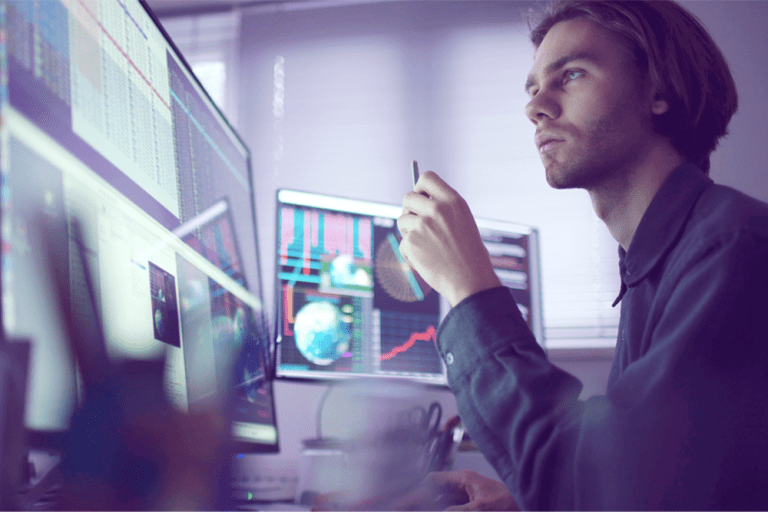
How Good Data Quality Practices Impact Your Data Integrity
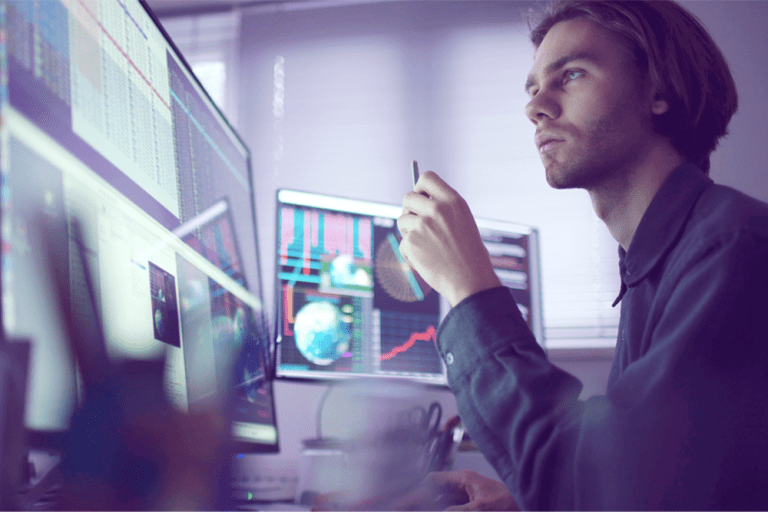
Every January, many of us resolve to make positive changes in our lives for the coming year. More often than not, those resolutions remind us of a fundamental truth about change – that to sustain positive results over the long term, you have to develop good habits and apply information relevance over time.
That rule applies to data quality as well. It’s about establishing good practices and maintaining those over the long term. It’s also important to have the right tools to help you determine information relevance and to have a solid plan of action.
Poor data quality is a big problem in most organizations. According to the analysts at Gartner, poor data quality costs the average company $12.9million each year. Addressing the data quality problem and achieving sustainable results requires an investment in time and resources. By developing the right practices and maintaining them over time, enterprises can deliver data-driven insights that engender confidence. That can be difficult to accomplish at scale, but with the right tools and a firm organizational commitment to data integrity, it is definitely achievable.
Data Quality Defined
The most general definition of data quality is “the fitness of data to serve the needs of your organization.” A more specific definition may depend on the unique requirements of each enterprise, but it generally encompasses these four dimensions:
- Accuracy: Is the information is factually correct?
- Completeness: Does the data contain gaps that prevent it from presenting a clear and meaningful picture of reality?
- Timeliness: Is the information up-to-date, and is it accessible quickly enough that it can support timely decisions?
- Accessibility: Is it accessible to the right people in a format that enables them to achieve the desired business results?
