eBook
Customer 360 Uplifts Customer Experiences and Fortifies Competitive Advantage in Retail
Read this eBook to explore how building insights from relationships between people, places and things require trusted data in order to build a Customer 360 in retail view.
Customer 360 in Retail – Data and the Digital Economy
It’s a hard fact. More data is available today than ever before – but most organizations can’t capitalize on it to improve their experience with customers. There are several reasons for this, but the most common ones are:
Integrating data technology is a major challenge. When critical information isn’t connected and available to decision makers, you miss important strategic opportunities.
An organization that can’t control its data simply can’t control its business.
Change is a constant. It’s not easy to hit a moving target. Place names, street names and people are constantly changing. People get married, divorced, move and change names.
The challenges don’t stop there. With the explosion of social media, people interact with your business across more channels and locations, and some even connect through more than one channel at the same time. Without the right tools, you’ll never gain insights based on the most accurate, precise and up to date data.
Yet, despite the challenges, organizations need a full Customer 360 view to both grow their business and protect it.
-
- Digital transformation and improving customer experience. Digital transformation is a key focus for many organizations. Across all verticals, businesses invest in these solutions to improve their omnichannel experience, drive personalization both in-store and online – through various touch points and optimizing their data in their CRM (Customer Relationship Management) systems. There is typically a need to go further than the single “collect” stage as this data may not necessarily be as actionable or insightful as today’s companies require.
- Data Governance/Risk Management. Many organizations today have very specific requirements to support their various initiatives around: FCC/AML, Data Privacy (GDPR, CCPA, Australian Privacy Act) or self-service BI (Business Intelligence) and analytics.
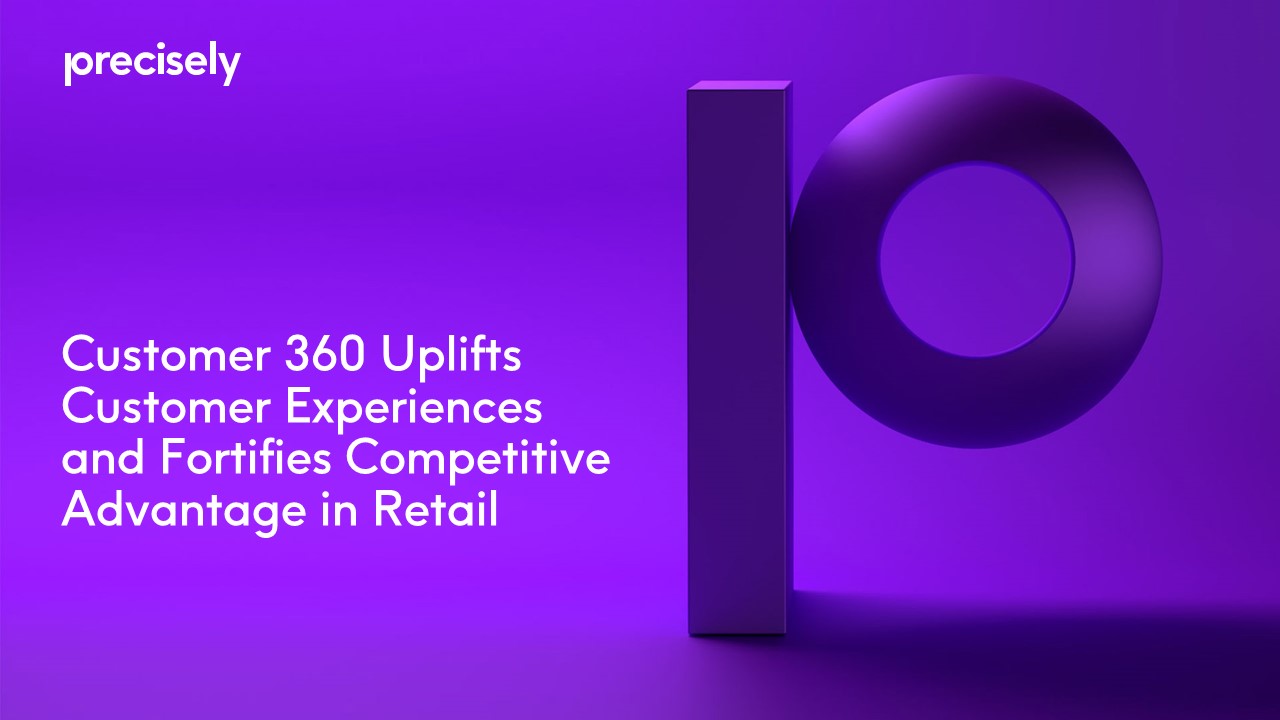
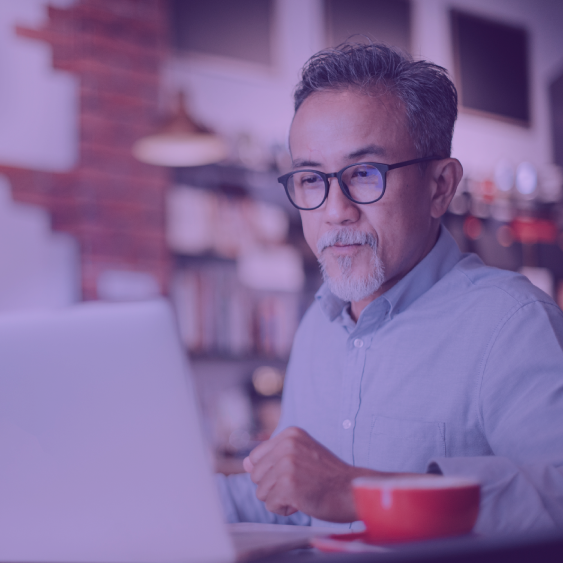
In this ebook, we will explore the power of going beyond the Customer 360 basics (name, address, account numbers, transactions) to greatly expand the knowledge of your customer. What they buy. How they shop. Where they spend their time. What they value. What their next actions may be…
