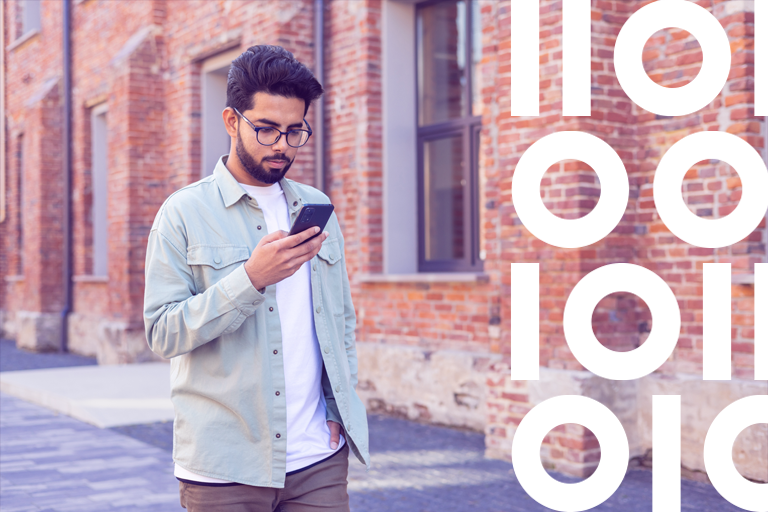
Claims Processing with Generative AI: Making Sense of the Data
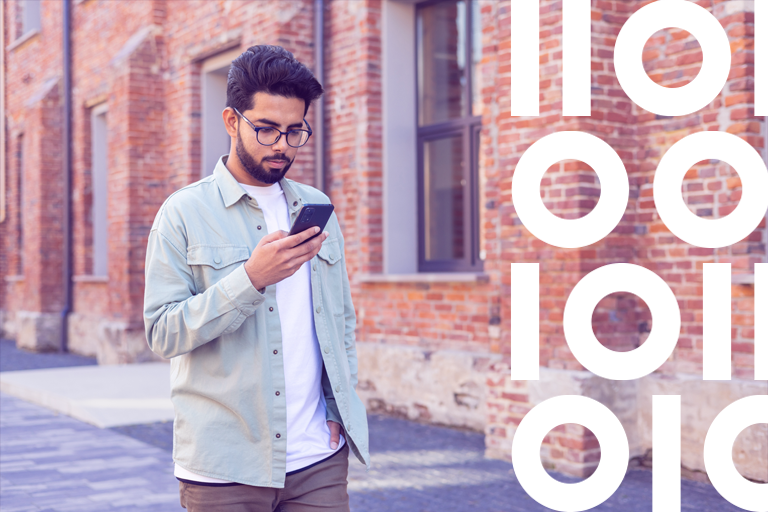
Insurance industry leaders are just beginning to understand the value that generative AI can bring to the claims management process. By harnessing the power of machine learning and natural language processing, sophisticated systems can analyze and prioritize claims with unprecedented efficiency and timeliness. They can ingest information as soon as it becomes available, summarize lengthy narrative content, and offer guidance to employees who manage the claims process.
As insurers begin to integrate these advanced technologies into their operations, the entire landscape of claims management is being reshaped, leading to faster, more customer-friendly service. Yet experts warn that without proactive attention to data quality and data governance, AI projects could face considerable roadblocks. This article focuses on some fundamental prerequisites for the successful use of generative AI in the claims management process.
How Generative AI Helps Claims Managers
Claims processing typically involves quite a few manual steps, making it both time-consuming and error-prone. From documenting losses and damages to verifying that a claim submission meets all the necessary criteria, each step requires meticulous attention to detail and often entails reviewing lengthy narrative documents such as accident reports, medical records, and legal demands letters.
As new information flows in, claims managers must constantly stay up-to-date on each claim, further adding to an already heavy workload. Generative AI can streamline many of these routine tasks, reducing the potential for errors and oversights, eliminating tedious work, and speeding up the entire process.
Automating these steps can also improve the customer experience for policyholders. For example, AI and machine learning can augment existing customer communication systems to provide highly personalized information for customers, keeping them informed throughout every stage of the claims process. AI-driven chatbots can assist customers in submitting claims, and then summarize and report the claim’s status at any time.
