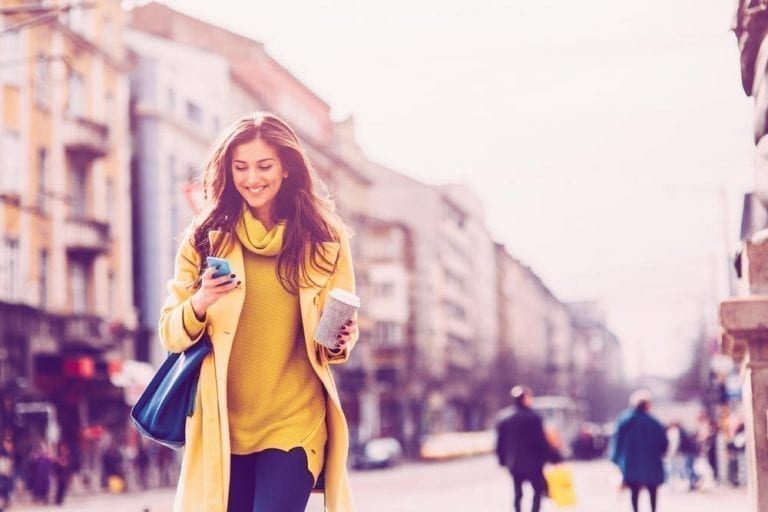
5 Data Quality Best Practices for Consistent and Error-Free Data
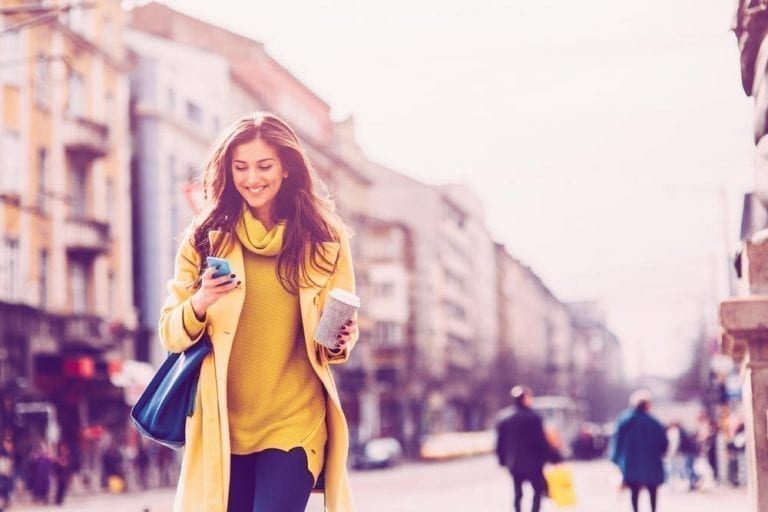
You know why data quality is important. But do you understand what data quality looks like in practice? If not, this post is for you. Keep reading for a primer on data quality best practices.
Ensuring data quality means making sure that your data sets are fit to serve the goals you intend to meet with them. Data that is inconsistent, contains errors, is incomplete or difficult to translate into the format you need is low-quality data.
If you lack data quality you may as well have no data at all. Without data quality, your data can’t reliably deliver insight into your business.
Top 5 data quality best practices
This is why you should adhere to the following five best practices for maximizing data quality:
1. Establish metrics
In order to track data quality and assess your organization’s ability to improve the quality of its data over time, you need clear metrics for measuring data quality.
Data quality metrics can include information like the number of incomplete or redundant entries in a database, or the amount of data you have that cannot be analyzed due to formatting incompatibilities.
The exact data metrics you use may vary. What’s essential is to have firm metrics of some kind in place for assessing data quality.
2. Perform data quality post-mortems
From time to time, something will go wrong due to poor data quality. You may be unable to import data into Hadoop because of formatting problems, for example. Or you may deliver marketing materials to the totally wrong people due to data quality errors.
You should make it a recurring practice to perform a post-mortem after such a problem occurs. Don’t just deal with the consequences and hope they don’t recur, because they will if you fail to take steps to understand and address the underlying cause of the issue.
Read the eBook
4 Keys to Improving Data Quality
This eBook will guide and inform you as to how to overcome the root problems of data quality and clarifying the roles of data quality management and data governance.
3. Educate your organization
Today, it’s hard to find an employee who doesn’t have a role to play in data management – whether he or she realizes it or not.
True, not everyone is a data scientist. But almost everyone works with data in one way or another…
- Administrative assistants enter manual data in an appointment book.
- IT personnel make decisions about which machine data logs to keep, and where to store them.
- Marketers design websites that automatically collect data about customers.
All of these people – and, indeed, everyone in your organization – should be educated in the basics of data quality. They should understand the importance of avoiding data errors, inconsistencies, and incompleteness.
Following data quality best practices will help you keep consistent, error-free data that meets its intended goals.
4. Establish consistent procedures
Speaking of consistency, making your data input, storage, extraction and analytics processes as consistent as possible is key to ensuring that your data itself also remains consistent.
Consistent procedures are based on clearly documented steps that everyone follows. Creating and enforcing procedural rules for handling data will do much to help avoid common data quality problems.
5. Perform data quality assurance audits
You don’t want to wait until you need your data to find out it has quality problems. Instead, you should perform routine and recurring audits.
An audit doesn’t have to involve manual work. You can make data audits a routine, ongoing process by taking advantage of automated data quality solutions, like those from Precisely.
Read our eBook 4 Keys to Improving Data Quality which will guide and inform you as to how to overcome the root problems of data quality and clarifying the roles of data quality management and data governance.