eBook
Data Governance 101
Read this eBook to learn about the challenges associated with data governance and how to operationalize solutions.
Mission impossible?
Overcoming data governance challenges
With today’s enterprises relying on big data analytics for business intelligence, implementing an effective data governance program is a top priority. Without data governance there are unanswered questions in understanding your data – “Am I using the right data?” “Is the data I’m using quality data?” After all, data is only valuable if you can translate it into actionable insights to inform strategic and operational decisions.
Creating a comprehensive data governance structure requires a process to deal with the most common problems around data. In fact, if a business can’t answer the following six questions, it’s a sign that they need a stronger data governance program.
6 Key questions to help identify the strength of your data governance program
- What does the data mean?
- Can I trust it?
- How do I find it?
- Where does the data come from?
- Is it the same thing to everyone?
- Who do I ask?
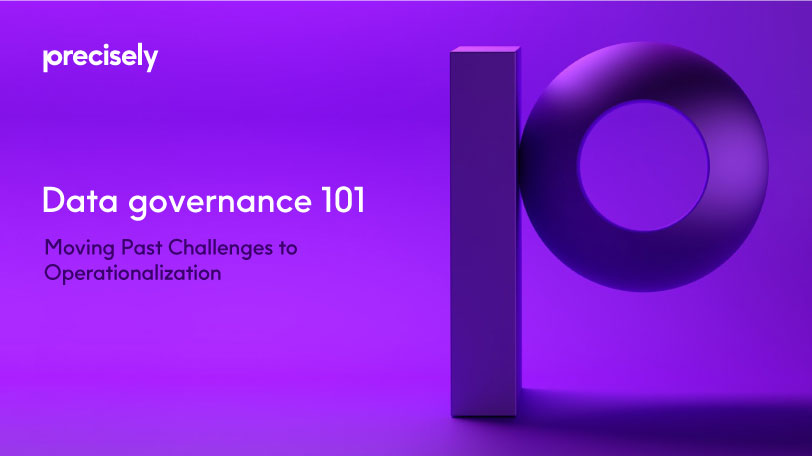
Understanding your data
One option is to put pressure on IT to do more. But with IT already stressed, overworked and lacking sufficient bandwidth, getting them to reprioritize often means sacrificing other high priority projects. Often, requests for data analytics take a backseat because IT is overwhelmed satisfying commitments for others across the organization.
Keep it secure
Ensuring the security of sensitive and personally identifiable information (PII) is a top priority for an effective data governance program. Having a place to view the data end-to-end is even more important. Many enterprises struggle to reduce data security risks due to unauthorized access or misuse of data, while others have difficulty managing the confidentiality, integrity and availability of data. By understanding the nature of the data, where it’s stored and how it’s used, enterprises can implement the appropriate governance guidelines for data use, and specify the right standards and policies around data ownership.
All roads lead to data quality
To keep data usable and reliable, users must trust their data. Most enterprises spend too much time gathering, normalizing, analyzing and reporting on data from multiple sources instead of understanding data provenance to make meaningful improvements. As data flows through the enterprise, the data must be accurate and timely, and must contain the right definitions and meaning. If you can’t pair the right definitions with accurate data, the data may be meaningless and insufficient. To derive business insights and analytics, enterprises must have accurate standardized data across all systems and processes to make solid business decisions
The new world of data privacy
Data protection regulations like GDPR and CCPA are changing, and complying with these new laws requires a strategic process. These new laws alone are driving many organizations to institute data governance since it’s imperative for enterprises to have the necessary policies in place to protect sensitive information, as well as ensure third-party data security. To enable data privacy, enterprises must go beyond third-party pre-and post-risk assessments and implement a data governance framework to provide visibility into these policies and how sensitive data and third-party data can be used.
Policy makes perfect
Many organizations have come to understand the critical nature of a governance framework. No matter where you are in the implementation process, having visibility into your data is a priority. Many organizations maintain legacy systems as new systems are implemented. Unfortunately, many times those organizations falsely assume their new platform will work seamlessly with their old system. Others experience siloed systems that cannot cross-communicate across the enterprise. For others, they just don’t have the right business rules in place. In each instance, organizations severely lack any visibility into their data. Whether it is examining current workflows, developing data definitions or the identification and documentation of appropriate business rules, developing the right business processes is critical to the success of a data governance program.
Data is complicated and multi-dimensional. If the goal of data governance is to make accessing data more efficient, by understanding the definition of the data, are there third party licensing constraints, where it is stored, who is the data owner and what policies dictate what can be done with the data, now is the time to determine if you have the right solution in place.
