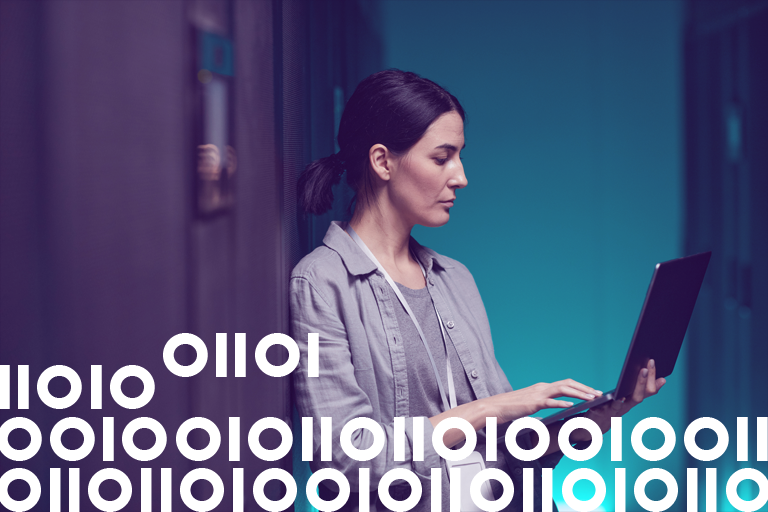
Mainframe Data: Empowering Democratized Cloud Analytics
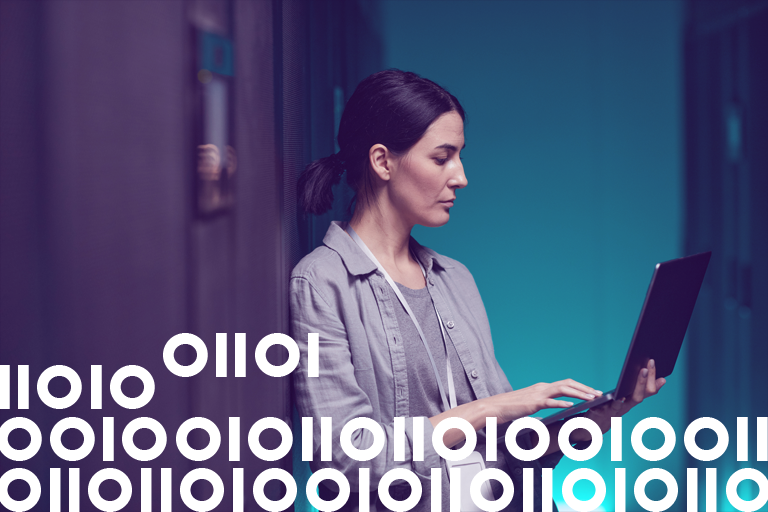
Rapid advancements in digital technologies are transforming cloud-based computing and cloud analytics. Big data analytics, IoT, AI, and machine learning are revolutionizing the way businesses create value and competitive advantage.
For organizations that continue to rely on mainframe systems to process their most mission-critical workloads, the digital revolution demands new approaches to integration. Businesses must tear down the barriers that stand between their core data assets and the globally distributed computing systems driving today’s economy.
IT leaders must navigate this transformation by implementing foundational system changes while also ensuring operational efficiency. How can today’s mainframe shops navigate these changes, address the challenges of mainframe integration, and come up with practical strategies for merging their mainframe data with cloud environments?
The Rise of Cloud-Based Computing
Pivotal changes can often be abrupt and unsettling. Typically, such shifts are the end result of an extended evolutionary process. This is certainly true in the rise of cloud platform-as-a-service computing. Several important trends have influenced this shift:
Budgeting methods and priorities: In recent years, large organizations have increasingly emphasized direct accountability for measurable profits or cost savings during their budgeting processes. Initially, this led to a preference for SaaS and PaaS over investing in IT infrastructure and personnel. Later, this trend shifted the responsibility for IT functions to the specific departments using them.
Consequently, managers now oversee IT costs for their operations and engage directly in cloud computing contracts. This shift has influenced how cloud resources are designed and marketed, focusing on easy access, modularity, and straightforward deployment.
The data-driven enterprise: Another key trend centers around a deeper understanding of data and its vital importance in creating and sustaining competitive advantage. Organizations have come to understand that they can use both internal and external data to drive tremendous business value.
