integration documentation, training, or knowledge sharing
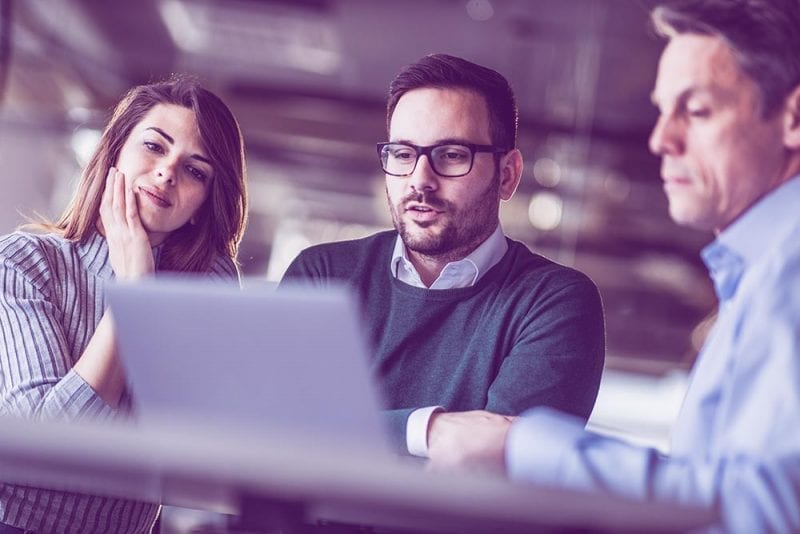
Conclusion
Data integration is a keystone of AI success. By integrating data from different sources and formats into a unified and accessible data ecosystem, you can prepare your data for AI deployment. Data integration also ensures that your data is accurate, complete, and trustworthy, which are vital qualities for AI applications that rely on data-driven insights.
This checklist will help you streamline your data management practices, identify and mitigate risks associated with data integration, prepare your data infrastructure for AI deployment, and gain insights on how to leverage data integration for AI success and innovation.