Data governance is an art, not a science
Data governance is not a standardized solution, but many pieces can be automated, such as extracting metadata, to accelerate both the deployment and on-going operations. With enterprises facing increased global competition, rising client expectations, tightening profit margins and increased regulatory demands, a cloud-based data governance solution can help organizations synthesize and visualize information about data in a manner which is easy to understand.
The right solution can also automate and aggregate data quality metrics to measure and analyze the accuracy, consistency and reliability of data at rest and in motion. This enables businesses to not only report on data lineage and governance metrics, but to continuously improve them.
The end-result provides a holistic view of data from both a business and technical perspective while also ensuring the appropriate data access controls are in place, making organizations better equipped to govern their data and take control of their business processes while also reducing costs.
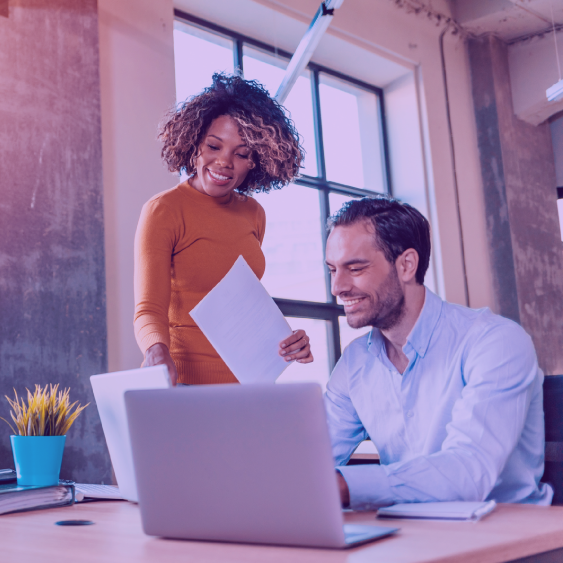
Can governance lead to data transparency and understanding?
33% of C-level execs don’t trust their data.
Can data governance help?
Data is a critical part of any organization’s DNA, moving from countless sources and flows through multiple systems in support of numerous mission critical business processes. Yet it’s rare to find anyone who will identify themselves as data owners, let alone take on that responsibility for data within their business line. The fact is, most people don’t know where the data that they rely on to make decisions originates, nor the level of trust they can put behind it. This is one of the main challenges and reasons why they don’t take ownership. This level of ambiguity makes it increasingly difficult for data users to understand how much they can trust and who to go to when they have questions. If you can’t trust the quality of your data and have no objective metrics to back up any quality claims, then you also can’t trust the insights you’re trying to gain by using it.
All it takes to discredit an analytical insight is for someone to present an issue with the ground breaking insight. When this happens, the overall morale is lowered and the culture of innovation is inhibited. Good data leads to better insights and increases the entrepreneurial spirit. This phenomenon should answer any questions on the ROI for data governance.
Why it’s critical to understand the quality of your data
Business users need transparency into the definitions and quality of their data to assess its fitness for purpose for solving business problems. Without transparency, data users are left in the dark and will likely make incorrect assumptions about what and how data should be used. For data consumers, understanding the level of your data quality is a big deal!
The reality is that measuring and communicating meaningful conditions around data quality is no simple task unless you have the right approach. Determining whether data quality is “good or bad” is not a binary condition – it depends on the expectations of each business process that is consuming the data.
Let’s take a look at an example:
Let’s assume you work at a bank and part of your job is creating monthly bank statements. It would be critical to have the correct address for each individual statement, including the correct name, street, street number, zip code, etc. To ensure having the correct addresses, business rules around address information will be defined and implemented. Because we are sending these statements to a bank client’s address, the threshold for errors would be extremely low.
But what if we were doing some repurposing of the data, something that had nothing to do with publication of bank statements? What if we were reporting on interests for certain types of products within certain areas or regions? In this scenario, the address information accuracy would have a lot more tolerance where only accurate zip codes were required. Inaccurate address information, bar the zip code, would not affect the outcome of this initiative.
We have established that the thresholds for good or bad data, regarding data quality, are completely dependent on the expectations of the business function using the data. This isn’t typically how we would think of data quality, and it raises the bar with regards to the types of measurement, articulation, dashboarding and communication capabilities required to achieve the result of an empowered data consumer across the various business functions. So how do we dive deeper into the quality needs for organizational data and figure out the thresholds of quality needed?
Measuring data quality
To provide a comprehensive measurement for the quality of data, an organization needs to have capabilities in place around data governance, quality and analytics. As information becomes available, these capabilities help detect, measure and report quality issues as well as provide an easy-to-reference business glossary that can model the business concepts and thresholds which give data quality context, impact and business meaning. To achieve tangible metrics we need the ability to calculate data quality scores. Once scores are defined and measured they can be leveraged to notify data consumers and data owners when thresholds are breached. Once notified, data consumers can use the glossary to get rich definitional information, ensuring a proper understanding of the data including the data’s lineage. Full visibility allows your team to gain valuable insights into not only the details of your data assets, but also with the associated risks with its use across various business applications.
