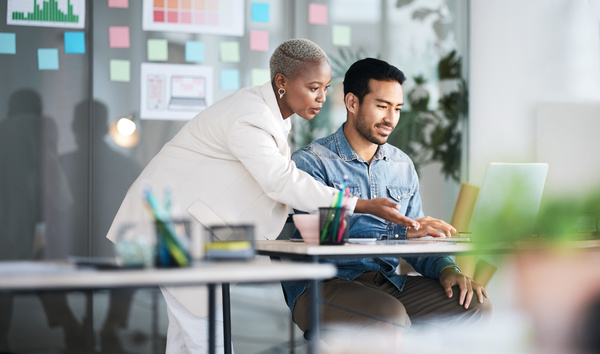
Why Your Master Data Management Needs Data Governance
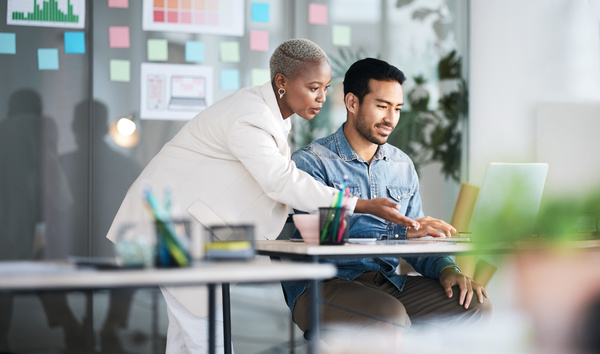
Data is pivotal for the success of business operations. With cloud computing, the capacity to extract value from data is greater than ever. As this realization grows, businesses are shifting their investments from hardware to technologies that optimize data assets. Master Data Management systems (MDM) play an important role in harmonizing data assets across large and midsize enterprises. However, to get optimal value from your organization’s data, you need to apply the discipline of data governance to your MDM.
MDM – Not the Silver Bullet
The goal of MDM is to identify, validate, and resolve data issues as close to the source as possible while creating a “Gold Copy” master dataset for downstream systems and services to consume. Many businesses have multiple systems that house customer data, for example. That may include ERP, CRM, digital marketing automation, customer service applications, and more. MDM offers a unified reference point that can harmonize and synchronize the customer information stored and maintained in those various systems.
An MDM consolidates important domain data into unique or linked instances (e.g. a “golden” record) and then uses that unique record as a reference point for aggregating associated data, purging duplicates, standardizing data across various applications, and creating rules to continuously resolve, merge, or disassociate records.
MDM helps ensure the consistency, completeness, and accuracy of shared data sets. Nevertheless, MDM is not a silver bullet for data quality. Generally speaking, it applies to a relatively limited subset of an organization’s internal data – namely, business entities such as customers, vendors, and inventory items, for example.
Although MDM serves a vitally important function by ensuring the shared master data is managed correctly and is fit for purpose, it does not offer the same benefits as a full-blown data governance program.
In addition to managing master data, every business must also handle a variety of data from other sources. That may include transactional and operational data, reference data, metadata, and audit information, for example. To be a truly data-driven organization, it’s important to govern all of the above.
Read our White paper
A Roadmap for Data Governance
This white paper explores four steps of the data governance journey, and how doing it right will payoff in the long run.
Data Governance: Casting a Wider Net
To maximize the value of your organization’s data assets, you need more than MDM. With data governance and the right technology tools, it is possible to achieve consistent, accurate results at scale.
Here’s what data governance adds to the bigger picture:
- Definition: What does a particular term mean? What is the language that users throughout your organization use to describe the data they work with every day? Are there different names, phrases, or abbreviations used by different groups throughout the company?
- Data classification and retention policies: Data may be classified in many ways based on both internal and external policies. These can further drive usage rights, disclosure, and disclaimers. Such classifications can also have profound implications with respect to regulatory compliance.
- Data lineage: Where did a particular record come from? Are there multiple data points that could affect a given dataset? What business rules dictate the priority of one record or system over another for creating or maintaining the so-called “golden” record? Do you have sufficient visibility into data lineage to support compliance?
- Search: What data is available and from where? Is it shared? Which business functions are using which set(s) of data? Who should have permissions to access specific datasets and records, and in what form?
- Collaboration: How can people become more knowledgeable about organizational data? How can they contribute their expertise? How can data governance leaders encourage users to take ownership of their data and processes while also collaborating effectively with other departments?
- Enterprise data quality: How trustworthy are the various types of data in use? Do you have high data quality? In other words, is it consistent, accurate, and complete? Is there a pattern or trend to the various domains of data?
- Operating models: Which policies, procedures, and resources will ensure smooth operations and drive quality, security, access rights, sourcing, and the proper use of data throughout the organization?
- Responsibilities: Accountability is key to the effectiveness of any organization. Assigning people to roles is one component, but what are the expectations? How do you establish models of interaction and measure the efforts of staff members navigating through the cultural, political, and personality landmines to ensure the optimal use of an organization’s resources and data?
For MDM to be truly effective, it must exist within the context of a proper data governance framework. Data governance ensures that corporate data assets are clearly understood from the perspectives of definition, sourcing, quality, and accountability.
Large-scale data-driven initiatives require data governance from the outset and at every phase of the project. Integrated Data Governance can also ensure the business backing and active participation in initiatives that are often perceived by the business as a technology exercise owned and operated by IT.
TechTarget sums it up nicely, noting: “Data governance and master data management need to be aligned. A master data management strategy in the absence of a solid data governance program is likely to encounter difficulties because the heart of such a project is to agree on a common golden copy of key data across an enterprise.”
The Value of a Business Glossary
In much the same way that organizations inventory their physical assets, data assets also need to be properly clearly defined, inventoried, managed, and open to collaboration. Organizations typically start this process with internal solutions leveraging spreadsheets, SharePoint, or other homegrown solutions.
Most organizations outgrow these ad hoc approaches very quickly. As the number of data assets and systems increases, and as an organization grows, it gets increasingly difficult to track lineage, workflow, impact analysis, or collaboration capabilities for the various data governance roles.
A data glossary serves as the glue that ties the data governance capability into the MDM project, ensuring business participation, accepted business term definition, and assigned and documented accountabilities for the governance of the mastered domains.
Knowing upfront MDM’s capabilities and especially its limitations can help an organization to incorporate solutions that provide a full 360° view, understanding, and transparency into their corporate data assets.
To learn more about how a comprehensive enterprise data governance solution can help you solve organizational data quality challenges, read our whitepaper A Roadmap for Data Governance.