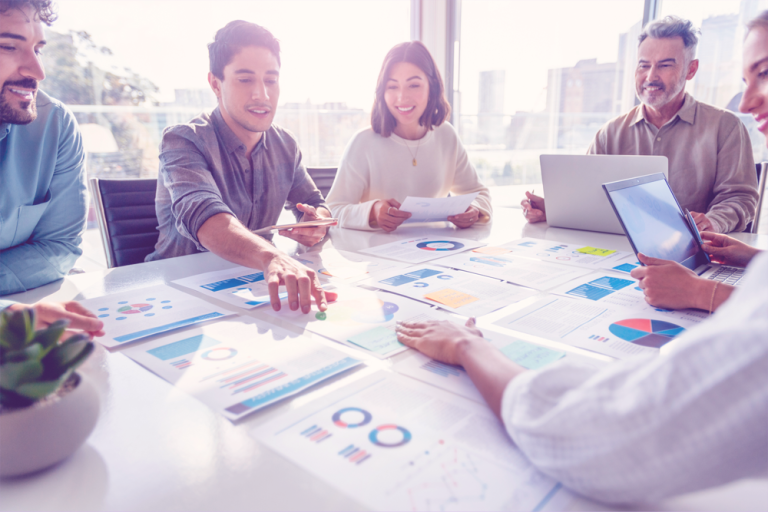
Data Integrity for ESG in Financial Services
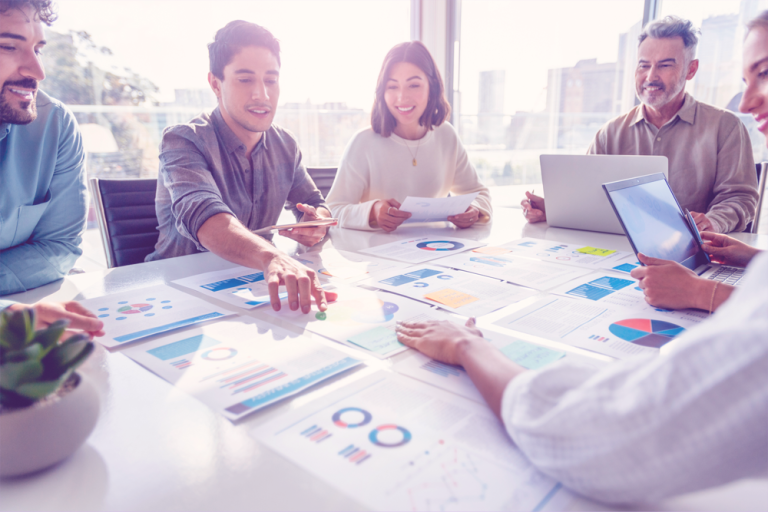
There has been a tremendous shift as employees, consumers, as well as investors are making more conscious decisions based on environmental, social and governance (ESG) factors. For many years, risk has had a significant seat at the table for a multitude of investment, lending, and other financial service organizations, but with the rise of ESG, the ramifications are reaching another level.
As more timely and accurate reporting is required to satisfy regulators and the growing interest across other influencers, it’s clear that a more accurate, consistent, and contextualized approach to report on ESG metrics is needed for the financial services industry.
Financial Services ESG Reporting: The essential role of data
Financial organizations are now assessing how they can derive more environmental, social and governance insights, while optimizing reporting through their existing infrastructure. This is also driving further scrutiny around the data integrity practices that impact their other business interests. Unfortunately, what many leaders are finding is that reporting and gathering insights from ESG data that is scattered across their complex infrastructure is a costly, and time-consuming process.
If an organization’s data lacks accuracy, consistency, and context, it will be challenging to be confident in their ESG metrics. In fact, if organizations do not already invest in the integrity of their data, they are already behind the curve, and the latest ESG regulations will only heighten that risk. To not only meet, but also exceed these requirements, there are four key steps which will improve data integrity and ensure an organization’s data infrastructure is ready for the next era.
Step 1: data integration
An organization’s infrastructure must be able to integrate data from diverse formats and disparate sources to have a comprehensive view of all ESG data. This will help them spot trends that would not have been visible otherwise and facilitate more efficient and timely reporting.
This may seem like a relatively simple concept, but it is incredibly complex. Most complex organizations have a huge number of internal functions which conduct business on multiple operating platforms – only made even more complicated with mergers & acquisitions. These organizations have data residing in all parts of their infrastructure, and perhaps even across the third parties with which they do business.
Accessing data through this chain of command and viewing it in one location is an inherent problem that flexible and automated data integration can resolve. Understanding the profile, provenance, implicit and explicit assumptions, and calculations of that data is a baseline requirement for accurate environmental, social, and governance reporting.
Solution Page
Environmental, Social, and Governance (ESG) Data Solutions
Financial Services organizations increasingly want to dive deeper and understand how the companies that they’re engaged with support ESG initiatives. See what that can mean for your organization.
Step 2: data governance and quality
The expression, ‘garbage in, garbage out,’ relating to data quality is a commonly used one — because it is completely accurate. Organizations working with bad data will receive bad results, which is why a solid data integrity plan is key. Not only does it help to bring data into one location, but it cleans it up too, bringing visibility and confidence in its quality.
Data governance is often a very manual – or at least a very siloed process, frequently led by IT programs. Instead, a board-level mandate on data is required, as well as business-led use case arguments for tools that automate the process. Not only does this save time, but it also provides real-time analytics which supports confident in-the-moment decision making. As organizations pivot to align with changing ESG initiatives, the timeliness of data governance and quality will be a crucial factor.
Step 3: location intelligence
Location intelligence unlocks essential context that the data on its own won’t otherwise reveal, connecting insights and serving as a common link between silos of data. For example, two facilities built 300 feet apart, despite the close proximity to one another, could have radically different hazard exposures, environmental impacts, and resiliency indexes. This is increasingly important for organizations to monitor as it affects how a business infrastructure, including supply chains, will operate as environmental factors such as climate change and continue to impact every part of the world.
Ultimately, location intelligence serves to elevate business decision-making in relation to people, assets, places, and opportunities. Virtually every data point in the world can be associated with location in one way or another. The breadth of information available on this topic is enormous, but getting it into a digestible, consistent, and accurate form can be very difficult.
Step 4: data enrichment
Data enrichment — the fourth pillar of data integrity — is the process of adding trusted third-party data to existing internal data to build context revealing even more insight. When accurate third-party datasets related to location, business, climate, or demographics are combined with existing business information, the whole adds up to more than the sum of its parts. This can also include dynamic datasets that track variations over time, such as weather or human mobility.
Enhanced environmental, social, and governance legislative requirements and disclosure frameworks mean that organizations are increasingly needing to report on the potential impacts of climate change on their own businesses. The addition of risk datasets related to wildfires, floods, earthquakes, and weather events, can help to reveal the history and likelihood for hazards in certain areas. This empowers businesses to make faster, more confident decisions to proactively forecast and manage risk.
Building on trusted data
Ultimately, as businesses rapidly move towards embracing ESG, they need to ensure that robust data foundations are being put in place to support the success of these initiatives. By building a meaningful strategy around data integration, data governance and quality, location intelligence, and data enrichment, organizations can be confident that they are making smarter business decisions based on data they can trust.
Financial Services organizations increasingly want to dive deeper and understand how the companies that they’re engaged with support ESG initiatives. See what that can mean for your organization, read our solution page, Environmental, Social, and Governance (ESG) Data Solutions.