Having a fit for purpose practice and solution around data provides users with the ability to choose the right data at the right time, gain a full understanding of the data sets, its definition, ownership and associated quality, knowing that their quality levels may differ from other consumers of the same data. This inventory of knowledge can provide straightforward answers to fundamental questions being asked by all data users, such as:
- Who owns the data?
- Can you trust the data for our particular business function?
- What’s the definition of the data?
- Are the definitions the same across all systems?
By providing data governance, quality and analytical capabilities, organizations can gain a broad and comprehensive understanding of the data, enabling all data consumers to extract maximum value, knowing the quality levels and minimum expected quality level needed to meet their own particular business needs.
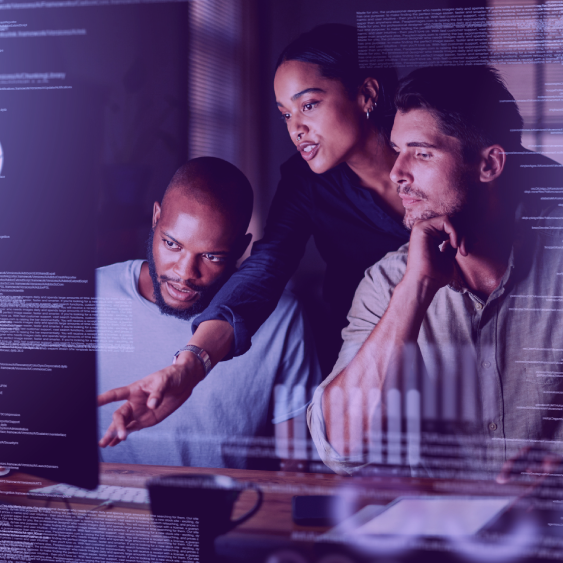
Metadata management 101: Managing data about data
Learn the ways to organize data and ensure proper metadata management
Lingering data usage problems
We constantly hear about the explosion of big data and how important data is to any business across any vertical. However, so many business users simply aren’t using their data because they don’t know what they have (do you have an up-to-the-minute inventory of your enterprise data?), they can’t find it (does any individual in your organization know where all of your important data resides?) or they just don’t trust it (we found it, but where did it come from and what does it mean?). If you can’t answer all of these questions definitively, then, surprise, you’re not alone!
In large organizations, data inevitably spans many systems and thus, IT has the challenging task of integrating data from various processes and systems. For example, many organizations maintain traditional and legacy systems and are expanding their data capabilities with cloud storage, big data Hadoop clusters and third-party vendor data. Each of these data repositories has its own rules and requirements. The modern organization’s Data Supply Chain is massive, complex and scattered and all it takes is one change, such as a 3rd party feed switch, to impact an array of business processes. It’s no wonder organizations struggle to pinpoint the right data when they need it. Yet, these same organizations require real-time insights at the speed of business to make informed business decisions to achieve or sustain a competitive advantage.
Metadata management 101
Organizations are now looking toward metadata to help solve this problem. In short, metadata is simply data about data. It can tell us various data attributes including where data resides and how to find it. Remember the days of finding a book by identifying information (aka metadata) such as an author, title, subject or date of publication? The card catalog is the first place you would go to search for something in the library. It would tell us where to find the book and how the library was organized. If a card catalog tells us how books are organized, we can think of a centralized metadata portal as a card catalog for data. Just as books reside across a library, on different floors, in different sections, etc., data is scattered across disparate systems in different formats in an enterprise. Metadata is (or at least should be) stored in a central location and used to help organizations standardize how data is located. However, before you can organize the metadata by type and understand how it functions, you need to go back and understand where metadata starts, and define your data.
Organizing and defining data
Before metadata can be used to create a glossary that tells organizations exactly where the data is located and how it should be used, we must understand the purpose and value of metadata to the business. To fully understand data, it must be triangulated—viewed from three different perspectives. Gathering metadata from these different perspectives is the only way to achieve a comprehensive understanding of how and where data lives in the business:
Physical data perspective
Organizations have multiple databases and each one has a code specifying where exactly each set of data lives. The metadata in this model should include information about where each system resides and where certain data sets are located within each system. Typically, this type of metadata can be automatically derived from the software that runs the physical hardware.
Logical data perspective
This category should contain metadata about how data travels from point A to point B. Essentially it is a map that tells organizations the data’s origins, what happens to it and where it has moved over time. The logical data model shows how data should flow through an organization and gives us a picture of where the metadata comes from and how it is transformed.
