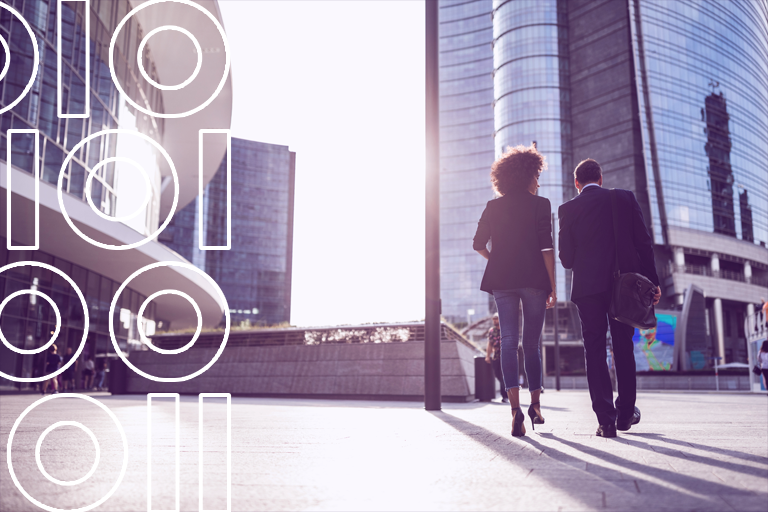
Expert Insights for Your 2025 Data, Analytics, and AI Initiatives
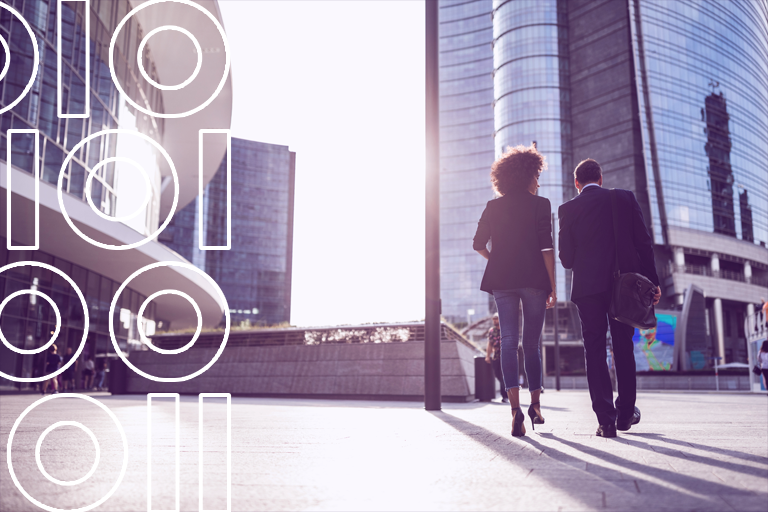
Key Takeaways:
- Data integrity is required for AI initiatives, better decision-making, and more – but data trust is on the decline.
- Data quality and data governance are the top data integrity challenges, and priorities.
- A long-term approach to your data strategy is key to success as business environments and technologies continue to evolve.
The rapid pace of technological change has made data-driven initiatives more crucial than ever within modern business strategies. But as we move into 2025, organizations are facing new challenges that are testing their data strategies, artificial intelligence (AI) readiness, and overall trust in data.
At the recent Trust ’24 Data Integrity Summit, leading data experts gathered to offer their insights into these shifts and what they mean for your business. Moderated by Josh Rogers, Precisely CEO, the panel featured:
- Tendü Yoğurtçu, PhD, CTO, Precisely
- Sanjeev Mohan, Principal, SanjMo
- Murugan Anandarajan, PhD, Senior Associate Dean, Drexel University LeBow College of Business
Their discussion was guided by findings from the 2025 Outlook: Data Integrity Trends and Insights report, published in partnership between Precisely and Drexel University’s LeBow College of Business, with panelists providing their unique perspectives into the driving forces behind these trends.
Read on for the highlights from this panel – including actionable tips to ensure success in your 2025 data, analytics, and AI initiatives.
