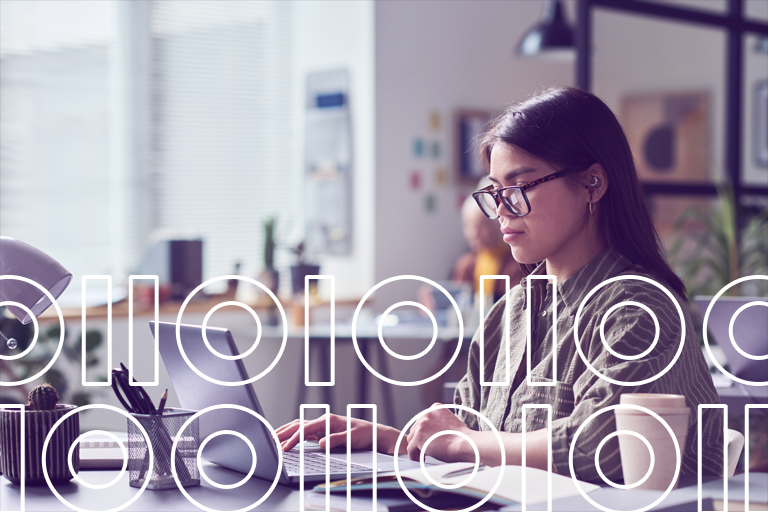
How to Power Successful AI Projects with Trusted Data
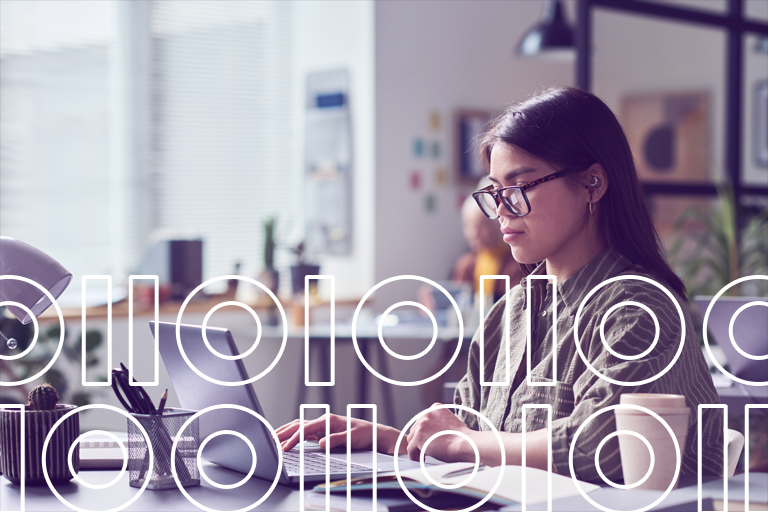
Key Takeaways:
- Trusted AI requires data integrity. For AI-ready data, focus on comprehensive data integration, data quality and governance, and data enrichment.
- A structured, business-first approach to AI is essential. Start with clear business use cases and ensure collaboration between business and IT teams for the greatest impact.
- Building data literacy across your organization empowers teams to make better use of AI tools.
It doesn’t seem like long ago that we thought of artificial intelligence (AI) as a futuristic concept—but today, it’s here in full swing, and organizations across sectors are working to integrate it into their core processes.
However, achieving success in AI projects isn’t just about deploying advanced algorithms or machine learning models. The real challenge lies in ensuring that the data powering your projects is AI-ready. Above all, you must remember that trusted AI starts with trusted data. And trusted data requires data integrity: data that’s accurate, consistent, and contextualized.
In a recent EDM Council webinar, industry experts Antonio Cotroneo (Director, Product Marketing, Precisely) and Sanjeev Mohan (Principal, SanjMo) discussed the critical role of AI-ready data in successfully moving AI projects from experimentation to production. Watch the entire session AI-Ready Data: Transforming Projects into Production to hear all their thoughts – and read on below for a preview of the top insights.
Three Top Challenges to Achieving AI-Ready Data
Whatever your unique use cases, high-integrity data is crucial for taking your AI initiatives beyond the lab and into full-scale production. Many organizations are eager to explore AI’s potential, but most face significant hurdles in moving their projects from the experimental stage to real-world applications.
Without proper data preparation, you risk issues like bias and hallucination, inaccurate predictions, poor model performance, and more. “If you do not have AI-ready data, then you’re more than likely to experience some of these challenges,” says Cotroneo.
Here are three top challenges organizations face when preparing their data for AI and their impact on the results.
1. Data integration struggles = biased outcomes
Ensuring comprehensive data integration across your organization is often the first stumbling block. If your business operates with fragmented data across silos, then your AI models are working with incomplete or inconsistent datasets.
The impact? This lack of access to critical relevant data can lead to your AI models producing skewed or irrelevant results, which can lead to poor decision-making.
2. Data quality and governance gaps = inaccurate results
A lack of data governance and quality can lead to inaccuracies, hallucinations, and AI failures. AI systems require high-quality, well-governed data to avoid missteps. Without this, you risk AI models producing incorrect or harmful outcomes – like the exposure of private internal data.