Conceptual data perspective
Conceptual metadata should convey the meaning and purpose of a data set from a business standpoint. It should tell users what the data means, for what purpose/s is it typically used, when it was created, if it is up-to-date and if it is confidential or not, etc. The conceptual data model requires human input to define the data. It also requires users to continuously update this metadata because it changes over time. For example, a data scientist might find a new use for ‘old’ data. In this situation, the use case metadata should be updated to reflect this new purpose for data. To effectively manage this much metadata, users must be able to go in and suggest updates with a process in place for certification or approval. Think Wikipedia, anyone can go in and add their two cents, but there are also controls for editing to ensure that sensitive or controversial topics are not corrupted with bad information. An effective metadata implementation will have similar controlled crowdsourcing functionality.
Once an organization views their data from these perspectives, the metadata model will start to take shape. The next step is to implement an appropriate data governance solution to organize their metadata and place it in a centralized repository.
Data governance solution
To create a comprehensive data glossary that offers business professionals transparency into the ownership dimension, organizations need to invest in a data governance solution. The solution should promote fluid communications between the data owners and the data consumers. In addition, it should have extensive collaboration capabilities for users to gain expertise on their data.
The solution should deliver an all inclusive view of an organization’s data landscape. By delivering transparency into all aspects of an organization’s data assets, business users can gain valuable insights into not only the details of their data assets, but also quality of their data and the attendant risks associated with its use across business applications. Like with any corporate asset, you need a current inventory and value assessment to even begin the process of tracking and securing data. Comprehensive, current metadata is essential for data protection and security.
Finally, the right solution should have automatic discovery capabilities, enabling the capture and monitoring of changes to metadata. Once changes are discovered, the technical metadata relationships are the raw material upon which a business lineage is built in order to deliver meaningful insights on data in a business context. With the proper data governance strategy, enterprises can successfully create a comprehensive data governance glossary of business term definitions that appear in data artifacts such as reports and applications. At the heart of data governance is more than just standardized terminology, but people that oversee the data and answer questions. This is accomplished by assigning data owners and data stewards that are responsible for organizing and maintaining data definitions, usage rights and data quality parameters so that business professionals can consume data in a business context.
Understanding data lineage from varying perspectives
Understanding data lineage from a business and IT perspective
When looking at data lineage, a single quote from Star Wars sums up a lot of the confusion and expectations, and our understanding and expectations of the function. Ask a technical user for lineage and one would expect to receive a complex diagram that represents flows through every single store, extraction and transformation point throughout the enterprise. Ask an enterprise architect about lineage and definition and expectations will differ significantly. Each visual will represent the “truths” according to their points of view, but may not represent the views of others whose views represent the business use and governance of the data.
“…many of the truths we cling to depend greatly on our own point of view”
Obi-Wan-Kenobi
Star Wars: Episode VI – Return of the Jedi
Lineage is pervasive
In our everyday lives, we tend to take GPS trackers for granted – but they accomplish a lot, tracking our routes from source to destination, collecting all types of statistics and optimization to storing historical data. In the workplace, the vehicle for us to make many of the critical decisions we make is data, and the routes are built using myriad of underlying technology components. Using the GPS analogy, as business users we are interested in getting our data at the target destination without worrying which satellite carries the signal, the additional maps, alternative routes or historical statistics needed at a granular level to deliver the data, but rather the high level view of how we get our data delivered in a trusted and timely fashion. Without this concept of a business oriented solution to navigate for data we use, we are left with a series of confusing artifacts (though important to the technical population), which attempts to provide some direction such as technical data lineage sourced from an ETL tool to track data flows as it moves from system to system.
What’s missing is business lineage
There are different perspectives on how to view your data – from a technical standpoint (where does it live and how does it move) and from a business standpoint (what do you need to know to make a good decision). While solutions such as Master Data Management (MDM) provide a perspective on data lineage based on a technical point-of-view, business users are aghast at trying to interpret these illustrations of technical data flows. If the business is to drive data governance and accountability, they need to understand data flows from a perspective of how the data is used to perform a business function. This is a very different perspective and requires the technical details to be synthesized in a language which captures the business impact.
As data flows through an organization, it goes through multiple systems and consumption points that can transform and alter the data. Because many systems act as source and target, understanding the data flow can help us have a better understanding of how to ensure data quality.
At the end of the day, the organization’s data impacts business decisions, how you manage your operations, mitigate risk, forecast profitability and much more. If you don’t understand the impact of the data on these items you won’t understand what to do when the data changes or new priorities emerge. Business data lineage should answer all of the questions that business producers, contributors and consumers need to solve a problem, finding the accountable party, or discovering new insights.
To understand the data lineage requirements, we should first understand the different personas of your users.
Types of users
In the business world, there are multiple departments with various employees all of whom have different goals. So, it is important to understand what each user is trying to learn from their data lineage.