The cloud is especially well-suited to large-scale storage and big data analytics, due in part to its capacity to handle intensive computing requirements at scale. BI platforms and data warehouses have been replaced by modern data lakes and cloud analytics solutions. Virtually every recent advancement in cloud computing, from IoT to chatbot AI systems, is connected in some way with a seemingly unstoppable tidal wave of data and a compelling need to access and interpret it.
Data democratization: Executives and managers have come to understand the value that data analytics can bring to virtually every business function throughout an organization. As a result, there is a widespread desire for access to timely, trustworthy data and the analytical tools to interpret it. This has compelled business users to expand their knowledge of data management and enhance their analytics skills. It has also highlighted the need to democratize data access and management, offering more open access to data that was previously unavailable.
Read our White paper
Empowering Democratized Cloud Analytics with Mainframe Data
In this paper you will find straightforward explanations of why new technologies and business trends are forcing IT leaders to open up mainframe data access; how the unique architecture of mainframe systems makes doing so very challenging; and practical advice on achieving the most important goals for integrating mainframe data into cloud environments.
Democratizing Your Mainframe Data
For IT leaders overseeing corporate data, the shift toward democratized, cloud-based operations presents significant challenges and risks. Integrating mainframe data is imperative, especially considering the nature of the core transactional data these systems house.
For those managing mainframe systems, bridging the gap between traditional mainframe operations and the cloud-native future might seem daunting. Yet, by adopting an evolutionary strategy and leveraging cutting-edge tools available today, the transition can be relatively seamless.
Business users want flexibility, effortless access, and quick solutions to their problems. That means having systems that are intuitive and easy to use, without dependencies on the IT department. For technology leaders, such systems must have structure, without being overly rigid. We call that “structured flexibility.” It presents some challenges, but it is certainly attainable.
Data integrity is also essential. Users expect immediate access to trustworthy data, but IT leaders know that this can be difficult. That means that for data to be trustworthy and ready to power the enterprise it should be accurate, timely, and contextually relevant. Consistency, accuracy, and completeness are aspects of data integrity, of course, but true data integrity extends much further than just data quality. The broader access granted by data democratization amplifies both the importance and the challenges of maintaining data integrity.
The Role of Data Governance
Data integrity and data democratization are critical to any successful cloud analytics initiative. Without a meaningful data governance framework, though, data democratization has the potential to negatively impact data integrity.
In a connected mainframe/cloud environment, data is often diverse and fragmented. The cloud environment must handle structured, unstructured, and mainframe data sets, as well as real-time data from connected devices. Democratizing data means allowing users to integrate external data sources such as marketing lists and supply chain information. This can add stress to data management teams and data governance processes.
The movement of data also grows more complex with data democratization. Secure data exchange takes on much greater importance. Managing data integrity is not just about network security; it also requires that organizations maintain high standards for data quality. The methods that work well on a mainframe don’t necessarily translate effectively to democratized data in the cloud. Moreover, mainframe data, with its unique formats and security controls, render data democratization even more challenging.
Maintaining clear data lineage with mainframe data can also be difficult. COBOL copybooks, crucial for mainframe data, are stored separately from their corresponding data and may contain complex logic. Ensuring data lineage (a record of all data changes) is vital for recovery and regulatory compliance. Integrating mainframe data into the cloud magnifies the challenges of metadata management, especially with the rapid growth in the amount of metadata.
Keys to Successful Democratization of Mainframe Data
Successful data democratization requires effective system integration decisions. Prioritize solutions that offer flexibility and ease in data sharing, allowing for streamlined creation and testing of data models. Look for tools that support a “Design Once, Deploy Everywhere” approach, ensuring compatibility with various cloud platforms, evolving business processes, and new use cases. Additionally, the ideal integration solution should seamlessly meld with current systems, emphasizing real-time data observability to proactively address potential issues.
Precisley’s Data Integration Service is an essential element of the Precisely Data Integrity Suite, an integrated, interoperable suite of enterprise-grade tools for designing and delivering accurate, consistent, contextual data for advanced data-driven decision-making.
To learn more about how Precisely can help you democratize your mainframe data, download our free whitepaper today, Empowering Democratized Cloud Analytics with Mainframe Data.