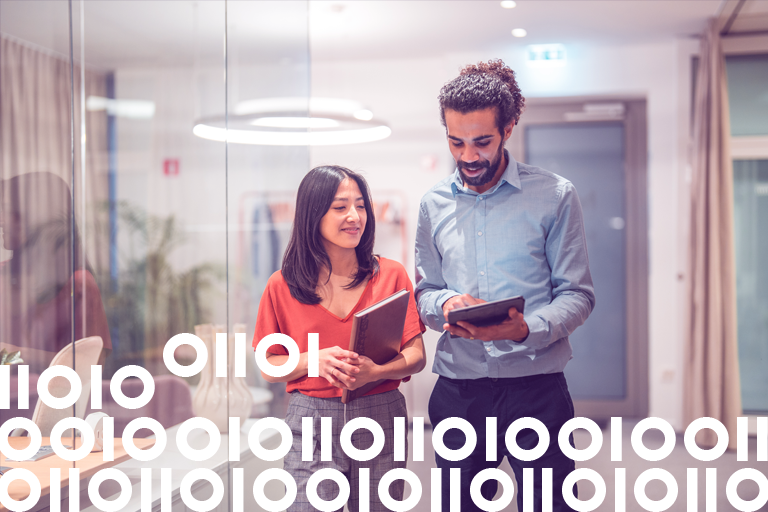
Demystifying Data Mesh
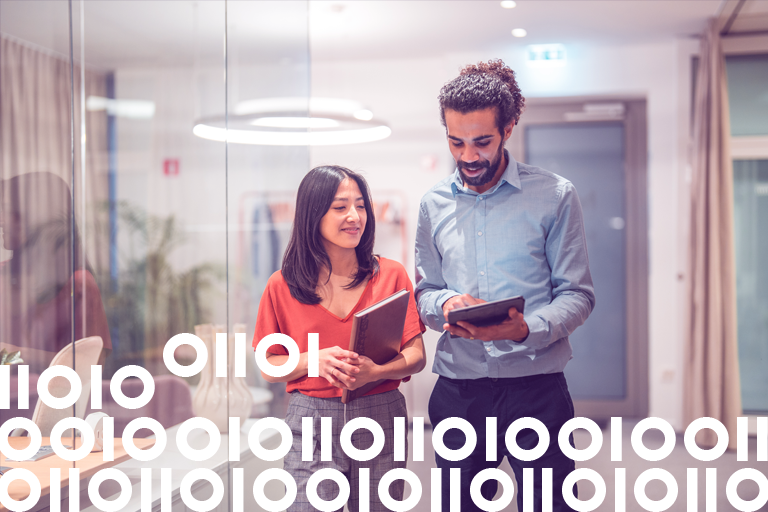
Key Takeaways
- Data Mesh is a modern data management architectural strategy that decentralizes development of trusted data products to support real-time business decisions and analytics.
- While a data mesh empowers domains with greater autonomy and innovation through human expertise, greater capabilities are needed by domain teams to properly access, organize, and govern data products.
- Preparing for a data mesh requires a change in mindset – as it is a framework, not a technology that can help you adapt to modern data management requirements.
Accurate, consistent, and contextual data leads to more confident decisions, but in a world drowning in data, organizations are struggling to manage and leverage it to produce trusted and timely data products for decision-making.
Cultivating high-quality data products to support confident decisions is a journey most organizations are on, whether they know it or not. Even without a specific architecture in mind, you’re building toward a framework that enables the right person to access the right data at the right time. However, complex architectures and data silos make that difficult.
It’s time to rethink how you manage data to democratize it and make it more accessible. Large, sophisticated organizations are exploring modern data management architectures to develop trusted data products to support real-time business decisions and analytics. One strategy being leveraged is a data mesh.
What is Data Mesh?
Data mesh is not a stand-alone solution but a modern architectural strategy that empowers your domain teams to create, organize, and maintain trusted data products so that data users can make more timely, data-driven decisions. Domain owners and subject matter experts can access critical domain data to respond quickly to data requests from their teams.
Unlike many existing approaches, with a data mesh strategy, data products aren’t consolidated into a central repository but remain in distributed data stores with technologies and processes deployed to make them more accessible and consumable.