AI Demands Data Integrity, but Data Confidence is on the Decline
First, our experts shared their insights into a top finding from the report: 67% of respondents say they don’t completely trust their organizations’ data for decision-making – marking an unfortunate increase from the 55% who expressed a lack of data trust in last year’s survey.
What are the major factors influencing this trend? The panelists shared their thoughts:
- Data ecosystem complexity is increasing. Anandarajan notes that complexity is a top factor in the decline of data confidence, citing the report finding that about 42% of organizations find their data environments becoming increasingly complex.
With the surge of new tools, platforms, and data types, managing these systems effectively is an ongoing challenge.
Yoğurtçu touched on three additional shifts that she sees as influencing the drop in data confidence:
- Data is increasingly viewed as a product. “We often hear people talking about data mesh, data fabric. Ultimately, they are trying to serve data in their marketplace and make it accessible to business and data consumers,” Yoğurtçu says. However, they require a strong data foundation to be effective.
- Cloud modernization presents challenges. Yoğurtçu also says that pervasive cloud modernization is a growing issue. With the rise of cloud-based data management, many organizations face the challenge of accessing both on-premises and cloud-based data. Without a unified, clean data structure, leveraging these diverse data sources is often problematic.
- AI drives the demand for data integrity. “Organizations are realizing that they don’t have a strong foundation and their data is not ready [for AI]. Our survey, in fact, shows 60% of participants say AI is a key influence for their data programs – however, only 12% report that their data is ready for AI initiatives,” explains Yoğurtçu. This gap underscores the urgent need for better data foundations.
AI was a recurring theme throughout the panel discussion, and our experts emphasized the crucial point that successful AI initiatives depend on a robust data strategy. “Senior leaders are realizing that there can be no successful AI project without a successful data practice,” notes Mohan.
When AI is only as trustworthy as the data it’s trained on, you must prioritize data governance, quality, and overall integrity – whether building new AI solutions or refining existing ones.
2025 Outlook: Essential Data Integrity Insights
What’s trending in trusted data and AI readiness for 2025? The results are in!
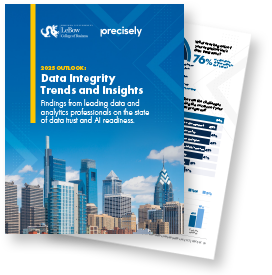
Read report
2025 Outlook: Data Integrity Trends and Insights
Download the report to see how your data program and level of AI readiness compare to your peers’, and unlock valuable insights to elevate your data strategy.
Strategic Steps for Getting AI-Ready: Upskilling and Data Strategy
Taking a deeper dive into the topic of AI readiness, the panel also highlighted the report finding that a lack of skilled professionals is one of the key barriers to AI adoption. While companies may have the right tools and governance in place, they often struggle to find the talent needed to maximize these resources.
In fact, 60% of organizations surveyed for the report cite a lack of employee skills and training as an AI adoption challenge.
In response to this insight, the panelists offered their own advice for organizations that find themselves in a similar situation.
According to Anandarajan, building a culture of data literacy is what will help to bridge this gap. “Being prepared for AI is not just about the technology, it’s about preparing your people,” he says. “You need to upskill them, you need to create AI champions who can lead, inspire, and drive change.”
Upskilling and empowering employees can help foster a culture that embraces data-driven decision-making and AI innovation.
And of course, getting your data up to the task is the other critical piece of the AI readiness puzzle. Yoğurtçu identifies three critical steps that you should take to prepare your data for AI initiatives:
- Identify all critical and relevant datasets, ensuring that those used for AI training and inference are accounted for. Without this, you run the risk of introducing harmful bias into your models.
- Plan for data quality and governance of AI models from day one. “Data quality has a multiplier impact on AI,” Yoğurtçu says. Poor-quality data can lead to faulty AI models, resulting in inaccurate or even damaging outcomes. And governance will be critical as regulations around AI continue to emerge and evolve.