While traditional master data management projects can provide some of this insight, they can take years to implement. By the time they’re ready, business needs have changed. Fortunately, there’s a better way.
Discover how to achieve a fully integrated customer view with accurate, enriched and accessible data – when and where you need it.
Customer 360 needs across industries
Retail
Create powerful customer experiences
- Inform real-time, mobile interactions
- Enable best-next engagement
- Place stores in the most profitable locations
- Optimize omnichannel sales
- Reduce risk and combat fraud
Insurance
Profit from a precise perspective
- Price, assess, manage and mitigate risk
- Detect fraud in near real-time
- Create personalized customer experiences
- Acquire new customers and retain existing customers
- Make all customers more profitable
Public sector
Connect with your community
- Allocate and manage government assets and services
- Analyze crime by day, time, location and type
- Improve agency efficiency through better citizen service
- Take steps to reduce waste, fraud and abuse
- Reduce improper payments and investigation costs
Financial services
Increase your share of wallet
- Comply with anti-money laundering and KYC
- Enhance entity resolution to combat financial crime
- Create personalized customer experiences
- Optimize branch, ATM and online networks
- Reduce call center volume
Telecommunications
Capitalize on your coverage
- Optimize wireless confirmed coverage mapping
- Access network assets versus subscriber locations
- Plug into big data opportunities
- Optimize cross-sell and upsell activities
- Increase customer loyalty
Healthcare
Enhance connections and compliance
- Reduce onboarding time and costs
- Improve member/ patient satisfaction
- Enable collaboration across payers and providers
- Identify gaps in care networks
- Create comprehensive, compliant customer records
The shared and evolving challenges
What is it specifically about data that creates a challenge to execute these strategic initiatives across your organization and within your industry?
- Typically, different silos of information and pockets of knowledge exist across all your different systems within your enterprise.
- These silos limit your ability to drive the strategic priorities and key use cases we mentioned above, to move your organization forward.
- It’s very hard to derive the insights and the analytical view that are needed without getting a handle on your data.
- The traditional focus within master data management has been around getting that single view of the individual.
