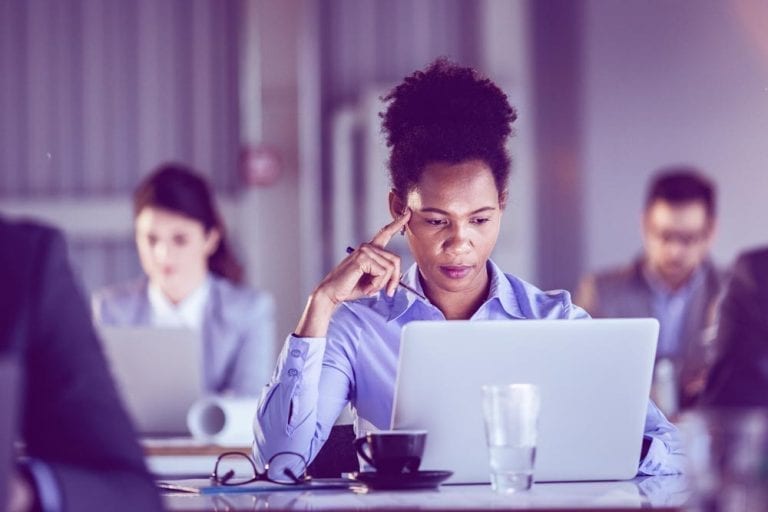
Addressing 5 Real-World Issues Impacting Data Quality
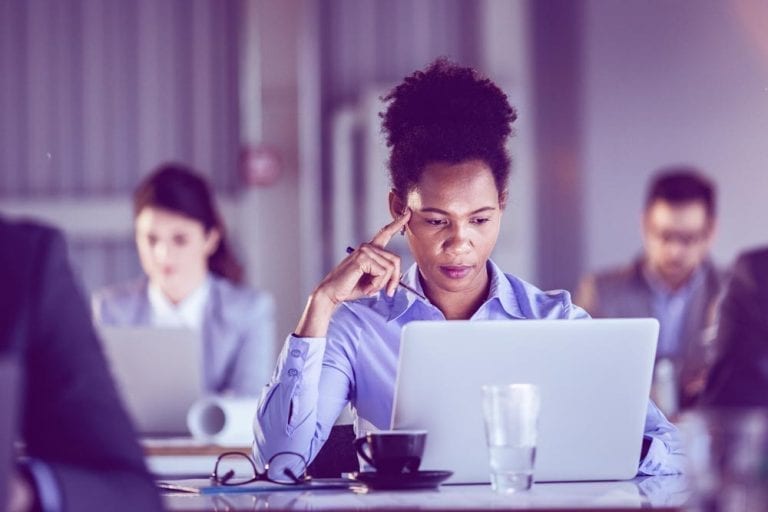
Data is the most important resource your company owns. When your data quality is good, it’s a tremendous asset. But when your data quality is bad, it’s a serious liability.
Anytime you’re making crucial business decisions using data-driven insights, you need to trust the underlying facts and figures. If they’re incorrect or incomplete, they could be skewing your perspective and leading you towards a disastrous decision.
The worst thing about poor data quality is that you often don’t notice the problem until you feel the consequences. These issues sit unsuspectingly in databases, raising no red flags until it’s too late. You can’t hope to avoid them. The only option is to eliminate the current bad data and prevent any future bad data.
Step one is learning what kinds of issues have the most significant impact on data quality. Once you understand the problem, you can target your solution instead of trying to clean up every piece of data you own.
Duplicate data
Redundant data doesn’t just waste space inside databases; it can also throw off calculations and lead to wildly misinformed insights. Data can be duplicated by human or machine error, but in all cases, it’s because of a bad integration effort. An automated system can identify and remove duplicate data while automatically combining data from across sources.
Incomplete data
A bad integration can also cause data to get deleted or lost. The missing data robs everything around it of context, rendering large data sets untrustworthy and unusable. Once again, a better approach to integration is the solution. Instead of doing things manually or relying on inflexible tools, integrate data using a tool that ensures it’s complete.
Read our eBook
4 Ways to Measure Data Quality
Assessing data quality on an ongoing basis is necessary to know how well the organization is doing at maximizing data quality. There are a variety of data and metrics that organizations can use to measure data quality. Learn how you can measure data quality and track the effectiveness of your data quality improvement efforts.
Inconsistent data
Data stored in multiple formats may not be understandable to every system trying to access it. The problem may be inconsistent file types or even just mismanaged file-naming conventions. The consequence is that whole categories of data are segregated from users. Standardizing data manually is a huge undertaking. Thankfully, better integration technology can do much of the work automatically.
Inaccessible data
When there is a disconnect between mainframe data and big data platforms like Hadoop, it leaves mission-critical data out of analytics. Inconsistent data collection and storage policies can have the same effect. Creating a link to the mainframe and instituting enterprise-wide data policies makes information broadly accessible.
Unsafe data
The more accessible data is, the less secure it becomes. Companies may be able to extract value from it, but at the same time, they are putting themselves at risk. Data integration and analytics efforts can’t make data vulnerable. Emphasizing cybersecurity during the integration effort prevents data lakes from becoming prime targets.
Bad data quality is a direct drain on companies. According to a Gartner survey, bad data cost each organization an average of $14.2 million annually as long ago as 2013. More importantly, the costs will continue to grow as companies become ever more data-dependent.
Instead of making do, start addressing the issues directly: Explore the big data solutions available from Precisely. Also, make sure to check out our eBook on 4 ways to measure data quality.