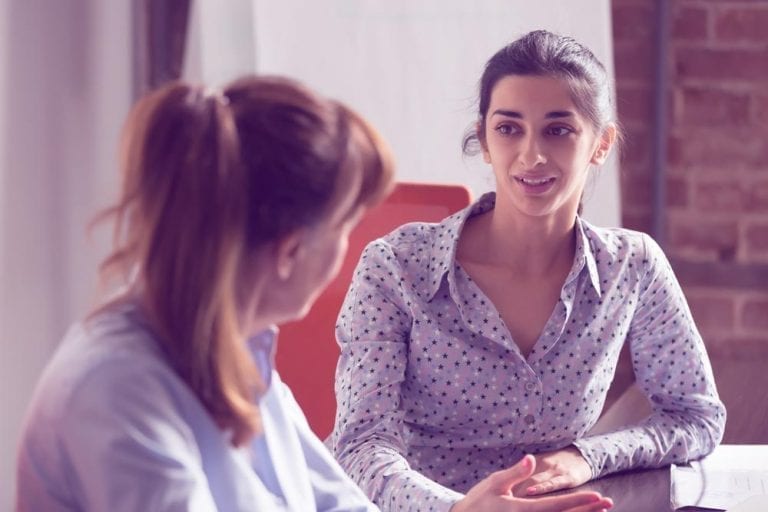
Data Integrity vs. Data Quality: How Are They Different?
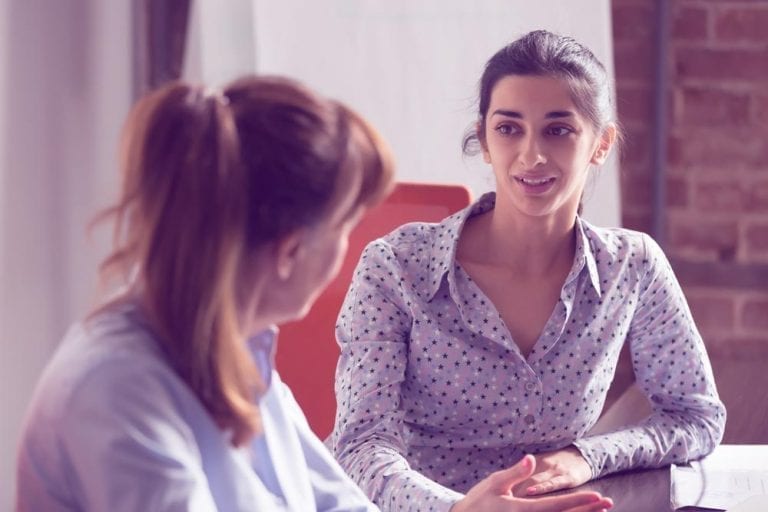
Data can be your organization’s most valuable asset, but only if it’s data you can trust. When companies work with data that is untrustworthy for any reason, it can result in incorrect insights, skewed analysis, and reckless recommendations to become data integrity vs data quality.
Two terms can be used to describe the condition of data: data integrity and data quality. These two terms are often used interchangeably, but there are important distinctions. Any company working to maximize the accuracy, consistency, and context of their data to make better decisions for their business needs to understand the difference.
Defining data quality
Data quality refers to the reliability of data. Data quality is an essential subset of data integrity. (Related: What is Data Quality?)
If data is to be considered as having quality, it must be:
- Complete: The data present is a large percentage of the total amount of data needed.
- Unique: Unique datasets are free of redundant or extraneous entries.
- Valid: Data conforms to the syntax and structure defined by the business requirements.
- Timely: Data is sufficiently up to date for its intended use.
- Consistent: Data is consistently represented in a standard way throughout the dataset.
Quality data must meet all these criteria. If it is lacking in just one way, it could compromise any data-driven initiative.
However, simply having high-quality data does not, of itself, ensure that an organization will find it useful. For instance, you may have a database of customer names and addresses that is accurate and valid, but if you do not also have supporting data that gives you context about those customers and their relationship to your company, that database is not as useful as it could be. That is where data integrity comes into play.
2025 Outlook: Essential Data Integrity Insights
What’s trending in trusted data and AI readiness for 2025? The results are in!
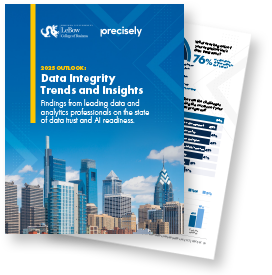
Defining data integrity
While data quality refers to whether data is reliable and accurate, data integrity goes beyond data quality. Data integrity requires that data be complete, accurate, consistent, and in context. Data integrity is what makes the data actually useful to its owner. (Related: What is Data Integrity?)
Obviously, data quality is a component of data integrity, but it is not the only component. Data integrity is based on six main pillars:
- Data integration: Regardless of its original source, on legacy systems, relational databases, or cloud data warehouses, data must be seamlessly integrated in order to gain visibility into all your data in a timely fashion.
- Data observability: Prevent business disruption and costly downstream data and analytics issues using intelligent technology that proactively alerts you to data anomalies and outliers.
- Data quality: Data must be complete, unique, valid, timely, and consistent in order to be useful for decision making.
