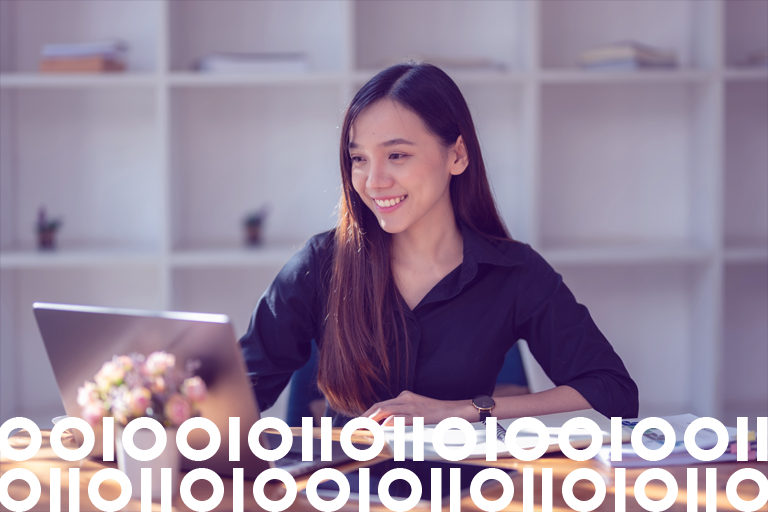
Gain an AI Advantage with Data Governance and Quality
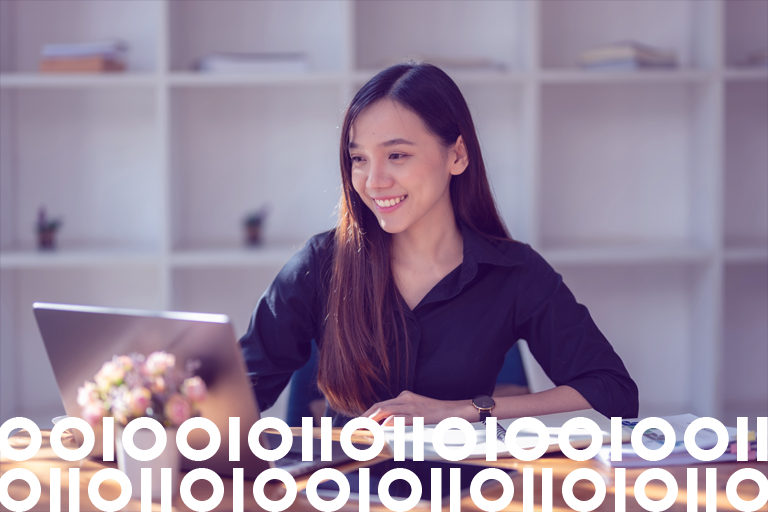
Key Takeaways
- Data quality ensures your data is accurate, complete, reliable, and up to date – powering AI conclusions that reduce costs and increase revenue and compliance.
- Data observability continuously monitors data pipelines and alerts you to errors and anomalies. Then, you can correct them before they’re introduced into an AI system.
- Data governance ensures AI models have access to all necessary information and that the data is used responsibly in compliance with privacy, security, and other relevant policies.
Have you ever wondered why some artificial intelligence (AI) initiatives fail while others soar? The answer often lies in the quality of data feeding those AI models.
That’s because AI tools are prime examples of “garbage in, garbage out” technologies. Poor data quality leads to inaccurate AI results, which can damage customer experiences, increase risks, and inflate costs.
To maximize your investments in AI, you need to prioritize data governance, quality, and observability.
Solving the Challenge of Untrustworthy AI Results
AI has the potential to revolutionize industries by analyzing vast datasets and streamlining complex processes – but only when the tools are trained on high-quality data.
Poor data quality erodes trust in your AI predictions and recommendations, which can slow down adoption and innovation. So, the risk of entering into these initiatives without taking care of your data first is simply too high.
Effective AI implementation requires data that’s accurate, consistent, and fit for purpose – which you gain with a proactive approach to data quality, robust data governance, and data observability.
Data governance for AI
Data governance helps manage your data assets, ensuring compliance with data privacy regulations and providing insights into data collection, storage, and usage.
