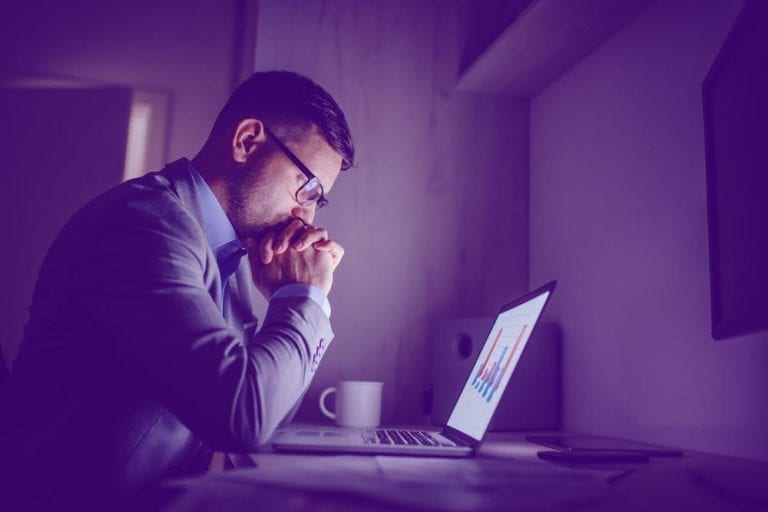
How to Clean Up Your Data for Anti-Money Laundering (AML) Compliance
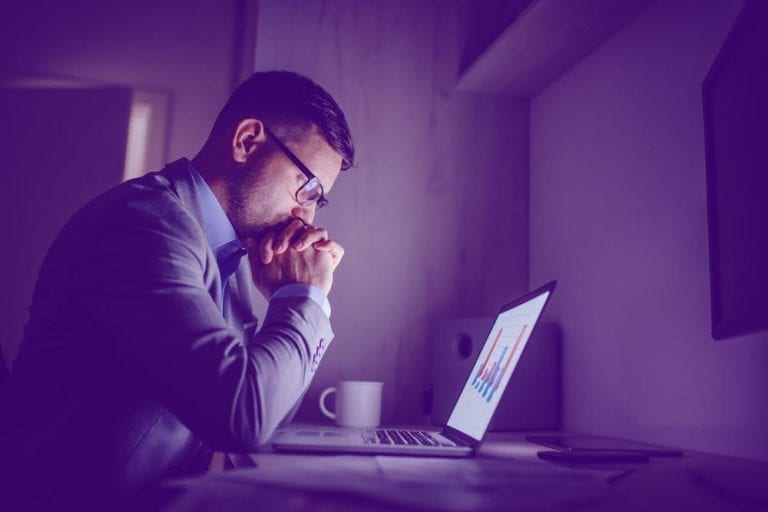
How big a problem is money laundering? Experts estimate that every year, criminal enterprises launder between $500 billion and $1 trillion through legitimate businesses. Any economic impact that large sends repercussions throughout the global economy, which is why anti-money laundering compliance is essential.
Firms throughout financial services are required to store data at scale and carefully track it for instances of money laundering. It’s a classic needle in the haystack scenario too large for even teams of auditors and compliance officers to take on. Many are turning to machine learning as an alternative to manual workloads and a long-term solution to anti-money laundering compliance.
Machine learning has an unparalleled ability to synthesize information, locate patterns, and identify anomalies. It can quickly and clearly distinguish suspicious transactions from millions of legitimate ones. Smart tech empowers firms to prevent money laundering, preserve compliance, and avoid expensive fines or disruptive investigations.
Data quality process for anti-money laundering compliance
As advantageous as machine learning may be, implementing the technology isn’t easy. There is an adage cautioning that 80% of machine learning is preparing the data. And if it’s not prepared, machines can’t learn anything. Therefore, anti-money laundering (AML) compliance depends entirely on cleaning up the relevant data first. Follow these steps:
1. Integrate all data assets
Data that is subject to AML laws streams in from multiple sources – mainframes, POS systems, ATM machines – throughout the day. It must be integrated into one place with a common file format for machine learning to be able to search it thoroughly.
2. Cleanse data at scale
Eliminating errors, omissions, and inconsistencies from data is possible selectively but difficult to do at scale. Firms need to be able to expand their data cleaning efforts exponentially or else machines will end up learning from untrustworthy data.
3. Resolve entities and identify customers
Money laundering involves a lot of moving parts which only become more obscure when they’re buried in mountains of diverse data. In order to track the perpetrators, firms have to be able to follow suspects through the digital paper trail even if their name, address, or other distinguishing information changes.
Read our White Paper
Global AML and KYC Compliance
This white paper explores how investigators are able to achieve efficiency and focus through consolidated alerts and by utilizing a visual interactive interface to review alerts.
4. Update data in real time
Compliance is an ongoing effort, not an occasional one. Firms need access to real-time data to locate the red flags of money laundering as soon as they appear. Once data is automatically updated, AML compliance becomes proactive instead of reactive.
5. Establish lineage to the source
By the time data is ready for machine learning analysis it has traveled across the enterprise through multiple clusters. Establishing the key connections in a money laundering case requires there to be a clear data lineage from beginning to end.
Preparing for machine learning can appear as challenging as ending money laundering itself. Precisely offers a complete toolkit for streamlining and standardizing data in advance of a machine-learning initiative. Don’t let your solutions lead to setbacks. Instead, lay the foundation for a future when your firm stands up to money launderers and lowers compliance costs at the same time.
Read our white paper Global AML and KYC Compliance, which explores how investigators are able to achieve efficiency and focus through consolidated alerts and by utilizing a visual interactive interface to review alerts.