Mohan stressed, “The reason data governance has been so problematic in the past is that it’s always been an afterthought. Make sure that your AI initiative has governance built in right from the get-go.”
3. Data enrichment gaps = irrelevant responses
Context is everything in AI. If you’re not enriching your internal data with trusted third-party datasets filled with rich contextual information, AI models are left with an incomplete understanding of the task at hand.
This lack of context can result in irrelevant outputs that don’t reflect the user’s nuanced needs. As Cotroneo explained, “Your data is the difference between having something generic and something that is properly crafted for your audience.”
Tackling these challenges head-on is critical to ensuring AI projects are built on a solid foundation. Next, we’ll cover some key considerations for ensuring AI data readiness.
eBook
Trusted AI 101: Tips for Getting Your Data AI-Ready
Future-proof your AI today with data integrity. It’s time to maximize the potential of your artificial intelligence (AI) initiatives. Get inspired by valuable AI use cases, and find out how to overcome bias, inaccurate results, and other top challenges. Technology-driven insights and capabilities depend on trusted data.
Practical Steps for Ensuring Data Readiness for AI
AI success requires a structured approach to data readiness. Here are some practical steps and best practices to guide your organization:
1. Identify business use cases
This is your first and most essential step. What business problem(s) are you solving with AI? Successful implementation starts with gaining a clear understanding of your business needs.
You also need to keep in mind that AI should be treated as a business initiative, not just a technological project. This requires collaboration between your business and IT teams, who can use their shared knowledge to identify the use cases that offer the most significant impact. As Mohan noted, “Business and IT need to work together because business has a good understanding of the use cases and pain points, and IT has the understanding of … the AI capabilities.”
It’s also important to note that the costs associated with AI are significantly higher than traditional data and analytics, making it essential to identify the most valuable use cases before proceeding with implementation.
2. Assess the current state of your data
With your use cases in mind, you then need to assess your data’s completeness, quality, and governance. Ask yourself questions like:
- Does our data have proper governance and quality controls?
- Is it contextualized with necessary third-party data?
- Are there data silos that could hinder our AI results?
It is crucial to know where all this data is and ensure that the people who need it have access to it and that it has integrity.
3. Make sure data elements are defined consistently
Consistency across data sources is equally important – and that’s something a robust data catalog can help you manage. A data catalog serves as a common business glossary. Without it, your organization risks crumbling under conflicting data definitions before even beginning your AI projects.
4. Build data literacy across your organization
Building data literacy throughout your organization ensures that all teams – from data engineers to business leaders – are aligned and capable of effectively using AI tools. Everyone must understand how AI works, the type of data it requires, and how to ensure high-quality inputs to achieve the desired outputs.
As Cotroneo puts it, when “everyone in the organization [has] some level of data literacy, that’s going to help them leverage these tools to the best of their ability.”
Real-World Examples of AI-Ready Data Implementation
Want an extra boost of inspiration for your journey? Here are just a few examples of how organizations are finding AI success by prioritizing data readiness:
Healthcare applications
In healthcare, where decisions can often be life-or-death, organizations have recognized the need for robust data management practices. By focusing on data integrity, they’re using AI to streamline clinical trials, reduce drug approval times, and make data-driven decisions that can save lives.
Mohan explained that with properly prepared data, these institutions “take AI to the next level.” AI can be used to answer critical questions for these organizations, like “… are there any hidden diagnoses for diseases? Are there opportunities for me to reduce the time it takes to get FDA approval for a new drug? Because a new drug can take ten years and billions of dollars to make it to market. The stakes are very high. So, AI can be a game changer in some very advanced use cases.”
Generali Real Estate
Generali Real Estate is leveraging AI and machine learning (ML) to empower its customers – including urban planners, real estate developers, and regional developers – to make profitable and equitable decisions about community development.
By training their models on high-quality data enriched with trusted third-party sources, Generali has taken AI projects out of the lab and into real-world applications with significant success. As Cotroneo noted, “That’s a hugely impactful story of someone taking a high-quality, high-integrity approach to their models, to their training process, to their AI development process in general. And in turn, the community is benefiting from those practices.”
Preparing for the Future: Staying Ahead in the AI Race
So, what are the final takeaways on getting your data ready for AI success?
Sanjeev Mohan reiterates the importance of having data governance in place from the beginning: “Have that structured approach: starting with a business use case, identifying potential ROI, identifying the model, doing the experimentation – and then you find out that, ‘Oh it didn’t pan out, let’s go back and take the next priority.’ So, it’s an iterative process, but it must be governed and done properly.”
Continuous learning and adaptation are also essential to staying ahead as AI evolves. When you invest in data management and prioritize data integrity throughout the journey, you’ll see the benefits in more accurate, reliable, and impactful AI outputs. As Antonio Cotroneo says, “Your data management investment is your AI investment. If you properly invest in your data management practice, you are on the path to AI-ready data.”
For more actionable insights and tips, read our eBook: Trusted AI 101: Tips for Getting Your Data AI-Ready.