A strong data governance framework is the foundation of a comprehensive data quality solution to ensure trustworthy AI. Having this framework in place is crucial for maintaining data privacy and security – enabling you to monitor data usage and ensure compliance with regulations related to personally identifiable information (PII).
With data governance, you also get the insights you need to understand data ownership, meaning, and quality, along with a clear understanding of how the data you use in AI applications is:
- collected: what are the data types?
- stored: where is it located?
- used: who has access to it?
Ultimately, data governance ensures the trust that’s crucial for accurate AI-driven decisions and reporting.
Data quality for AI
Remember the “garbage in, garbage out” adage? Let’s reiterate again: for AI to deliver trustworthy outcomes, the data it uses must be high quality. What does “quality” data mean, exactly? It needs to be accurate, complete, reliable, and timely.
Let’s break down those four characteristics.
- Accuracy: Accurate data ensures correct information, reducing the risk of erroneous AI conclusions or hallucinations. For example, inaccurate customer data can lead to misguided personalized recommendations, resulting in frustrated customers and lost revenue.
- Completeness: Comprehensive data prevents gaps that could bias AI outputs. Does your data cover all necessary aspects to inform your AI models accurately? Incomplete data can skew results and lead to faulty conclusions.
- Reliability: Have you ever encountered conflicting data from different sources? Reliable data aligns with other trusted sources, ensuring consistency across AI models.
- Timeliness: Up-to-date data ensures AI models make decisions based on the latest information, enhancing their relevance and effectiveness. Outdated data can lead to invalid decisions, which impacts AI performance.
Effective data quality management requires sustainable practices. Automated controls can validate new and updated data against real-world criteria before it’s used. Consider implementing automated practices like:
- data validation to ensure new data conforms to established standards before acceptance, saving time and reducing human error.
- deduplication to prevent bias and inefficiencies in AI models. Duplicates can inflate data volumes unnecessarily and skew results.
- data reconciliation to synchronize new data with existing datasets, ensuring consistency and accuracy. This is crucial for maintaining AI model integrity.
Read eBook
Trusted Data, Powerful AI: Driving Better AI Outcomes through Data Quality and Governance
Are you ready to transform your business with AI you can depend on? Read this eBook to dive even deeper into these use cases and concepts.
Data observability for AI
Traditional data management methods can’t handle the vast amounts of data required by AI. That means that when errors and anomalies occur, they’re more likely to go unnoticed – and the longer they’re undetected, the longer you’ll have flawed AI results.
To identify and correct anomalies before they impact your AI models, you need data observability tools that use advanced AI/ML techniques to proactively monitor your data pipelines. Then, they can immediately alert the appropriate users of any issues – including data drift (changes to data distribution over time), which can impact model accuracy. Detecting and addressing errors and anomalies faster can help you maintain stronger AI performance.
Automated checks are also key to data observability. Automated tools ensure data transformations are correct and no data is lost or corrupted during transfers, providing you with an additional layer of security.
High-Value AI Use Cases
Organizations across industries are already experiencing the benefits of AI, and the use cases are growing each day. If you’re looking for inspiration on where to start within your own business, think about opportunities like these:
- AI recommendations: Fast, personalized recommendations enhance user experiences by suggesting tailored products, content, or services. High-quality, governed data ensures these recommendations are relevant and useful.
- AI-powered workflows: Automating workflows with AI increases productivity, efficiency, and reduces costs. Reliable data integration from multiple sources is essential.
- Machine learning applications: ML accelerates business processes with greater accuracy. Data quality and observability are critical for training reliable ML models.
- Foundation model training: Pre-trained on large datasets, foundation models enable capabilities like natural language processing. High-quality data prevents harmful biases and ensures fair outputs.
- Chatbots: AI-powered chatbots deliver efficient, personalized assistance that enhances user engagement. Training chatbots on high-quality data ensures they provide accurate responses.
Trust Your Results with Data Quality, Observability, and Governance for AI
Success in AI initiatives hinges on the quality of your data. By implementing robust data governance, ensuring high data quality, and leveraging proactive data observability tools, you can trust your AI systems to make accurate and informed decisions. These practices not only enhance AI outcomes but also drive better business results.
Are you ready to transform your business with AI you can depend on? Dive even deeper into these use cases and concepts – read the eBook, Trusted Data, Powerful AI: Driving Better AI Outcomes through Data Quality and Governance.