Narrowing the Employee Skills Gap
Generative AI helps companies narrow the experience gap and bring new employees up to speed faster than ever. Like most industries, the insurance sector is facing a labor shortage. Turnover has been relatively high in recent years, leading to an influx of newcomers with little or no experience in claims management.
AI technology can ingest and synthesize large volumes of both structured and unstructured data very quickly, offering claims guidance that helps adjusters to better assess cases. AI can flag claims that may be at high risk of litigation or other escalation, poring over hundreds of pages of medical records or legal pleadings to discover details that a human reader might miss.
Machine learning models can explore cases to detect potential cases of fraud, synthesizing data sets from a broad range of sources, and then prioritizing claims for potential investigation and follow-up. Claims managers with relatively little experience might miss these details, whereas AI technology can refine its technique over time to improve accuracy continuously.
One insurance industry expert refers to this as the “bionic co-pilot,” a powerful tool for assisting claims managers by augmenting their capacity to work effectively and freeing them to engage in meaningful conversations with customers, bringing more compassion and empathy to the interaction than ever before.
Data Quality and Data Governance
Insurance carriers cannot effectively leverage artificial intelligence without first having a clear data strategy in place. When AI models are trained with subpar or inaccurate data, the repercussions extend far beyond initial inaccuracies. Bad data has a multiplier effect when companies deploy it for AI.
AI/ML models make inferences, derive business insights, and determine outcomes, all of which are foundational to decision-making processes. If the training data is flawed, the resulting insights and decisions will be flawed as well, potentially leading to a cascade of negative effects. It’s imperative to ensure the quality and governance of the data used in training AI models. Organizations must pay meticulous attention to how they collect and manage data and proactively attend to data quality.
As many observers have pointed out, generative AI also can “hallucinate” if organizations don’t manage their use of it proactively. This risk further underscores the importance of high-quality, trustworthy data. A seemingly minor error, like a two-digit discrepancy in a policy number, has the potential to trigger a cascade of issues. Insurance carriers need to avoid those scenarios by proactively managing data quality. They also need data observability tools that allow them to trace errors back to their source and rectify the problem.
By proactively enriching internal datasets with demographic and location-based data, insurers can multiply the effectiveness of their AI/ML investments. Most insurers are already taking advantage of geospatial analytics, but the leading innovators are aggressively adding context and depth to their data with curated data from highly trusted sources.
2025 Outlook: Essential Data Integrity Insights
What’s trending in trusted data and AI readiness for 2025? The results are in!
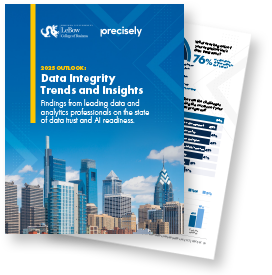
Bias in AI has also emerged as a key concern. If insurance carriers train AI models based on a limited set of demographic criteria, for example, they could end up drawing false conclusions about specific groups of people. That can lead to bad publicity, as happened several years ago in the case of Apple and Goldman Sachs. Governments are increasing their attention to these issues. Business leaders will need to proactively address issues like bias in the face of emerging regulations.
Generative AI promises to have a transformative effect on insurance claims management, but the successful deployment of this technology hinges on two critical factors: people and data. Training, enabling, and empowering employees to work alongside AI is essential. By augmenting their work with AI, employees can leverage their expertise while benefiting from AI’s efficiency and scalability, leading to enhanced productivity and innovation.
At the same time, insurance carriers must attend to data integrity and data governance. Data is a key differentiator, especially for long-standing companies, some of which have over a century of data at their disposal. Ensuring data integrity, security, and ownership is paramount, as these form the foundation upon which highly differentiated and transformative models are built. In essence, the synergy between empowered people and robust data governance sets the stage for generative AI to drive meaningful and impactful changes for insurance carriers.
Precisely the global leader in data integrity, in partnership with the Center for Applied AI and Business Analytics at Drexel University’s LeBow College of Business, surveyed 565 data and analytics professionals on their organizations’ data strategies, priorities, challenges and the state of data trust and AI readiness. The results are in, read the report today! 2025 Outlook: Essential Data Integrity Insights