Data quality is a very important component of data integrity, but the two concepts should not be conflated with one another. Whereas data quality is focused on fitness for use, data integrity encompasses a much broader perspective. Data integrity is about building trust in data-driven decisions. It encompasses data integration, data quality & governance, location intelligence and data enrichment. Together, these ensure that your data tells a complete, accurate, contextual story about the things that matter to your business. Data integrity, therefore, involves information relevance as well.
Challenges to Achieving High Levels of Data Quality
Businesses are increasingly turning to data analytics to drive both tactical and strategic decisions. Artificial Intelligence and Machine Learning assist personnel with recommendations and automated workflows to achieve greater efficiency and rapid response to urgent situations. When data is incomplete, inaccurate, or inaccessible on a timely basis, it can severely diminish the value of a company’s investments in AI and analytics.
In a worst case situation, poor data quality can render the output of such systems invalid, leading to bad business decisions.
A sound data integrity strategy includes data quality solutions capable of standardizing and validating data, identifying gaps or discrepancies, and triggering workflows and processes to correct those errors at scale. That includes automated data discovery that catalogs and profiles data sources from across the entire enterprise. It also requires a rules-based approach to finding and fixing data quality issues promptly, and automation tools that support proactive detection of potential issues so that they can be addressed quickly.
2023 Data Integrity Trends & Insights
Results from a Survey of Data and Analytics Professionals
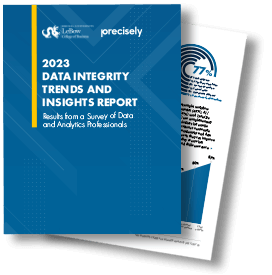
Data quality tends to decay over time. As customers relocate, as their names change, as they go out of business or pass away, the information about those customers ceases to be accurate. Over time, zip codes are re-defined, streets renamed, new area codes introduced, and boundaries redrawn. As an organization scales and the quantity of data increases, it can be a challenge to keep up with all those changes. That calls for scalable systems and processes that have been proven to work well in large enterprises.
Data quality also demands standardization and well-considered business rules that support consistency, completeness, and accuracy. Here again, enterprise-grade data quality systems are needed to support these kinds of initiatives at scale. Automation is a necessary component of this process.
Finally, we are seeing a proliferation of software systems and data sources in most modern enterprises. A few decades ago, many enterprises operated with just a few core software systems, with relatively little integration among them. Today, most companies are operating one or more ERP instances, a separate CRM system, digital marketing automation, e-commerce, and multiple other systems that often work with overlapping data.
Add to this the myriad of files stored on local hard drives and shared network folders, and the list of potential data sources can quickly grow to the point where it seems unmanageable. Automated discovery and profiling provide a foundation for reining in the chaos and starting down the road toward improved data quality.
Data Quality Serves the Higher Purpose of Data Integrity
In the larger context of data integrity, data quality and data governance ensures the accuracy, completeness, timeliness, and availability of data to support sound business decisions. To understand how data quality fits into the bigger picture, it’s helpful to take a closer look at the other three pillars of data integrity that operate in conjunction with data quality.
Integration ensures that data flows smoothly throughout the organization, eliminating data silos and making information available to people and systems that need it. As those barriers are broken down and more data is shared across multiple platforms, the data elements stored in those systems may be inconsistent. A sound integration strategy combined with the best data quality tools will ensure that your organization is working from a single version of the truth, and that information is accurate, timely, and fully available to those who need it.
Data enrichment adds context and depth to your corporate data by augmenting the information you already have with curated data from trusted sources. Data quality establishes a firm foundation of consistent, accurate data, ensuring that your company gets maximum value from its data enrichment initiatives.
Location intelligence adds further context, unlocking a vast amount of geospatial information about your customers, vendors, competitors, and more. As with data enrichment, it’s important to build your location intelligence initiatives on a foundation of internal data.
Alongside its data integration products, data enrichment, and location intelligence tools, Precisely offers a full range of data quality solutions for enterprises of all sizes, empowering people throughout your organization with relevant information that can be trusted to make data-driven decisions.
Precisely partnered with Drexel University’s LeBow College of Business to survey more than 450 data and analytics professionals worldwide about the state of their data programs. Now, we’re sharing the ground-breaking results in the 2023 Data Integrity Trends and Insights Report.