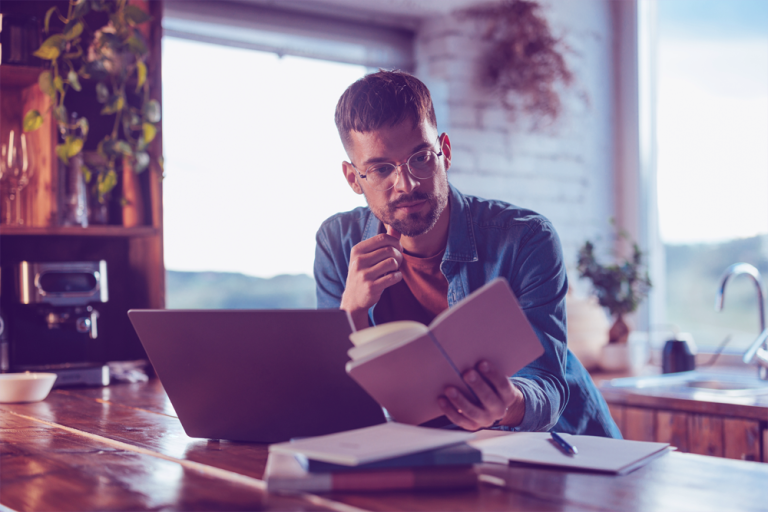
5 Best Practices for Data Observability Success
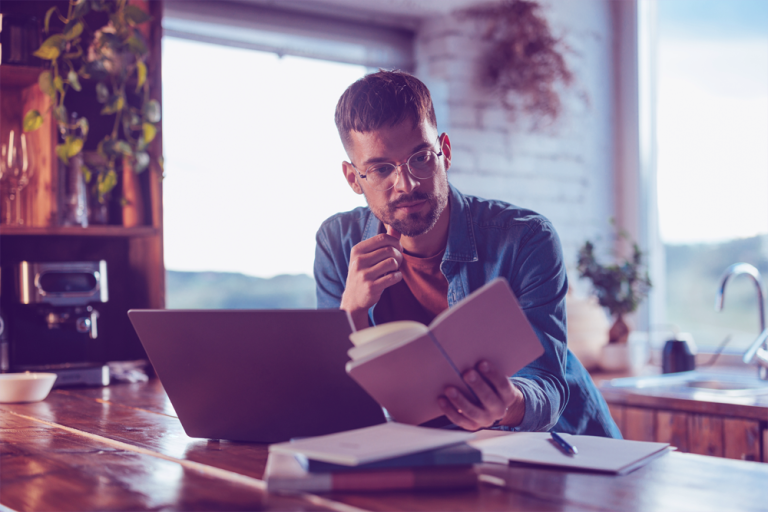
Working backwards to repair data issues can get very expensive, very fast – that’s nothing new. But what if you could take control and catch those anomalies in real time, fix them faster, and cut costs? Data observability makes it possible.
Data observability improves your trust in data by monitoring its health and proactively flagging issues to you, before they spiral into bigger problems for your business. A recent TDWI Checklist Report by James Kobielus identified how to succeed with data observability tools. It comes down to five best practices:
- Define core data quality and pipeline metrics
- Consolidate data observability silos across domains
- Unify monitoring of enterprise data and its pipeline
- Deliver actionable observability to stakeholders
- Scale and automate data observability
With consistent monitoring of data quality, reliability, and performance, you’ll be well on your way to a clearer understanding of data health, less data downtime, and better decisions.
Why data observability is essential to your data strategy
At a high level, data observability impacts key areas of your data strategy by enabling you to easily visualize your data health. And by proactively identifying and alerting you to anomalies, you’re able to fix problems faster and avoid costly downstream issues.
While you can read full details in the TDWI Checklist Report, we’ve provided a snapshot of their five best practices for succeeding with data observability tools below.
1. Define core data quality and pipeline metrics
What it means:
Asking questions that establish the core metrics that matter the most to your business. Quality metrics include data creation and movement, data synchronization, and changes to data’s schema, distribution, relationships, and metadata.
Pipeline metrics can include its capacity to handle expected workloads, frequency of unplanned downtime in each segment of the pipeline, and how well the pipeline consistently and proactively detects and resolves harmful issues.
Why it matters:
Metrics like these provide actionable insights for team members who are responsible for data across the business. Comprehensive visibility makes it easier for stakeholders to continuously track and optimize the health of data as it moves through pipelines.
2. Consolidate data observability silos across domains
What it means:
Establishing standardized observability rules across all data domains. “Enterprises should consolidate the collection of metadata on pipeline workloads, performance bottlenecks, availability status, and processing delays,” writes Kobielus.
Why it matters:
In short, siloed metadata for pipelines and data sets won’t provide you with the big picture you need of data health. With greater visibility into issues, you can trace errors back to their roots, and ultimately become more proactive in tackling them head-on.
Report
TDWI Checklist Report: Data Observability
This TDWI report for Data Observability examines key benefits and features of data observability and why organizations should make it part of their data management practice.
3. Unify monitoring of enterprise data and its pipeline
What it means:
The proactive monitoring of pipelines for data quality issues and anomalies. With triggered remediation rules in place, you’re able to address problems faster – before they negatively impact your business.
Why it matters:
With a holistic view of the organization’s data, quality data observability tools provide real-time alerts when systems break, and reduce false positives by measuring not just individual metrics – but how they may impact the system at large.
That means less time spent tracking those false positives, and greater cost savings on fixes for data issues.
4. Deliver actionable observability to stakeholders
What it means:
Granting a level of data observability to teams throughout your business. After all, responsibility for data is dispersed throughout an organization – but different teams have different needs. All parties involved need to understand how data’s being processed in the pipeline.
Why it matters:
Data observability has countless use cases, from IT to data teams – including, engineers, scientists, analysts – to executive leadership looking for greater trust in data, less risk, and a cost-effective investment that aligns data delivery with their desired business outcomes.
5. Scale and automate data observability
What it means:
Leaving behind the inefficient, old school IT system management approaches to data pipeline management. Instead, we need to embrace cloud-based, machine learning (ML)-powered data observability tools that automate core pipeline functions.
Why it matters:
Powerful automation streamlines critical processes and saves teams a lot of headaches. Validating data as it’s ingested, tracing the cause of anomalies back to the source, and preventing unplanned data downtime are just a few of the many benefits to be found with this modernized approach.
And when the issue remediation itself can’t be fully automated, ML helps to guide data professionals as they work to repair the issues more effectively.
Scale up your pipelines, scale down the effort with data observability
So why is now the time to automate with a modern, ML-powered data observability solution?
Kobielus sums it up: “In order to keep pace with data volumes and workflows, enterprises must automate the data observability process to the maximum extent possible … to scale up their data pipelines while scaling down the human effort needed to monitor and manage them around the clock.”
The results? Data-driven decisions that unlock new possibilities for your business.
To learn more, read the full report: TDWI Data Observability Checklist.