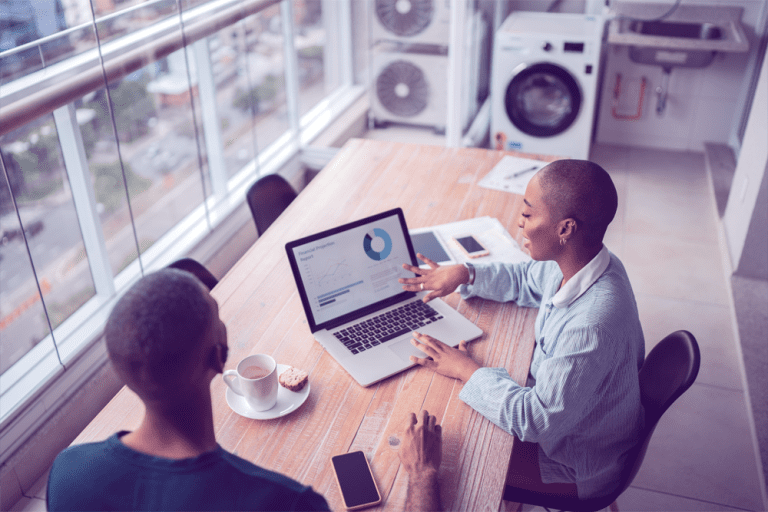
Kickstart a Data Quality Strategy to Build Trust in Your Data
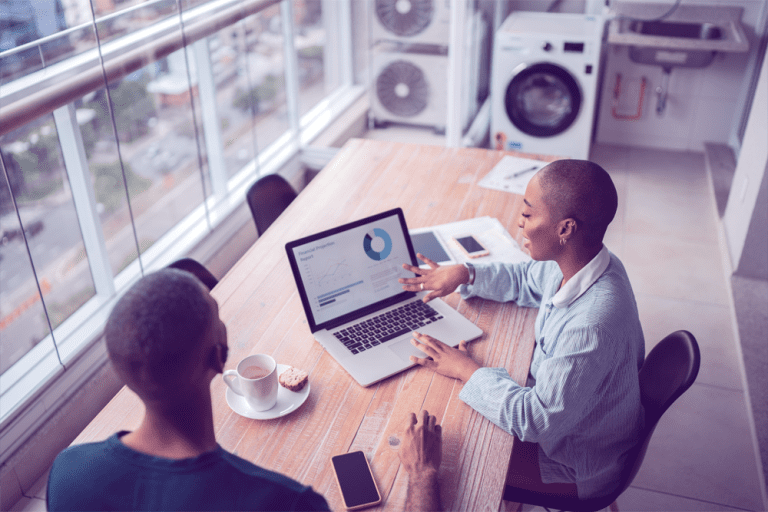
Data quality strategy plays a foundational role within the broader context of data integrity. From the executive suite to the front lines, the people who rely on analytics to help them make important decisions must know that they can trust the integrity of the underlying data. Establishing that trust typically begins with a good data quality strategy and data lineage tools that profile and catalog your enterprise data. This creates a strong foundation on which to build confidence in data-driven decisions.
Data integrity is about accuracy, consistency, and context. Accuracy defines whether or not the data is factually correct. For example, is this the customer’s correct name and address? Consistency determines whether or not the data is harmonized across and within systems. For example, is address data represented in various systems according to postal standards, using the same street names, spellings, and abbreviations? Context refers to the completeness of datasets, such that they reflect all of the dimensions that may be relevant to decision-makers. This includes factors based on location, for example, or details that shed new light on customers’ buying behavior.
The terms data quality and data integrity are often used interchangeably, but there are some important distinctions. Data quality is a foundational component of data integrity, along with several other pillars – namely, data integration, location intelligence, and data enrichment. Data integration eliminates silos and connects data throughout the organization. Location intelligence provides valuable additional details offering geospatial insights. Data enrichment provides contextual nuance and meaning by enriching data with information from external sources.
What Is Data Quality?
If data integrity is built on the four pillars of data quality and governance, data integration, location intelligence, and data enrichment, then what exactly is data quality and how is it different from data integrity? The answer lies in understanding the various components of a sound data integrity strategy. Business leaders must concern themselves with the following questions:
- Understanding. Where does my data live across the enterprise? What’s semantic entities or domains are represented? What sorts of rules and transformations need to be applied to the data to really make it fit for use? Are we using data governance and catalog tools to thoroughly understand our data?
- Measuring. What are the key performance indicators (KPI) we need to measurement across the enterprise? What critical data elements are needed to support those KPI’s.
- Monitoring. How should we be monitoring those measurements? What aspects of data quality need to be monitored in real time for alerting and remediation?
- Cleansing. How can we manage the various cleansing operations that must be performed on our data– for example, validating the address in the customer record, such as standardizing the name and perhaps merging records wherever we find duplicates?
- Governing. How can we create and support enterprise-wide policies and standards that will enable us to optimize data usage across all of the different systems within our organization?
An effective data quality program requires a deliberate, systematic, and sustained approach. At Precisely, we often say that data integrity is a journey, but that an effective data quality strategy is a critical step early in the process. But unlike a linear journey, the path to data integrity is an iterative process that requires an ongoing commitment to excellence. It also needs to be agile, and it requires best-in-class solutions to support collaborative effort in ways that deliver real value to the business.
Challenges of Poor Data Quality
Unfortunately, most organizations continue to struggle with data quality. As the volume and velocity of data continue to increase, the problem simply cannot be ignored. According to the Harvard Business Review, 47% of newly created data records have at least one critical error. As organizations strive to roll out better, faster, more flexible analytics initiatives and AI/ML technology, the impacts of that poor data quality are being felt more than ever. In fact, according to the same HBR survey, 66% of organizations’ data backlog of data debt is negatively impacting their AI/machine learning and analytics aspirations.
Data democratization, likewise, is an important trend, as more companies recognize the value of putting analytical capabilities into the hands of more users. Without trusted data, these kinds of initiatives will stall. Worse yet, they may erode confidence in any future programs aimed at empowering people to make data-driven decisions.
Data Lineage Tools Serve as a Cornerstone
Data quality efforts should be directly tied to the business value they produce. That means setting measurable goals and monitoring outcomes relative to those objectives. The best data quality programs will set target KPIs (key performance indicators) and will monitor those via dashboards and scorecards. This empowers data stewards to track progress, as well as to identify and follow-up on any questions or potential issues. Just as importantly, it equips them to communicate the success of data quality initiatives to executive sponsors by the most compelling means possible: with data.
Once the KPIs have been identified, associated reports and critical data elements can be derived. It is critical to ensure a common understanding of these data elements, as well as to ensure the quality of this data so that users can trust in it. This data should be cataloged and profiled. In parallel, business definitions should be agreed upon and documented. Quality of the data should be made visible to all users, and those who have subject matter expertise should be provided with a method to resolve a variety of validation and cleansing issues. That may include automated processes to standardize and cleanse the data, reconciling the data with outside sources. or applying business rules to ensure the data meets expected standards.
Precisely’s Data Integrity Suite delivers the essential elements of accuracy, consistency, and context by addressing all four pillars of data integrity. Precisely’s modular architecture lends itself to an incremental approach that starts with a data quality strategy and data integration, proceeding to data governance, location intelligence, and data enrichment to further enhance business value. Our world class data lineage tools and solutions ensure that you have a clear roadmap to your corporate data assets. The net result is confidence across the organization – trust in the insights from your data analytics and AI/ML initiatives.
To learn more about data integrity, download our free whitepaper, Get the Lowdown on the When, Why and How of Data Governance.