- Deriving the best name address for one record requires key functional capabilities like data integration, data quality, matching and linking.
The shift we have seen for the last 10 years is to not focus on mastering a single entity in isolation, but rather on understanding how that customer or product – whatever the entity might be – relates to other aspects of your business.
The need is around building clear models that the business can leverage strategically, and then understanding which relationships exist within the data available to your enterprise. Whether they are your customers’ relationships to the products/services you provide, or whether it’s their relationships to one another through households or organizations they may belong to.
Relationships to external data- through social media or specific locations are also key. These are all important to establish and go well beyond what traditional solutions have been able to deliver to organizations- enabling them to model, understand and optimize the interactions they have with their customers (transactions or communications via social media, call center, website, point of sales, mobile devices).
Brands can model all this information whether from anonymous or personalized touchpoints.
This will enable the true understanding: that true 360 degree view, releasing customer centric insights to really move your business forward strategically around key strategic priorities.
Strategic initiatives like digital transformation/customer experience really requires that we get a handle on your very complex data environment and data ecosystem across your enterprise. Working with key clients, we have seen patterns emerge.
The customer 360 journey
It all starts with the right questions.
When we look at managing information across the enterprise data ecosystem, we begin by simply trying to get an understanding of the questions we need to answer.
- How does the business need to see the data that exists across the enterprise?
- What are the key kind of entities that we need to understand?
- What are the relationships between the entities that we need to understand?
- How can we model these? Simply, on a whiteboard, in a semantically focused way – the business can work with the IT organization to gain deeper understanding – for example mapping customers, products, organizations, web interactions and calls – understanding required views and relationships.
Building those models is really the key first step to kind of bringing that business & IT conversation together.
How do we understand quality data to support our models exists within (or possibly outside) our organization?
Most organizations have hundreds of data sources that may contain some sort of customer information and that list is expanding. This would also apply when you look at mastering other entities, like product.
Discovering them based on the semantic models that you’ve built, tagging those data resources, cataloging them in a way that allows you to redeploy or reintegrate them for other use cases per the requirements of your next strategic priorities helps to lay a foundation for ongoing improvements.
Profiling is needed to understand the general quality of the data and it’s fitness for purpose. How do addresses and location information help us understand context?
Profiling your customer data is your first view around what context exists and whether these relationships that you need to model are easily formed or if more upstream work is needed (cleansing, mastering, deduplication, defining key data quality rules, standardization/ validation, linkage/consolidation, creating a golden record).
Enterprise challenge:
Pockets of knowledge across systems of record, insight and interactions
- Organizations can enrich again with location intelligence or geo-spatial information to enable additional context
- Persisting the information into a central repository or perhaps federating certain aspects of it from external systems that we may not want to master frequently across the enterprise.
- Operationalizing these models is the key to driving strategic priorities forward and moving towards more real time environments. For true data democratization, we need to enable user experiences understand to find patterns, visualizing the information and the relationships that we’ve developed through our model. We strive to develop a master record or a centralized view of your data – enabling the ability to search, govern, edit, and maintain it
Through real time interfaces, we aim to integrate these models into other applications to drive true value across the enterprise. Agility is also a key aspect of successful projects. It’s not enough to spend a year or two building a master data management repository and centralizing data sources, if you don’t take the final step of operationalization.
Many client organizations are driving key analytical processes and systems of insight based on the information consolidated within the single view.
Agility is key. It’s not enough to spend a year or two building a master data management repository if you don’t take the final step of operationalization.
To avoid going down the path of a “waterfallish” type project and not realizing that value- it’s critical to incorporate the agility and the iteration in these processes to really bring that value to bear on the business.
Traditional MDM has been very focused on developing that golden record for the name and address in the case of the customer domain.
When we look at single view or Customer 360, it’s much more dynamic, agile through this federated or virtualized view.
Mastering still has to happen to a certain level, but how do we provide those deeper insights?
How do we drive those predictive analytics by linking up to other systems in more of a dynamic, federated manner, including those traditional processes, while still providing connections, understanding relationships, plus other data and attributes to really drive that contextual view?