- Data governance: Manage data policy and processes with greater insight into your data’s meaning, lineage, and impact.
- Location intelligence: Make data more actionable by adding a layer of richness and complexity to it with location insight and analytics.
- Data enrichment: Add context, nuance, and meaning to internal data by enriching it with data from external sources. Adding business, consumer, or location information gives you a more complete and contextualized view of your data for more powerful analysis.
The bottom line
Data is a strategic corporate asset, and both data quality and data integrity are essential for organizations looking to make data-driven decisions. Data quality is a good starting point, but data integrity elevates data’s level of usefulness to an organization and ultimately drives better business decisions.
To begin your journey to data integrity, you may first need to address issues of data quality. Companies that make a proactive effort to fix data quality issues and prevent future ones see better outcomes from all their data-driven initiatives with a deeper look at data integrity vs data quality.
The Precisely Data Integrity Suite is the first fully modular solution that dramatically improves a customer’s ability to deliver accurate, consistent and contextualized data. It spans the full spectrum of data integrity, with accuracy and consistency drawn from best-in-class data quality and data integration, and the critical element of context from market-leading location intelligence and data enrichment.
FAQs for Data Integrity vs. Data Quality
Can you have good data quality and not have data integrity?
Yes, it’s certainly possible to have good data quality without data integrity. The two terms are sometimes used interchangeably, but they have different meanings.
Data quality refers to the reliability of data based on its completeness, uniqueness, validity, timeliness, and consistency. That means having large enough datasets to accurately represent the information in question, including information on all relevant fields. They should be free from redundancy without containing duplicate records, conform to the syntax and structure defined by business requirements, and be up to date with respect to their intended use. Finally, they should be consistent, meeting a common set of standards across entire datasets.
As defined by Precisely, data integrity goes much further than data quality. Data quality is an essential subset of data integrity, but it is possible to have good data quality without also having data integrity. For example, a company that lacks data integration will suffer from data silos, which undermines an organization’s ability to put that data to practical use. If information lacks context, it may also suffer from poor data integrity. Data enrichment with third-party data and geospatial insights improve integrity by adding context to existing datasets, improving the overall trustworthiness and usefulness of the information.
How does data integrity impact business outcomes?
Data with high integrity is better suited to deliver business value because it provides a more holistic understanding of reality. For example, if product managers analyze customer behavior based solely on information from a mainframe transaction processing system, they may be missing the bigger picture that effective data integration could provide. By combining that information with customer data residing in digital marketing automation software, details maintained in a product information management (PIM) system, and other data from across the organization, business users can get a much better understanding of the data that matters most to them. The same scenario benefits from data enrichment as well.
By joining demographic data with internal customer information, the company can develop a much richer understanding of who its customers are. Location intelligence brings yet another layer of contextual richness by opening up thousands of new data points that shed light on customers’ lifestyles and interactions with your company. Effective data governance completes the picture, ensuring that information is safeguarded against unauthorized access, that it has high data integrity, and that the organization remains in compliance with legal and regulatory requirements. In short, any situation that calls for analytics will also benefit from increased levels of data integrity, enabling users to drive better business outcomes.
What should you do if your data lacks integrity?
Every organization’s journey to data integrity differs, but each path includes quality data. That means proactively fixing fundamental data quality issues and implementing systems to prevent new data quality problems from cropping up. To achieve this at scale, companies need enterprise-grade tools that apply business rules to define and enforce data quality, route potential problems to the individuals best suited to address them, and monitor KPIs for the organization.
Data quality is a single step, though. To build trust in your data and ultimately drive better business outcomes, develop a long-term vision for your organization and its use of data as a strategic asset. That means understanding how your team will use the data and how data integration, enrichment, and location intelligence can enhance its overall value.
Which approach works best for businesses looking to improve the integrity of their data?
A modular approach allows businesses to tailor a data integrity strategy to their unique needs. For example, companies may wish to select a data quality solution that best fits their business, adding data governance, data integration, enrichment, and location intelligence in a way that best suits their plans for delivering data-driven value. Every organization has unique requirements, and a modular approach offers flexibility in designing a complete solution and rollout plan that meets those needs. For example, insurance carriers specializing in property and casualty coverage can benefit significantly from location intelligence tools to help assess risk, detect potential fraud, and uncover new market opportunities. Consumer products companies might prefer to focus on data enrichment to understand their target audience better and reach the right consumers with the right messages.
A modular toolset for data integrity ensures interoperability without requiring custom integration or inconvenient workarounds. The Precisely Data Integrity Suite contains everything you need to deliver accurate, consistent, contextual data to your business – wherever and whenever it’s needed.
What tools does Precisely offer to improve data integrity?
The Precisely Data Integrity Suite is a set of interoperable services that enable your business to build trust in its data. Data with integrity has maximum accuracy, consistency, and context – empowering fast, confident decisions that help you add, grow, and retain customers, move quickly, reduce costs, and manage risk and compliance.
Precisely the global leader in data integrity in partnership with the Center for Applied AI and Business Analytics at Drexel University’s LeBow College of Business, surveyed 565 data and analytics professionals on their organizations’ data strategies, priorities, challenges and the state of AI readiness. The results are in – read our report today – 2025 Outlook: Essential Data Integrity Insights.