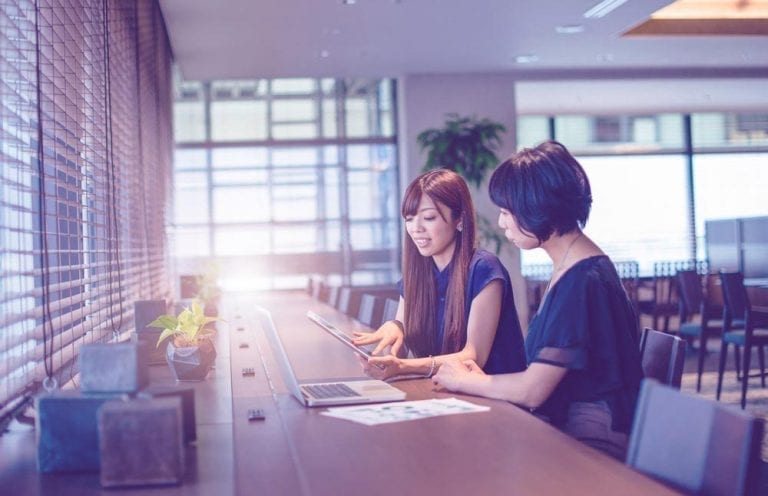
Deep Data is the New Big Data
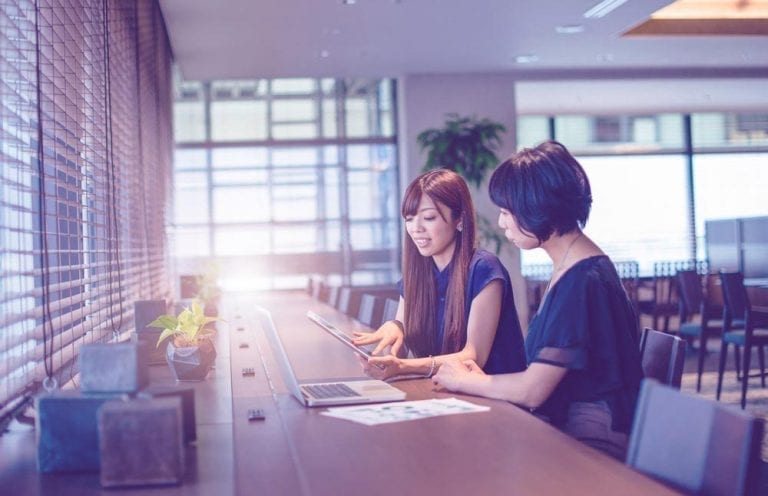
It’s no longer enough for your data to be big. Today, data needs to be deep, too. Here’s why deep data is so essential for enterprise data analytics, and tips for making your data deep.
These days, anyone can collect lots of data. Data collection can be easily automated, and data storage is cheap.
In fact, because we live in an age when everything is digitized, it’s virtually impossible not to collect lots of information. From network switches to remote sensors to customers’ browsing history, everything spits out data at a dizzying pace – and companies need to make sense of that data if they want to understand the trends that power their business.
Big data vs. deep data
Yet simply collecting lots of data is not enough. Large-scale data collection gives you big data – meaning a large volume of data to analyze – but it doesn’t necessarily mean you have data that is valuable.
To be valuable, your data needs to be not just big data, but also “deep” data. The term “deep data” encompasses two essential components: data quality and data integrity.
Data that is collected haphazardly is unlikely to have either quality or integrity. No matter how big the amount of data you collect, you can’t derive much value from it if you are not able to analyze it rapidly to glean accurate, reliable information.
Deep data challenges
Generating deep data can be tough for two main reasons.
First, data quality tends to vary widely. Information might be missing, inaccurate, or inconsistent within a database.
For example, consider the data quality challenges you face when collecting information about visitors to a website. Parts of the data you collect about the technology used by your visitors are likely to be incomplete because some users will be using browsers or operating systems that cannot be identified.
eBook
4 Ways to Measure Data Quality
Learn how to measure data quality and track the effectiveness of data quality improvement efforts. There are a variety of data and metrics that organizations can use to measure data quality. We’ll review of few of them in this eBook.
The data is also likely to contain inaccuracies. For instance, if a customer uses a virtual private network (VPN) to mask his or her geographic location, the data you collect about the geographic origins of website users will not be completely accurate.
Last but not least, the data will be inconsistent if you have collected more information on some users than on others. That could happen if, for example, not all users spend the same amount of time on the site.
The second challenge you face when attempting to muster deep data is constraints on your ability to turn data into action quickly. If you need to translate data from one storage format to another before analyzing it – as you will probably have to do if you have multiple types of systems or platforms within your infrastructure, each of them generating and storing data in different ways – you risk delays that could prevent you from analyzing the data while it is still relevant. Converting between different data formats is likely to introduce data quality problems, too.
The need for immediately actionable data is especially acute today when real-time analytics are often the only type of analytics that can deliver value. If you want to use data analytics to make product recommendations to customers on your website by combining browsing history information collected from your Web server with account information stored on your mainframe, you’ll need to integrate those two data sources, then run analytics on the integrated data in real time. Otherwise, your customers will have left the site by the time your analytics results are ready.
Improve data integrity to capture deep data
In addition to challenges with data quality, there is the challenge of data integrity to consider as well. Though these terms are sometimes erroneously used interchangeably, the two concepts are different. Data quality is a subset of data integrity, but it is not the totality of it.
Data integrity consists of four main pillars. They are:
Each of these pillars deepens the insights you can derive from your data. Think of each pillar as a layer of information from which you can gain a richer, more nuanced understanding of your data. Without adding data integrity to the mix, you will never achieve truly “deep data.”
The cost of shallow data
Just how much can “shallow” data eat into business value?
Data scientists can spend up to 90 percent of their time cleaning up bad data. That’s a lot of effort that would be better spent analyzing data, rather than preparing it to be analyzed. Poor data undercuts marketing efforts.
Data is an invaluable strategic asset that can make or break long-term success. Trillium Quality can help you transform high-volume, disconnected data into trusted and actionable business insights with scalable enterprise data quality
Read our eBook, 4 Ways to Measure Data Quality, to learn how to improve the quality of your data and build data best practices into your company’s DNA.