The focus is on context. This is what drives the deeper understanding of the relationships, as opposed to just focusing on mastering a single entity in isolation.
This is a lot of what we’ve seen evolving over the years and a lot of what we’ve incorporated into our product innovations – including metadata and graph.
The innovation journey
Cloud is a key part of the vision, deploying solutions to the cloud, building a cloud native architecture.
Let’s look at the different approaches to this in the industry.
We focus on the best value solutions for our customers: applying machine learning to specific problems that exist within data management, information management and Customer 360.
Resolving entities, building match rules, semantic data discovery – these are all processes that can greatly benefit from adding machine learning into that sort of data steward loop of iterating over these processes.
A span of personas
There are a number of different types of personas for these types of projects: from traditional IT roles like data engineer or data scientist to a business level like C-Suite, marketing/business analyst; from data producers to data consumers.
Leaders need to see key reports with their own views into the data and really drive their own decision making based on that.
All of these personas are key to ultimately delivering full value to the enterprise.
A hybrid role
In looking at the different personas and roles involved in Data Management, we see more and more individuals across the enterprise that span both business and IT sides of the house. This has triggered a need for business user focused interface to drive access to data and analytical information.
The information consumers
Chief
Marketing Officer
- How do I improve the quality of leads and demand generation process?
- Am I over-messaging the same people without knowing it?
- Who are my top customers and how where do I find more of them?
Chief
Customer Officer
- How can we deploy a solution for providing consistent customer experience across all of our channels?
- How can I identify the major phases or our customer journey and determine what information is needed with each phase?
Chief
Information Officer
- What foundational technologies are needed to shift the business to a digital-savvy org?
- How do I enable a seamless flow of information between the front office and back-office systems?
- How do I enable my organization to be customer-centric?
Chief
Compliance Officer
- How do I recognize potentially fraudulent business transactions?
- How do I stay compliant with regulations without ballooning the cost of compliance?
The information workers
BI or CRM
IT Director
- Is the name and address data on customer records correct?
- How can I implement data quality and accuracy directly into the CRM system?
- How do I harness more social data in my CRM?
- How do I enhance my existing BI reports with information from new sources like Hadoop, Mongo, Amazon S3?
Data Steward, Data
Governance Analyst (IT)
- How can I ensure consistency and accuracy of data as it flows from application to application?
- Is there a way to quickly review and manage all changes to business data?
- How can I identify issues with sources systems (such as calculation routines and missing values)
Enterprise Architect
Data Architect
- How can I re-use enterprise data across more applications and systems?
- Is there an easier way to communicate overall data quality status?
- How can I support data sharing and master data management objectives (such as official product hierarchies valuation models, customer segmentation profiles and preferred suppliers)?
CDO/CAO
- How can I find ways to leverage existing data?
- Is there a way I can use the information to identify new sources of revenue?
- Is there a way to augment missing information with internal and external sources?
- How can I manage the health of data that I’m governing to stay compliant?
Evolving approaches to data management
Typically, if we look at traditional solutions as it relates to managing data, you have a few options:
Traditional approach (relational databases)
- Most popular is a relational database, which represents data in a tabular format. Links between data elements are typically handled through join tables.
- RDBMS struggle at times to really manage or understand highly connected data – where the relationships are key to drive true understanding and insight. Join tables perform great for the most part, but certainly anybody who’s ever written a joint statement and SQL understands that they can get very complex very quickly. Their performance can break down under scale.
- Graph database has an advantage in its ability to effectively break those relationships directly into the underlying data structures that exist on disk.
- Graph visualization techniques allow us to represent data more intuitively than tabular format reports for user personas that are much more visually focused.
Next generation approach (consumer graph)
- For example, a consumer graph representing different purchases across different organizations, relationships, including information like dollar amount or date of transaction. Any persona can look at this view from a distance and understand intuitively what it represents fairly quickly (compared to a simple table of data without any visual representation).