- Leverage enriched data. Enriching your internal data with trusted third-party data can enhance accuracy and provide additional real-world context for your AI models, making them more reliable and less prone to hallucinations and other errors.
Overall, AI success truly depends on a business outcome-driven approach. “We need to take a step back and not start from technology … it’s not a technology initiative. AI is a business initiative,” says Mohan. “Which means that we need to understand the use case, what data we have … and then come up with the expected ROI.”
From there, Mohan says, experimentation can take place across your different use cases to determine the best fit, and identify where your data still needs improvement for the task at hand.
Data Quality and Governance are the Top Data Challenges – and Priorities
Another report finding discussed by the panel was that as organizations increasingly view data as a strategic asset, data governance and quality have emerged as the top priorities for data integrity improvement – which makes sense, given that data quality (64%) and data governance (51%) were also the top data integrity challenges, according to our survey respondents.
To help navigate these challenges, our experts offered their best practices to guide organizations focused on improving data quality and governance – and their insights are invaluable.
The panel agreed that a holistic approach to data management is key, and data governance is the place to start. They offered practical and valuable takeaways to keep in mind if you’re setting out to make improvements to your data program. Here’s a snapshot of their advice:
- Start small with clear business use cases that demonstrate ROI. Explains Yoğurtçu, “As you prove the value to internal stakeholders, then you can expand to multiple sources and add more capabilities over time.”
- Take a proactive approach. Yoğurtçu also states that you need to “ensure that trusted data is served in a timely fashion.” Data management with real-time data observability and automated health checks to ensure ongoing data integrity.
- Leverage AI to enhance governance. “Large language models are excellent at inferring hidden relationships and context,” says Anandarajan. So, you should leverage them to dynamically generate data validation rules rather than relying on static, manually set rules.
- Focus on metadata management. As Yoğurtçu points out, “metadata is critical” for driving insights in AI and advanced analytics.
Location Data is an Untapped Resource
The increasing use of location-based data was another report trend explored by our panelists. In the report, 67% of respondents say they use location intelligence, across various use cases. And spatial analytics has risen as a data integrity priority – from 13% in 2023 to 21% in 2024 – a 62% increase overall.
With regards to what may be driving this increased focus on spatial analytics, Yoğurtçu feels that organizations are now recognizing the untapped potential of spatial insights, saying, “80% of data has a location attribute, and many of the analytics were not leveraging that attribute.”
She highlights that location data is particularly valuable in industries like retail, telecommunications, and insurance, where it’s used for hyper-accurate decision-making in areas including site selection, last-mile delivery optimization, and risk assessment.
By enriching their data with location-based information, businesses can gain a competitive edge and make more informed decisions.
“Bad addresses are expensive,” adds Rogers. “The other piece though, is that if you have it right, and you have it associated with a physical location, you have this opportunity to add so much additional context and complete that data and those insights.”
Yoğurtçu emphasizes this point, and adds that context will continue to grow in importance as AI systems evolve: “Context and location insights are very important to take full advantage of and use data in a powerful way. And context also enhances the large language models. Now, we are talking about large action models – and these will require higher accuracy, enabled by additional context.”
Final Takeaways for 2025 and Beyond
As we look ahead to 2025, 76% of survey respondents say data-driven decision-making is a top goal for their data programs. But to achieve this, as highlighted throughout our expert panel discussion, you must focus on:
- starting with your business case and building a strong foundation of data integrity as part of your data strategy
- taking a long-term, disciplined approach to your data strategy – rather than only looking at the short-term. As Mohan states, “It’s time to invest in what’s next, not what’s now.”
- upskilling teams to handle both data management and AI technologies
- embracing enriched data, including spatial insights, to unlock new business opportunities
To stay ahead in this rapidly evolving space, it’s essential to focus on these key trends and recommendations. Find even more inspiration with the on-demand replay of this panel and all of the other engaging sessions from Trust ’24. And for an even deeper dive, get your copy of the full 2025 Outlook: Data Integrity Trends and Insights report today.