This idea of decentralization has already gone mainstream in the software development space with cloud-native architectures and microservices – and now, some of the same concepts are breaking into the data and analytics space.
The Opportunity
In a data mesh approach, the data team responsible for a particular data domain is tasked with making data products available to data consumers upon request. This requires domain owners and subject matter experts to self-serve critical data on demand.
Data domain teams have a better understanding of the data and their unique use cases, making them better positioned to enhance the value of their data and make it available for data teams.
The data products are then strategically packaged so the data team doesn’t need to be involved when analysts and data scientists need access. With this approach, demands on each team are more manageable, and analysts can quickly get the data they need.
This contrasts with large, centralized groups that must prioritize many requests, leading to bottlenecks that may take days or weeks to respond.
The result: Greater autonomy and human expertise lead to greater innovation.
Data Mesh Challenges
While data becomes more accessible through this decentralized data strategy, the benefits do come with additional risks. Some of the most common challenges you need to be aware of include:
- Data standardization. With more autonomy and distributed data, checks and balances are more important than ever to ensure data is standardized, safe, secure, and accurate. An organizational-level set of standards and rules is required to ensure that you avoid chaos, even with data managed autonomously. Standards are still necessary at the domain level, and conflicting rules can lead to disruption and poor-quality data.
- Data ownership. As your teams gain more autonomous data control, challenges around who owns what data may also emerge. Data domain overlaps exist in many cases, and each domain’s ownership and accountability may not be as clear, leading to inefficiencies and duplication of efforts.
- Data interoperability. Multiple domains often need to share data assets. Quality and formatting may differ with more autonomous domain teams producing data assets, making interoperability difficult and data quality guarantees elusive.
- Data discoverability and reusability. The move to productize data also requires a way to package data products so they are easily and uniformly discoverable and consumed. Data products must be properly designed and organized to be reused across the organization.
2025 Outlook: Essential Data Integrity Insights
What’s trending in trusted data and AI readiness for 2025? The results are in!
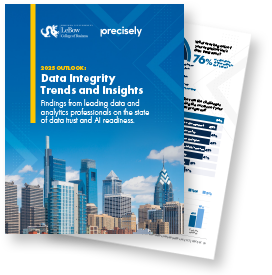
Preparing for a Data Mesh Strategy
Key pillars when preparing for a data mesh strategy include:
- A mature data governance strategy to manage and organize a decentralized data system.
Proper governance ensures that data is uniformly accessible and the appropriate security measures are met. With the responsibility to build data products at the domain level, data governance must be federated or implemented at the domain level for greater autonomy, yet coordinated with a centralized data governance strategy.
- Self-service access to critical data on-demand for decentralized data teams – enabling them to be more agile in responding to data requests from their team. Data observability tools are another critical piece of the technology stack required to ensure that data pipelines operate as intended and can troubleshoot errors in data products.
- A channel or infrastructure that enables users to access data products easily and allows data domains to communicate effectively. A standard publishing and discovery platform is needed to ensure data consumers know these products are available. Data catalogs or data marketplaces can be used to enhance the value of your data mesh strategy.
- Metadata that’s accurate, comprehensive, up-to-date, understandable, accessible, and shareable so data products are more discoverable. Standardizing naming conventions also makes data products interoperable. DataOps tools and strategies help discrete domains share repeatable workflows, reducing the duplication of efforts and improving efficiency.
Looking Ahead: A Data Mesh Mindset
As your organization explores the possibilities of a data mesh strategy, it’s important to adjust your mindset and think about it as a framework, not a technology.
New architectures will be required to enable your strategy as the process shifts. Investments in solutions that deliver data integrity – accurate, consistent, and contextual data – will play a pivotal role in realizing this cultural shift. These tools will help you continuously enhance the process, but it begins with a change in perspective and a cultural transformation regarding data ownership and agility to transform data into valuable data products.
Does that align with your business goals? Then you need to know more about data mesh architecture.
Precisely the global leader in data integrity, in partnership with the Center for Applied AI and Business Analytics at Drexel University’s LeBow College of Business, surveyed 565 data and analytics professionals on their organizations’ data strategies, priorities, challenges and the state of data trust and AI readiness. The results are in, read the report today! 2025 Outlook: Essential Data Integrity Insights