eBook
How "Good Enough" Quality is Eroding Trust in Your Data Insights
Explore key data quality insights from data professionals in the data quality survey. Read this eBook to explore key highlights from the survey and take a deeper look at the full survey results.
Survey background
Precisely’s Enterprise Data Quality Survey explores the challenges and opportunities for organizations looking to bring data insights across the enterprise as data volumes grow and new technologies emerge.
Respondent profile
Precisely polled 175 respondents, 69 percent of whom work for organizations with over 1,000 employees. Participants represented a range of industries, with the largest percentage coming from Financial Services (25%), as well as a range of positions, ranging from CDO to Data Analyst, with the majority in data-focused roles (29%).
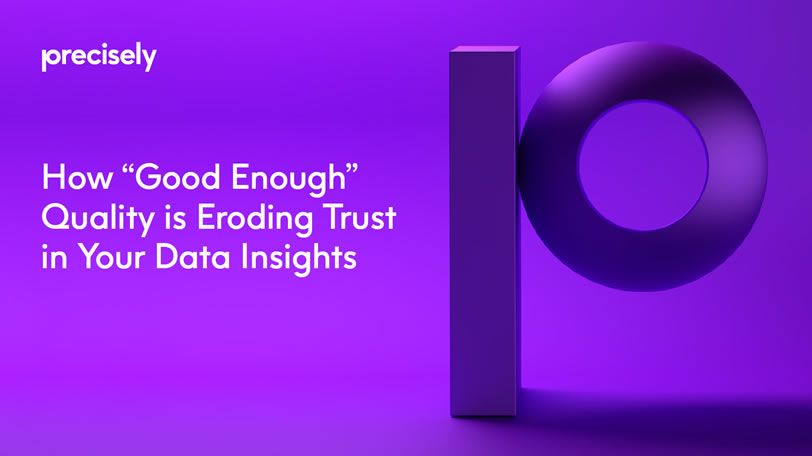
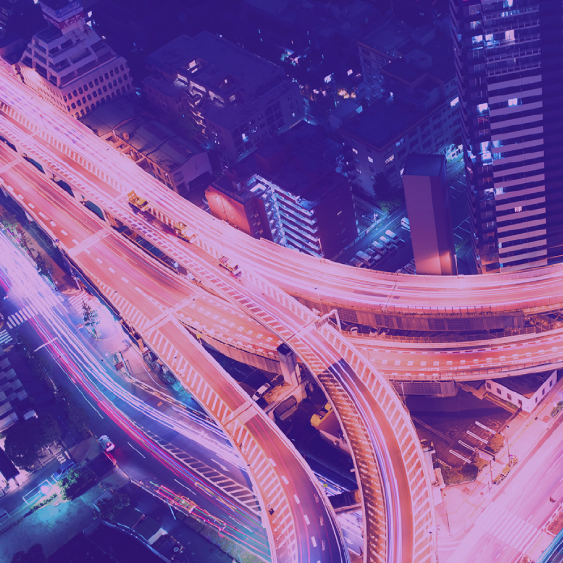
Good data isn’t good enough anymore
There is a disconnect around understanding, confidence, and trust in the data and how it informs business decisions.
72 percent responded that the quality of the data used to run their business was good or better and 69 percent stated their leadership/ c-suite trust data insights enough to inform business decisions on them. Yet, they also reported that only 14 percent of stakeholders had a very good understanding of the data and that less than 60 percent of the data was well understood by stakeholders.
More than 70 percent also reported that sub-optimal data quality negatively impacted business decisions, and almost half found that untrustworthy results or inaccurate insights from analytics were due to a lack of quality in the data fed into systems such as AI and machine learning.
Data quality is a top challenge for machine learning
Poor data quality is enemy number one to the widespread, profitable use of machine learning. The phrase “garbage-in, garbage-out” has a multiplier effect with ML — first in the historical data used to train the predictive model and second in the new data used by that model to make future decisions.
With almost half reporting that untrustworthy results or inaccurate insights from analytics were due to a lack of quality in the data fed into systems such as AI and machine learning, it’s not surprising that “many sources of data” (69%) and “volume of data” (48%) are among the top 3 challenges companies face when ensuring high quality data.
3/4 of respondents also identified as having challenges profiling or applying data quality to large data sets.
A Wall Street Journal article revealed a recent report by Forrester Research Inc. found data quality a top challenge for AI projects and that “companies pursuing such projects generally lack an expert understanding of what data is needed for machine-learning models and struggle with preparing data in a way that’s beneficial to those systems.
Understanding data across the organization
How well do you (or other key stakeholders) understand the data that exists across your organization?
Very Good
Understanding
Good
Understanding
Partial
Understanding
Minimal
Understanding
Very Little or No
Understanding
Defining “good” understanding of data
If you answered, Very Good or Good, what percentage of your data is well understood by you/key stakeholders?
Greater that 70%
70%-50%
50%-30%
30%-10%
10% or less
Data attributes lacking visibility
Of those who responded that had partial, minimal or very little understanding of their data, the top three attributes respondents lacked visibility into were:
- Relationship between data sets
- Completeness of data
- Validation of data against defined rules
Use of data profiling tools
Less than 50% of respondents take advantage of a data profiling tool or data catalog where insight may be centrally provided for broad access.
Instead, respondents rely on other methods to try to gain understanding of data, with more than 50% of respondents using SQL queries or similar and over 40% using a BI tool.
Only 17% are profiling data manually.
Profiling large data sets
3/4 of respondents identified as having challenges profiling or applying data quality to large data sets.
How would you rate the quality of the data used to run your business?
Only 8% of respondents reported having excellent data quality.
How would you rate your organization’s ability to get a single view of customer?
More than 30% of respondents lack ability to get a single view of the customer.
Challenges to ensuring data quality
Many sources of data (70%) and volume of data (48%) are among the top 3 challenges companies face when ensuring high quality data.
Applying governance processes to manage and measure data quality is second with 50%.
Consequences of poor data quality
Those who reported Fair or Poor data quality cited Wasted Time as the number one result (92%), followed by Ineffective Business Decisions (72%) and Customer Dissatisfaction (67%).
Confidence in data sent to analytics platforms
70% of respondents are “Somewhat confident” in the data their organization sends to analytics and data visualization applications.
Poor data quality leads to inaccurate data insights
47% of respondents had untrustworthy or inaccurate insights from analytics due to lack of quality.
Only 16% are confident they aren’t feeding bad data into AI and ML applications.
Leadership trust in data insights
Although confidence in data sent to analytics systems is lukewarm and almost half reported they’d had untrustworthy results from analytic platforms, nearly 70 percent of respondents still state that their leadership trusts the insights enough to inform business decisions.
Data quality is growing in priority
Although levels of confidence and trust in data appears mixed, 75% of respondents cite data quality as a high or growing priority.
Only 4% feel data quality is not a priority.
Data quality in the cloud
Leveraging cloud computing for strategic workloads
Have partial to no understanding of the data that exists in the cloud
Rate the quality of their data in the cloud as Fair of Poor
Data quality in big data
Have a data lake or enterprise data hub leveraging distributed computing platforms like Hadoop or Spark
Do not have a process for applying data quality to the data in the data lake or enterprise data hub
Rate the quality of their data in the data hub as Fair or Poor, while 32% rate their data as “Good”
Responsibility for data quality
51% reported that IT is responsible for data quality, while business users and data stewards play a critical role.