- This is even more critical when factoring in big data or perhaps sensor data- when managing and understanding those relationships become so key that it’s difficult to do it in many cases within a relational system, or those systems may drive more of a waterfall type approach. And you wind up losing a lot of that agility that allows your business to continue to compete as required.
Metadata & graph databases
Industry trends point to semantic metadata areas as a key enabler, where again business and IT sides of the organization can come together to understand the problems that they’re trying to solve and to make these projects more strategically focused.
At the core of these projects: features like business glossary, modeling, mapping between higher level logical models and lower level physical models and data sources.
Putting a graph database as the center of all this enables the true understanding of these relationships, focusing on relationships between key entities.
This is the intersection between your different data sources, databases and data records. Where the intersection exists, it provides that key knowledge to deliver context to your enterprise for democratizing that data.
The graph database offers us a new way to organize this customer information and it drives towards the key strategic aspects of Customer 360. Enabling that agility across the business to help contextualize and continue to evolve their views of their customers, their understanding of customers and doing it faster than their key competitors to unlock that value and drive those strategic priorities- such as digital transformation and omnichannel customer experience.
A lot of that insight is hidden away in these silos and therefore difficult to bring to bear on these strategic priorities, so the ultimate goal is really driven towards strategic Customer 360 and its graph technology.
Let’s take a step back to define what a knowledge or a property graph is – as these terms are used somewhat interchangeably. There are different kinds of forms of graph databases.
There are basically three key concepts:
- Entities which are sometimes referred to as “nodes.”
- Relationships which are the links between those nodes or entities.
- Properties that may belong to each of these. Whether thinking of customers, products or organizations, they all have different characteristics you’re looking at modeling. These can all be represented as either entities or relationships between those entities.
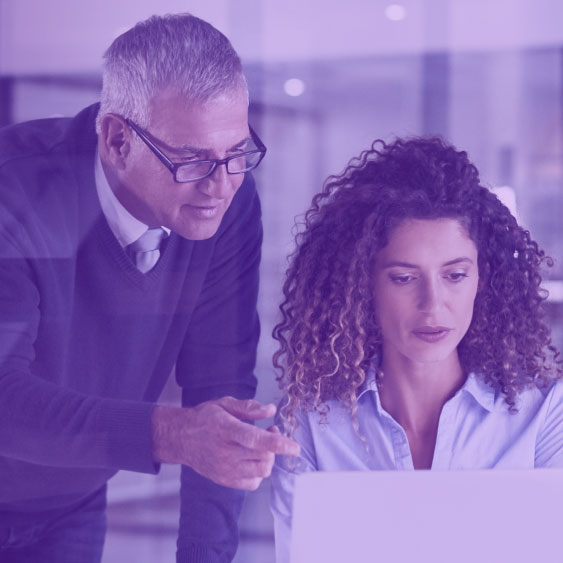
Graph database applied – Customer 360
If we look at how graph database is applied in Customer 360 or master data management projects, it’s worth noting a few key trends:
Graph databases are key enablers for both data and metadata management. Understanding the relationships between your data elements as well as the relationships between your data sources.
If we look at metadata management problems like data lineage or impact analysis, if I’m looking at a report, what is the source of this data? I’m thinking about changing column name and the state of database. What impact does it have downstream, when looking at the flow of information across the enterprise? This boils down to analyzing a graph-based view of your enterprise, information, architecture or ecosystem.
This is where we really drill in to understand those relationships again, not only between your data elements, your data records, but between your data sources and the consumers of that data.
The graph database drives that agile information management approach forward, providing a lot of flexibility, to enable these kinds of projects.
Graph visualization provides us a better way to understand clients’ behaviors- delivering a more accurate representation of that data and the associated connections or relationship between it.
Furthermore, graph technology is very versatile. From a modeling perspective, it’s very performant from a complex query perspective.
Let’s take a typical example like a social network: we have ourselves, our friends and their friends. If you look at how that might be represented in a relational database and you look at how a query might be represented to pull that information out, it gets complex with just one or two hops.
One of the great things about graph is that it works on the concept of a traversal, which means you land in a specific place and then you traverse outwards.
