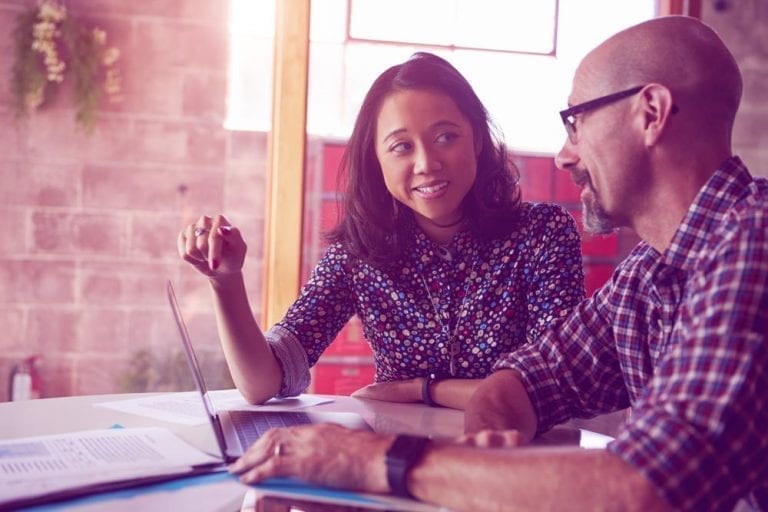
What is Data Quality? Explaining What Data Quality Actually Means
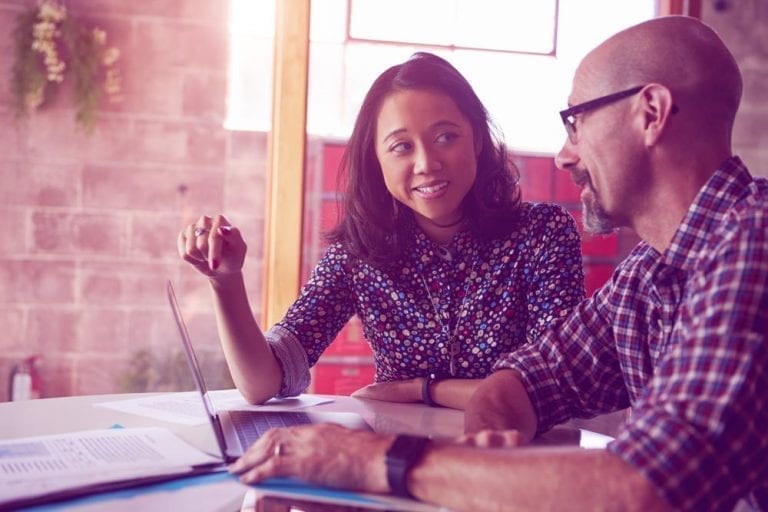
If you work with data, you’ve probably heard the term more than a few times, but what is data quality? Do you know what it actually means, and what data quality analysts do? If not, this article’s for you.
It may not be quite as popular a buzzword as big data, but it’s an oft-used term in the data world. Data analysts like to remind everyone that having quality is essential to derive value from data.
But they don’t always take the time to define it or provide real-world examples of the types of problems that data quality tools correct. So, let’s take a look.
What is data quality? A definition
Data quality can be defined as the ability of a given data set to serve an intended purpose.
To put it another way, if you have high quality, your data is capable of delivering the insight you hope to get out of it.
Conversely, if your data is of poor quality, there is a problem in your data that will prevent you from using the data to do what you hope to achieve with it.
Examples of common challenges
To illustrate the definition further, let’s examine a few examples of real-world challenges.
Imagine that we have a data set that consists of names and addresses. Data like this is likely to contain some errors for various reasons – both simple and complicated ones.
Simple causes of data errors are names and addresses that were entered incorrectly, or address information that has changed since it was collected.
There are other, more complicated problems that may exist in the data set. One is entries that are ambiguous because of incomplete information. For example, one entry might be an address for a Mr. Smith who lives in the city “London,” with no country specified. This is a problem because we don’t know whether the London in which Mr. Smith resides is London, England, London, Ontario or one of the other dozen-or-so cities around the world named London. Unless you use a data quality tool to correct this ambiguity, you’ll face difficulty using your data set to reach Mr. Smith.
As another example of a complex problem, consider the issue of seemingly redundant addresses within the data set. Let’s say we have multiple entries in our database for people named Mr. Smith who reside at 123 Main Street. This could be the result of a simple double-entry: Perhaps the data for Mr. Smith was entered more than once by mistake.
Another possibility is that there are multiple Misters Smith – a father and son, perhaps – residing at the same address. Or maybe we are dealing with entries for totally unrelated men who both happen to have the same last name and reside at 123 Main Street, but in different towns. Without correction, there’s too much ambiguity in a data set like this to be able to rely on the data for marketing or customer-relations purposes.
Read our eBook
4 Ways to Measure Data Quality
See what quality assessment looks like in practice. Review four key metrics organizations can use to measure quality of their data
Fixing problems
One way to correct data quality issues like these is to research each inconsistency or ambiguity and fix it manually. That would take a huge amount of time, however. It’s not practical on a large scale.
A much more time- and cost-efficient approach is to use automated tools that can identify, interpret and correct data problems without human guidance. In the case of a data set composed of names and addresses, they might do this by correlating the data with other data sets to catch errors, or using predictive analytics to fill in the blanks.
The never-ending battle
Because data quality is defined in terms of a data set’s ability to serve a given task, its precise nature and characteristics will vary from case to case. What one organization perceives as high-quality data could be rubbish in the eyes of another organization.
Understanding how quality changes based on context is important because it means that it is not something you can simply obtain and keep. You may have it today but lose it tomorrow if your goals change and your data in its current state can no longer meet them.
So, think of data quality as a never-ending battle. It’s something you need to be constantly working on and improving to ensure that your data is ready to meet whichever tasks you throw at it.
Using Precisely to trust your data
As organizations liberate data from traditional silos across the enterprise and centralize it in data lakes for high-powered analytics, data governance is becoming a top priority, especially in highly regulated industries, such as banking, insurance, financial services and healthcare.
Precisely has combined the power of high-performance data integration software to quickly and efficiently access data from any source and load it into the data lake, while using data quality tools to profile that data.
How good is the quality of your data? Find out by reading our eBook: 4 Ways to Measure Data Quality