Data custodians
Data custodians are usually technical organizational resources, and typically are interested in the physical storage and movement of their data. They understand the more granular enterprise data flows and how to navigate the various “hops” that data makes. Their interest in lineage is the multiple steps data takes through environments, persistent stores and any other technical variations.
Data support
The second type of user is Decision Support. These users are typically business analysts who may understand some, but not all, of the technical information. They’re interested in the business perspective on application and vendor data, data mapping and data transformations.
Decision makers
High-level users are the Decision Makers, who understand the high-level flow of the information and the business rules and policies that impact lineage. They’re looking for information about data lineage through business processes, like business functions, business rules and business policies.
The two views of data lineage
In addition, there are also two different ways to view data lineage depending on the user and what they want to accomplish.
Directional view
This view explores data’s origins, where it moves over time and describes what happens to it through diverse processes. This type of view helps provide visibility into the data analytics pipeline and simplifies error tracking, and is typically used by high-level or intermediate users.
Impact view
Rather than look at directional details of lineage, this view looks at relationships. This allows people to interactively explore data relationships, query the entire glossary from a visual perspective, as well as show the impact security policies have on different data domains. It’s usually used by the low-level user.
Solution
Organizations can implement an automated data governance tool with interactive data visualization and lineage capabilities. The solution should deliver both a directional and impactful view, and include extended search capabilities covering data relationships and hierarchies.
The solution should also allow for low-level, intermediary and high-level users to gain valuable insights into data flows, definitions and responsibilities. It must also be interactive and should skew more to the business population while still providing technical oversight for critical data elements. The ability to automate where possible to reduce the manual footprint is also valuable for the extraction of business lineage, utilizing connectors where possible to extract technical metadata and lineage from various systems and applications.
The importance of data lineage
So far we’ve identified who benefits from data lineage, as well as the various perspectives for different audiences. But why do we need it? Here are just a few reasons:
- Data lineage helps explain the different processes involved in the data flow and their dependencies, which allows the various user groups to better understand the data they consume and make critical decisions that may impact the organization.
- Data lineage helps with the maturity of a data governance program because it provides the needed information, at the right level of granularity and context for business to understand and direct the program.
As noted earlier, data continues to grow exponentially both in volume and scope, making knowledge about data even more critical. Understanding your data’s quality, correctness and completeness using data lineage helps all audiences better understand their data and make more informed decisions.
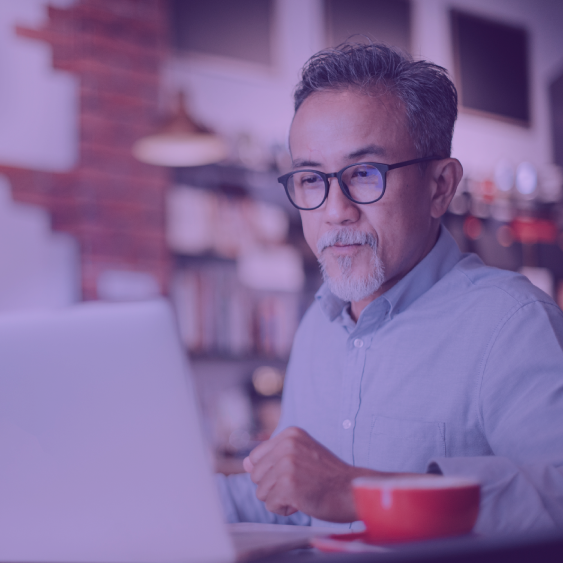
Creating a data governance business glossary: A practitioner’s point of view
How a business glossary clarifies data meaning
During my time as a product manager, Chief Data Officer and Data Strategist, I’ve come across many quick fix solutions to tackle the business glossary challenge including, “we have an Excel-based solution.” In reviewing the solution with the client, more often than not, it does effectively inventory and define terms, but it soon becomes apparent that this is an overly simplistic view of data beyond a simple cataloging of terms with limited ability to capture the important relationships and gain valuable insights into this important enterprise asset.
Taking a business user’s perspective when looking for the right information to consume, the search for data typically starts by looking for the likely candidates by performing some form of key word searches – easy enough. Next, they will want to answer questions related to the quality level of the data, find out how to receive the data and gain an understanding of how and where they may be used. They may want to initiate a conversation with the data owner or leverage a mind share of knowledge through collaboration with other peers. Data consumers have the right to be able to answer these questions quickly, but these types of inquiries will not be sufficiently addressed with the simplified and flattened view of data being provided by Excel or SharePoint-based solutions.
Data alone does not prove value, it is its movement and interrelationships with people, processes, technology and other data assets that generates value.
