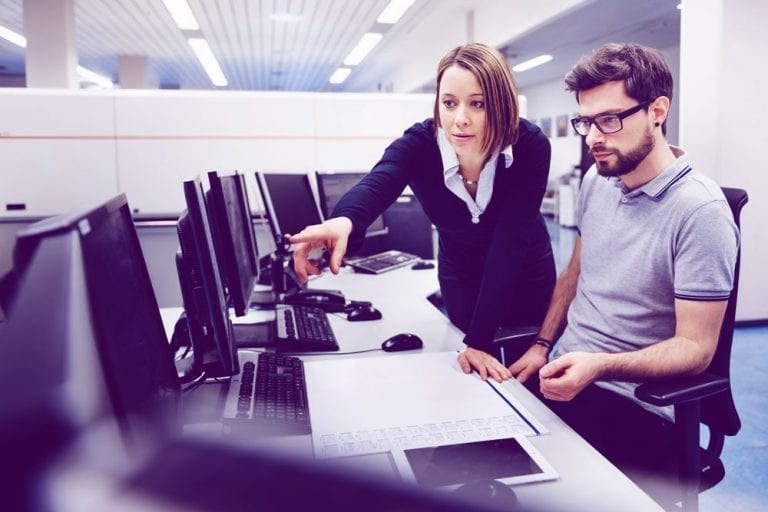
Maximizing Data Value through Integrated Data Governance and Data Quality
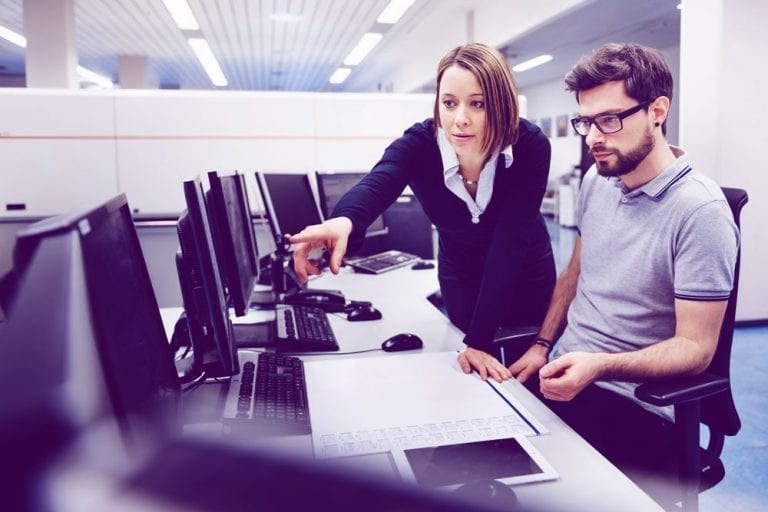
The costs of bad data have had a significant impact across the business world. As reported by Gartner’s Data Quality Market Survey, poor data is responsible for an average of $15 million in losses annually. These staggering numbers come as no surprise when you consider the massive volumes of data being created, ingested and transformed daily.
The continuous struggle to maintain data integrity and maximize data value is the biggest challenge many organizations face in data management.
The risks of bad data are incredibly high. Bad data breeds distrust among data users, and prevents them from leveraging it to gain critical business insights. Inaccurate data can quickly pollute downstream processes and proliferate throughout an organization’s data supply chain. And the idiom “garbage in, garbage out” aptly applies to data analytics, where poor quality data can lead to flawed business intelligence.
Businesses today need more than a basic data quality tool to ensure data asset value. They need a comprehensive strategy that uses advanced controls, quality scoring, and monitoring, rooted in a framework of data governance. Integrating data governance and data quality is a critical step to a successful data management strategy.
Integrating data governance and data quality
As data is created internally or absorbed from a third party, a top priority needs to be verifying and maintaining its integrity. While traveling through the data supply chain, it’s exposed to new processes, procedures, transformations, and uses, which all impact data quality. This data must be tracked and reconciled to monitor the attrition of data across the process.
It’s crucial to proactively solve data integrity issues before poor data quality creates significant problems and negatively impacts your customer experience, brand reputation, operational efficiency, and business decisions.
Read the Whitepaper
Overcome Business Compliance Challenges with Better Data Quality and Data Governance
Read this whitepaper and explore how the intersection of data quality and data governance can help you overcome compliance challenges while offering significant business benefits.
Enterprise data governance provides a foundation for protecting data integrity across the data supply chain. It establishes the people, technologies, and processes to empower business users to easily understand, access, and apply enterprise data for business purposes. Data governance also educates data consumers regarding data usage, meaning, and quality levels, to build trust and encourage data utilization.
But, that trust can’t be built without robust data quality controls to ensure reliable and accurate data.
Establishing baseline data quality
Data governance and data quality go hand in hand. So to ensure data integrity enterprise-wide, data quality checks should be embedded directly in your data governance framework. These checks fall into the following five categories:
- Traditional checks for data quality ensure the completeness, consistency, and conformity of data. These validations are critical for measuring the quality and trustworthiness of data being used for business intelligence and analytics – without them, you’ll lack the confidence you need to make good decisions.
- Controls for balancing and reconciliation assure that data arrives accurately at its expected location. These validations are used to monitor critical business processes. Missing or inaccurate data can mean everything from lost revenue, to reputational damage.
- Timeliness checks are also necessary to monitor when files arrive and flag any late or missing files. Timeliness validations are valuable when dealing with third party data. SLAs need to be monitored to ensure third parties are not negatively impacting your business.
- Statistical controls are essential to validate data sets based on statistical values, like expected standard deviation or industry-defined methods.
- Reasonability checks are vital to affirm data values meet defined and expected thresholds. Statistical and reasonability checks allow businesses to find data anomalies that otherwise may go undetected by traditional checks.
Enforcing data quality checks within your data governance framework is an easy way for your organization to score and monitor data integrity – preventing data issues and building trust among business users. Still, while data governance and data quality are undoubtedly tightly interwoven, governance is about so much more.
Data integrity is only half the equation
Data governance is also about building data understanding across the business. Here, there are a few different approaches you can take:
- Top-down: this is a good starting point to understand where you need to have data quality rules. You’ll identify what the critical processes in the business are, then determine which applications and data are critical in those processes.
- Middle-out: identify any regulatory policies, look at the processes and data associated with those policies, then implement data quality checks accordingly.
- Bottom-up: discover and profile your data. This gives users an understanding of what’s available and where validations are needed to improve the data.
Data understanding ensures that data is used appropriately to maximize value and minimize risk. On top of that, you also could gain a competitive advantage thanks to business users feeling more empowered to increasingly leverage data assets for analytical insights.
Organizations that take advantage of the synergies between data governance and data quality have a stronger data management strategy that generates better analytics results. Data integrity checks ensure the quality of data before use, while data governance provides business users with easily understood, well-prepared data. By combining data quality with data governance efforts, business users can quickly develop meaningful insights from their data to spur organizational growth.
Read this whitepaper Overcome Business Compliance Challenges with Better Data Quality and Data Governance and explore how the intersection of data quality and data governance can help you overcome compliance challenges while offering significant business benefits.