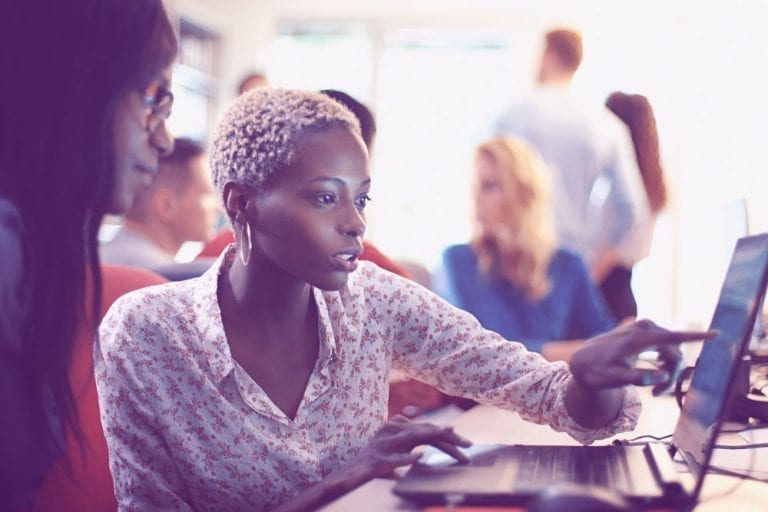
Data Profiling Plus Data Quality Equals Better Analytics
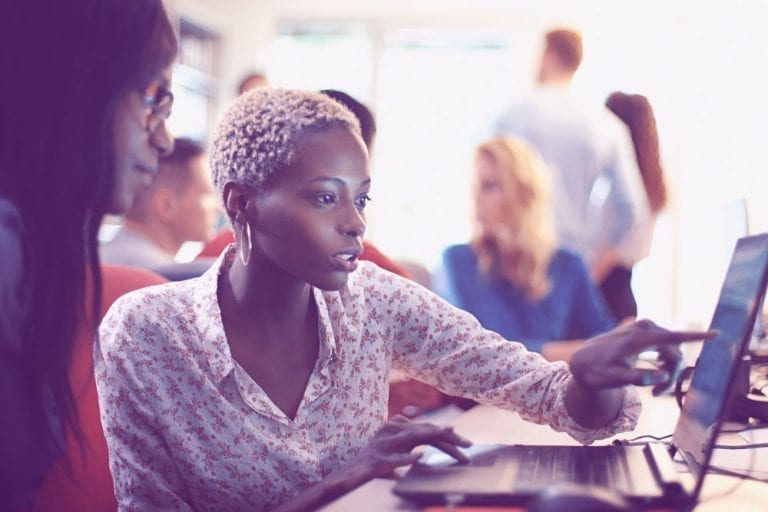
Today, more than ever, big data is king. It is indispensable for functions such as advanced analytics, business intelligence, machine learning, AI, data profiling and data governance. For many modern enterprises, the ability to extract maximum value from corporate data and data profiling tools has a direct bottom-line impact.
But the value of the information a company can derive from its data depends directly on the quality of that data. In our recent study, more than 72% of respondents said that sub-par data quality had negatively affected business decisions. Adds Precisely CTO Dr. Tendü Yogurtçu, “Almost half also found untrustworthy results or inaccurate insights from analytics were due to a lack of quality in the data fed into downstream applications such as AI and machine learning.”
Corporate data profiling tools often comes from diverse sources within and outside of the organization. In order to assure its usability, each dataset must meet the required data quality standards. Assessing data for those characteristics is the role of data profiling.
How data profiling works
To extract maximum value from any set of data, you must understand both its content and its context. Data profiling allows you to comprehensively examine your data to:
- Determine its quality in terms of accuracy, consistency, completeness, and validity.
- Understand the logical relationships between the data types and datasets that make up the source data pool.
There are three basic aspects of data profiling:
- Structure discovery – focuses on ensuring that the formatting of all data is consistent with a chosen standard.
- Content discovery – aims at eliminating inconsistencies, ambiguities, and inaccuracies to ensure the quality of each individual item of information.
- Relationship discovery – characterizes key relationships between data items, including the similarities, differences, connections, and associations between information from different sources.
Data profiling is typically employed when moving data from one system to another, such as from a transaction processing system to a corporate data warehouse.
Read our eBook
Governing Volume: Ensuring Trust and Quality in Big Data
As the volume and variety of big data sources continue to grow, the level of trust in that data remains troublingly low. Discover how a strong focus on data quality spanning the people, processes and technology of your organization will help ensure quality and trust in your analytics that drive business decisions.
Trillium DQ for Big Data provides comprehensive insight
Trillium DQ for Big Data is the industry leader in data profiling tools and data quality. It provides a single, integrated solution that standardizes, cleanses, and validates data on both distributed and cloud platforms.
Unique benefits of Trillium DQ for Big Data include:
- Runs natively in distributed processing environments to deliver data profiling and data quality both on-premises and in the cloud. It also enables discovery, registration, and assessment of associated metadata.
- Features a “design once, deploy anywhere” capability that allows you to build and test your data quality projects locally, then deploy them to Big Data environments such as Hadoop MapReduce with no necessity for re-coding or tuning.
- It comes with hundreds of built-in business rules to allow you to quickly identify and address data governance and compliance issues.
- Automatically manages the technical aspects of executing data profiling and data quality tasks.
- Has an intuitive user interface that does not require specialized technical expertise.
When designing your data profiling project, it’s critical to take the specific characteristics of each data source into account. For example, different use cases, such as Big Data analytics or machine learning, typically have different requirements for data cleansing, format standardization, or pattern matching.
Careless application of generic cleansing or formatting regimes can actually strip out information that is necessary for a particular use case. That’s why having an in-depth understanding of the data extracted from each source is crucial. The Trillium DQ for Big Data user interface allows you to quickly select different data sources and perform data profiling to characterize them individually.
With Trillium DQ for Big Data, you can ensure that your advanced analytics, business intelligence, machine learning, AI, and governance tasks have a base of current, consistent, validated, and reliable information to provide insights that create bottom-line value for your organization.
For more information, read our eBook: Governing Volume: Ensuring Trust and Quality in Big Data