The value of data
Knowing these relationships, ensuring the data is properly managed and kept current are many of the capabilities of an enterprise business glossary. Users should be able to get answers to the following questions about data and its inter dependencies:
- Where is the data consumed within the organization?
- Is the definition current, certified and available or are we reviewing an older definition of the term?
- Are there synonyms, abbreviations or phrases used to describe the same term?
- Does everyone use the same terms consistently?
- What issues are outstanding for this business term right now?
- What are the impacts of changing the source, formats or delivery of terms?
In order to gain comprehensive insights into the deep understanding of data, we need visibility into a number of dimensions of the data such as consumption, technical and business representation, associated policies and rules, as well as the roles and responsibilities associated with the various data assets. An enterprise solution provides users with these capabilities and with the ability to appropriately structure and synthesize the dimensions that surround your data.
Shared data – Its value and risks
There are many cases in an organization when new projects rely on data where it is shared across departments and even business units. When business lines are dependent on each other’s data, a robust business glossary becomes critical as each business unit has its own priorities, dialect and functional use of information that may be the same or differ in definition and rights from other consumers. It therefore becomes an imperative to leverage a robust solution that shares knowledge to meet everyone’s objectives. Think of the business glossary as being the card catalog in a library. Books can cross many different classifications such as thriller, autobiography, history or geography. They can have specific attributes that can help you search for the book such as genre, publisher, format, author and publication date. In much the same way, a business glossary can use various classifications and content about data to aid in the search, availability and usage of the enterprise data. Having this searchable catalog at an enterprise level provides a level of transparency around helping to avoid ambiguity around the data in use.
The business glossary
A business glossary defines not only the data vocabulary across an entire enterprise, but ensures consistency of business terms. It synthesizes all the details about an enterprise’s data assets across a multitude of data dictionaries and organizes it into a simple, easy to understand format. Glossaries bridge the business and technical divide by providing transparency into definitions, synonyms and important business attributes while tying these important attributes to the more technical definitions stored within the various critical system, reports or processes. It also identifies the owners of data and subject matter experts while enabling collaboration between different departments.
Let’s consider a simple example where the marketing department has a field in their database that simply says “Name.” That could be a first name, a full name, or a last name. It’s not clear. A business glossary identifies this discrepancy and creates a field that is more clearly labeled with the right business context information. It then becomes clear to all users that Name means full name, with first name first and then last name.
A glossary can also provide lineage so the enterprise can understand the flow and dependencies of the data. It can also identify critical business process relationships, provide transparency into the various data quality dimensions and communicate data access methods and usage restrictions to data consumers. Having common data definitions and transparency, users can easily communicate and ensure they are using the right data for the right purpose.
Delivering a comprehensive understanding of an enterprise’s data, the business glossary can enable data owners, data stewards and data consumers to effectively manage and apply data to extract maximum business value within their functional areas while being cognizant of the other consumers of the shared data assets.
Creating a business glossary
Business glossaries don’t just exist; they must be created over a period of months or even years. Depending on the size of the organization, business glossaries can be complicated because data is complicated. Different people in different departments have different perspectives on data. Getting cross departmental agreement on standard definitions based on individual perspectives of data is a strenuous task. Unless, of course it’s automated.
In order to create a comprehensive business glossary, enterprises should implement a data governance solution that connects the dots of data lineage, data definitions, data quality, with the business glossaries. The solution should provide the ability to not only measure the outcome of data quality rules, but also articulate the impact of the data quality by the expectations of the business. The suite should offer data consumers transparency into the ownership dimension, and ensure fluid communications between the data owners and the data consumers. The solution should have extensive collaboration capabilities in order for users to gain expertise on their data.
Finally, the right solution should have automatic discovery capabilities, enabling the capture and monitoring of changes to metadata. Once changes are discovered, the technical metadata relationships may be investigated to deliver meaningful insights on data. With the proper data governance suite, enterprises can successfully create a comprehensive business glossary that is user friendly, flexible and most importantly maintainable.
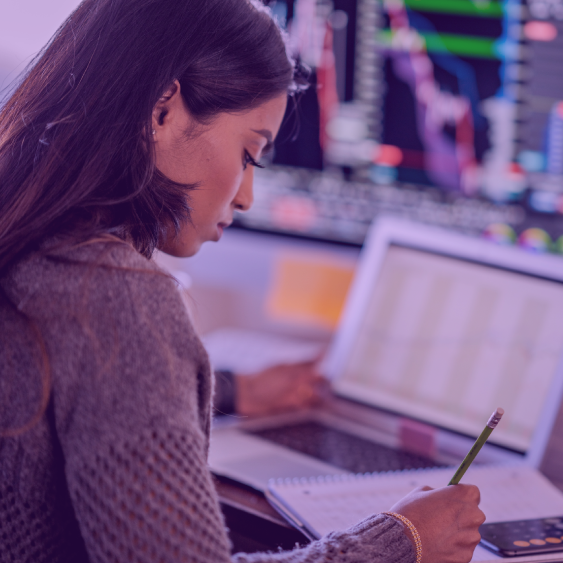
Wrapping analytics around data governance
Learn how automating analytics around data governance will give you insight never seen before
Data is a competitive advantage for any organization that knows how to properly extract its insights. But to be successful, organizations must govern both internal and external data to improve its quality, use, trust and more. When done properly, governance also bridges the technical-to-business divide by engaging all parties to combat the increasingly complex demands around regulations and compliance. Data governance gives organizations the transparency into all aspects of their data assets, from the data available, its owner/steward, lineage and usage, to its associated definitions, synonyms and business attributes.