This delivers performance and versatility when it relates to highly connected and dense networks of information, which is virtually all customer information these days with the specific benefits of visualizing data in more of a graph-based way.
Graph visualization – uncover context in hidden relationships
Graph visualization is great at providing those perspectives on your data that are difficult to see with tabular or charted data. It’s a very dynamic way of exploring data. It adds a lot of ability to provide context around specific lines of business. Different types of models for different areas of the business are different integrating applications. APIs allow applications to find patterns in data in more of a graph-based way.
For example, with fraud prevention, there are key patterns we look for in terms of how data is connected within a network to establish fraud activity. We can enable those kinds of queries through API to plug them into other applications to provide alerts.
The visualization aspect on top of modeling and query building supports clearly communicating the requirements between business and IT and supports the strategic business objectives to translate required changes or work to do within your data and information architecture.
Metadata management – key to establishing context
Let’s conclude by looking at the initial steps in the process that we outlined earlier in this book: understanding the business requirements and how we map those to our existing IT and data ecosystem.
Most users struggle to find the data that’s going to deliver that value to their business. They spend a lot of time either locating the data or getting permissions to view data that they may already have permissions to view. They’re just not aware of it.
The data is constantly expanding. It’s constantly changing. It creates a lot of headaches in terms of finding out where the data is, integrating the data together. There simply aren’t very many organizations that truly have a handle on this problem- which is limiting their ability to move their strategic objectives forward.
We really need to enable these users in their quest to find the data in what is effectively a very complex and very distributed environment.
And we have to strike a balance as we build the systems out to support these end users, between connecting or collecting data – trending more and more towards a connect versus a collect strategy.
This means not necessarily undertaking heavyweight mastering multiple times a day of multiple systems, rather doing that in a way that provides key links to the other things that can be pulled in dynamically.
Transactional or clickstream data is updated minute to minute. We may not want to master that multiple times per day but rather reach out and get it more dynamically.
From modeling down to mapping, reporting and visualizing data, being able to understand the metadata context of data is critical and drives towards self-service use cases – whether for reporting or analytical purposes, with a focus on deploying high-level entities and high-level models that business users can access.
How to drive strategic priorities and deliver value:
What are the key strategic enablers as it relates to managing information and data?
- Understanding relationships through knowledge graphs or property graphs, from the modeling, visualization and querying perspective.
- Building the trust on data: verifying that it’s accurate and clean
- Getting the appropriate context set around data.
- Geo – and spatial enriching data – visualizing entities on top of a geospatial representation or leverage geocoding and boundary data like a map, we can also use graph specific algorithms to analyze that data to get more of a spatial perspective and add further context, adding fuel to our context driven approach.
- Rapid prototyping and iterative development deliver fast and efficient results to the business – demonstrating its value in a much quicker way.
Graph models shine in promoting understanding of the relationships between data and metadata, ultimately moving towards more of a self-service based capability to provide flexibility to both the technologists and the business side of the house – supporting a capability to self-serve data around key requirements and priorities.
End-to-end customer data management
Business and technology strategic enablers
For the business
- Deep understanding of relationships in data through knowledge graph enabled modeling
- Highly accurate and “clean“ data to advise strategic business decisions
- Location intelligence to deliver contextually relevant views of customer
For the technologist
- Agile information management approach supports rapid prototyping to help technologists deliver fast, efficient results
- Graph models enable technologists to better understand relationships between data
- Self-service and SaaS capabilities provide ultimate flexibility for busy technologists
Conclusion
In this eBook, we discussed processes that are involved when building Customer 360 views, and specific benefits both graph databases and metadata management provide for those types of projects.
This all starts with a clear understanding of the requirements and all related upfront work, like defining data sources and integrating them. Building your data quality rules and the enrichment around location intelligence. All of those are key elements. Virtually any Customer 360 or master data management project needs to provide these end to end capabilities to help any business drive more effective customer engagement, fraud detection, risk assessment – effectively any priority that requires that you get a better understanding of your data will benefit from this type of approach.