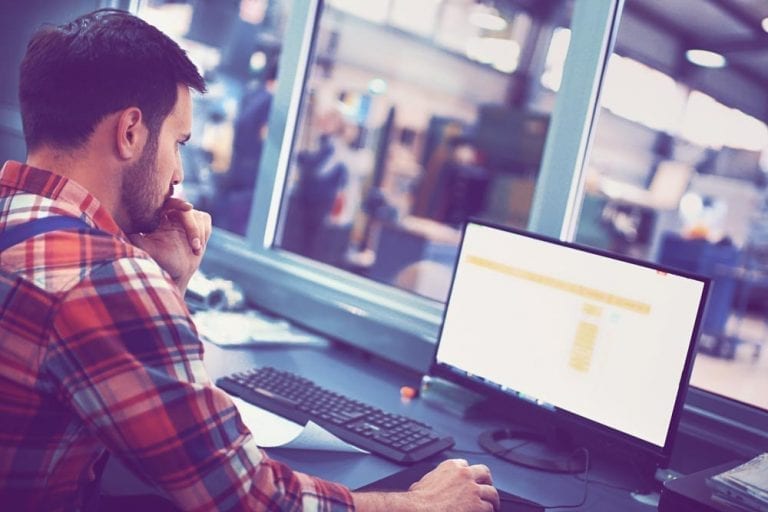
Data Integration 101: What It Means and Why It’s Important
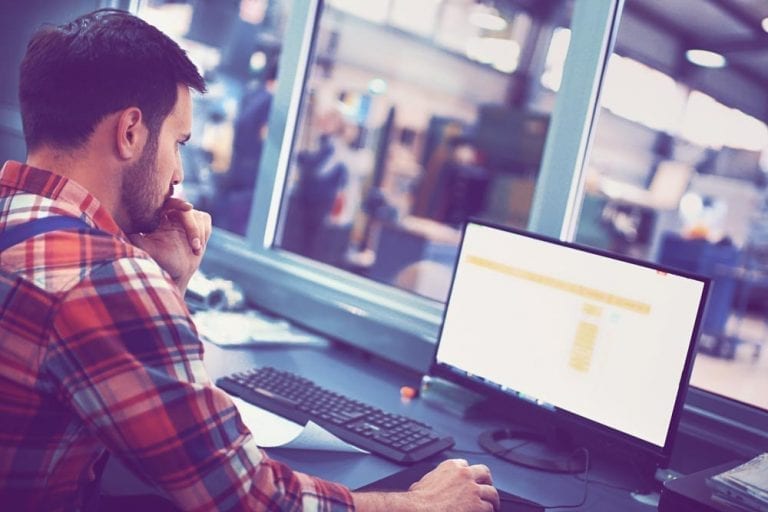
Leveraging data integration for vital insights
How can business intelligence analyses be effectively conducted on data that comes from many different sources and locations, each with its own unique formatting standards? Solving that problem is what data integration is all about.
Enterprises today generate huge amounts of data in their daily operations. Some of it is produced by the sales, marketing, and customer service arms of the business. Other parts may arise from the company’s financial transactions, or perhaps its research, development, and production activities. Each source contributes its part to a pool of data that, when taken as a whole, can be analyzed to reveal strategically vital information.
What is data integration?
IBM defines data integration as “the combination of technical and business processes used to combine data from disparate sources into meaningful and valuable information.”
In essence, data integration produces a single, unified view of a company’s data that a business intelligence application can access to provide actionable insights based on the entirety of the organization’s data assets, no matter the original source or format. The pool of information produced by the integration process is often collected into a data warehouse.
Why it is important for business
Business intelligence applications can make use of a comprehensive set of information provided through data integration to derive important business insights from a company’s historic and current data. By providing executives and managers with an in-depth understanding of the company’s current operations, as well as the opportunities and risks it faces in the marketplace, it can have a direct bottom-line impact.
Also, the data process is often indispensable for collaborating with outside organizations such as suppliers, business partners, or governmental oversight agencies.
One important application of data in today’s IT environment is in providing access to data stored on legacy systems such as mainframes. For example, modern big data analytics environments such as Hadoop usually are not natively compatible with mainframe data. A good solution will bridge that gap, making an organization’s valuable legacy data available for use with today’s popular business intelligence applications.
How it works
A variety of approaches, both manual and automated, have historically been used for data integration. Most solutions today make use of some form of the ETL (extract, transform, load) methodology.
As the name implies, ETL works by extracting data from its host environment, transforming it into some standardized format, and then loading it into a destination system for use by applications running on that system. The transform step usually includes a cleansing process that attempts to correct errors and deficiencies in the data before it is loaded into the destination system.
Advantages of a dedicated solution
Historically, integration has often been performed in an ad hoc manner by individuals charged with producing reports based on data from different systems or applications. But when manual processes are used, or even if several generic software tools are cobbled together to complete the task, extracting needed information from disparate streams of data in a timely fashion can be extremely time-consuming, difficult, and error-prone.
A well-designed data integration solution, such as Precisely Connect, will automate the process, and allow the creation of blended datasets without manual coding or tuning. Connect software provides connectivity between a wide variety of sources (including mainframes, Databricks, Snowflake, etc) and can even be used to optimize other integration solutions.
For more information, get our checklist of the 10 key features when hiring a data integration vendor. Click to read The Data Integration Top 10.