Full visibility into an organization’s data allows all data users to gain valuable insights into not only the details of their data assets, but the attendant risks associated with its use across business applications. Many organizations across multiple industries are now looking to implement a data governance framework, however, many organizations do not understand the importance of analytics for its success.
Why analytics are important to data governance
The reason analytics are so important to data governance can be summed up in one word, automation. Analytics can help automate some important tasks that would normally take large teams of people to accomplish. Analytics can also provide additional insights into data that would otherwise go unnoticed. By applying techniques, such as machine learning to data sets, organizations can automatically detect anomalies based on historical patterns, rather than a person setting a rule to look for them.
This is increasingly important as complex demands around regulations and compliance continue to increase. Let’s use the General Data Protection Regulation (GDPR) as an example. The intent of GDPR is to strengthen and unify data protection for all individuals within the European Union (EU). The goal of GDPR is to give control back to citizens and residents over their personal data and to simplify the regulatory environment for international business by unifying the regulation within the EU.
To ensure GDPR compliance, organizations across the world will need to ensure governance around personal data documentation, identification, tracking and usage approval. A data governance framework with analytics incorporated into it can deliver enterprise-wide control and visibility into personal data processing risk areas, automatically identify where proper oversight may be lacking, and utilize machine learning to account for any hidden personal data, in order to comply with GDPR. But to achieve compliance requires the right solution. And to do so, using one solution instead of many is the preferential route for many organizations in an effort to increase efficiency and reduce cost.
A solution for wrapping analytics around data governance
A proper data governance solution should deliver an all inclusive view of an organization’s data landscape allowing organizations to easily define, track and manage all aspects of their data assets. This enables collaboration, knowledge-sharing and user empowerment through transparency across an enterprise.
With analytics wrapped around the solution, it should include a self-service, big data analytics platform designed to handle not one, but rather multiple steps from data ingestion and preparation to data analysis and operationalization. Where data quality checks are required, an integrated platform solution must be able to conduct data profiling, completeness, consistency, reconciliation/balancing, timeliness and value conformity in order to roll up data quality KPI’s alongside data definitions within the data governance business glossary. The solution should be designed with a data preparation visual workflow to empower the business user to aggregate and control data in order to accelerate and improve the subsequent data analysis process, applying analytics to extract value from the data. Users should find a solution that enables them to source data from multiple data platforms and applications. It should empower users to apply statistical and process controls, as well as machine learning algorithms for segmentation, classification, recommendation, regression and forecasting.
Users can create reports and dashboards to visualize the results and collaborate with other users. Additionally, it should allow users to create automated notifications, manage exception workflows and develop automated data-processing pipelines to integrate the results of that analysis back into operational applications and business processes.
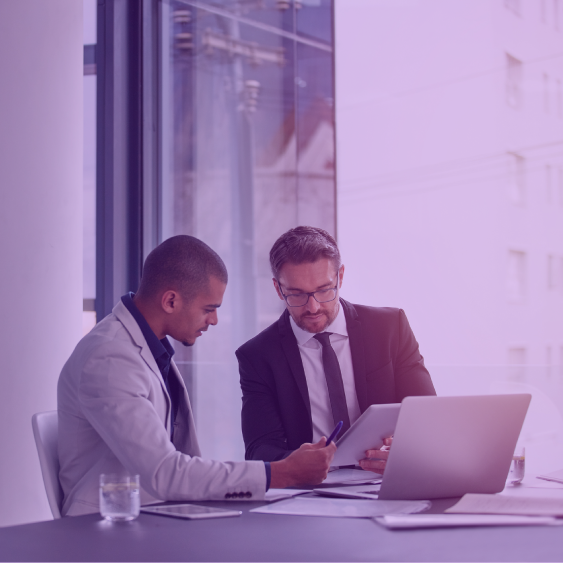
Why a data supply chain is required in the age of big data
Learn why a business glossary is pertinent to a Master Data Management system
In recent years there has been a growing awareness among organizations around their data and the role it plays in the success or failure of their most critical business functions. This shift in mindset along with the evolution of cloud technologies has formed the basis of change in technology budgets from a concentration on hardware and infrastructure purchases to one that leverages technology and services that make the best use of corporate data assets. In line with this has been the rise in popularity of Master Data Management systems (MDM). Used in the management of critical shared data domains such as security master, product master, or client master, MDM, when properly implemented, can form the cornerstone of an organization’s Enterprise Data Management (EDM) strategy.
MDM – not the silver bullet
The goal of MDM is to identify, validate and resolve data issues as close to source as possible, while creating a “Gold Copy” master dataset for downstream systems and services to consume. MDM provides many benefits and, when implemented correctly, can ensure consistency, completeness and accuracy of core shared data sets. But MDM is not the silver bullet of data quality for the enterprise. At its core, MDM manages just a single area of the data universe namely, business entities. If we look a little deeper into an organization’s data use, we find that many business and technology functions rely on a mixture of operational data, reference data, metadata and audit information in addition to the aforementioned master data, with the quality of each being of equal importance. MDM does a commendable job of ensuring the shared master data is managed correctly and is fit for purpose, however MDM does not represent a full data governance or EDM program.
Quality is only part of the data equation, whereas organizations need a broader view and transparency into the data they plan on using for critical decisions, and this is something that MDM systems are not well positioned to provide.
In addition to mastering data, the following capabilities need to be addressed:
Definition
What does the term mean? Are there any other names (synonyms), phrases or abbreviations that this term is known by. Is the term calculated or licensed?
Data classification and retention policies
Data may be classified many ways based on both internal and external policies. These can further drive usage rights, disclosure and disclaimers.
Original source
Where did the data come from? Are there multiple sources? Are there any sourcing/priority rules when creating the gold record? What is the authoritative source for a particular set of data? Can it be overwritten?
Search
What data is available and from where? Is it shared? Which business functions are using which set(s) of data?
Collaboration
How can people become more knowledgeable about and around organizational data? How can they contribute their expertise?
Enterprise data quality
How trustworthy are the various types of data in use? Is there a pattern or trend to the various domains of data?
Operating models
It’s fair to say that the data won’t manage itself, there needs to be policies, procedures and resources applied to ensure the operations and drive quality, security, access rights, sourcing and the proper use of data throughout the organization
Responsibilities
Probably the most contentious area and the one where most companies struggle is where the accountability lies. Assigning people to roles is one component, but what are the expectations? How do we establish models of interaction and measure effort of staff navigating through the cultural, political and personality land mines to ensure the optimal use of an organizations’ resources and their data?
MDM’s myopic focus makes it impossible to address these areas across the organization’s broader spectrum of data, and highlights the key differentiators and importance of data governance to the organization.
“MDM Without governance…is just data integration!”
Aaron Zornes
Proper governance sits on top of MDM, data movement or data warehouses for that matter, and ensures that the data is understood by the business from a definitional, sourcing, quality and accountability perspectives. When embarking on large scale data driven initiatives, especially ones that bring large cultural and operational changes, it’s imperative that data governance is established early and incorporated into every phase of the project. Data projects that neglect data governance run the risk of delivering a technical masterpiece, that is both impractical and too complex for the business to understand or utilize. Integrated Data Governance can also ensure the business backing and active participation in initiatives that are often times perceived by the business as a technology exercise owned and operated by IT.
This astonishing statistic from Gartner solidifies the fact that business participation in many MDM programs is lacking and that business fails to understand, embrace or value these multi-million dollar investments in MDM. Review any statistics on failed or underachieving MDM projects and all will most likely point to a lack of data governance incorporation to manage the people, processes and most importantly the data needed to succeed.
“The data governance, prioritization, people and process aspects of implementing an MDM solution will likely derail the project before the technology fails.”
Wang and Karel
The value of a business glossary
The establishment of a data governance framework, operating and reporting models are a great first step for organizations to manage their data. In much the same manner as organizations inventory their other corporate assets with HR and finance systems, the data assets need to be properly defined, inventoried, managed and ultimately opened to collaboration. Organizations typically start this process with internal solutions leveraging spreadsheets, SharePoint or some other homegrown solution. The challenge with these solutions arise as more and various types of data assets need to be populated, as well as the ability to track lineage, workflow, impact analysis or collaboration capabilities for the various data governance roles. In the end, the glossary becomes the glue that ties the data governance capability into the MDM project, ensuring business participation, accepted business term definition and assigned and documented accountabilities for the governance of the mastered domains.
Knowing up front MDM’s capabilities and especially its limitations can help an organization to incorporate solutions that provide a full 360° view, understanding and transparency into their corporate data assets.