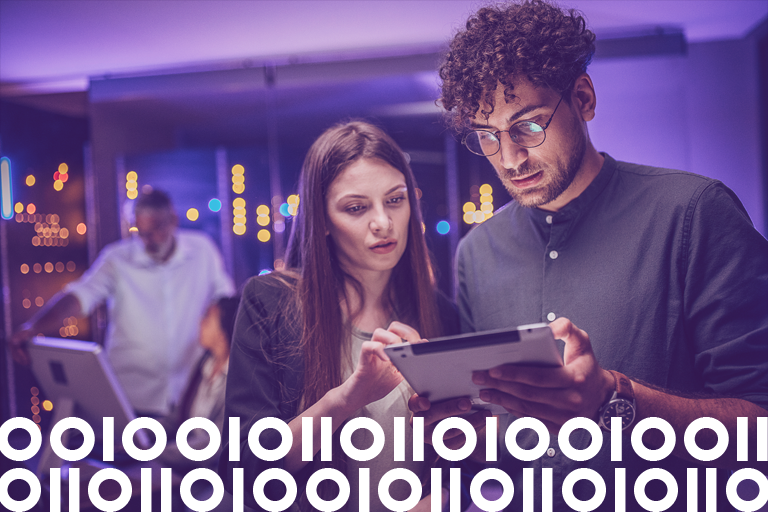
Your Guide to Unlocking Trusted AI with Reliable Data
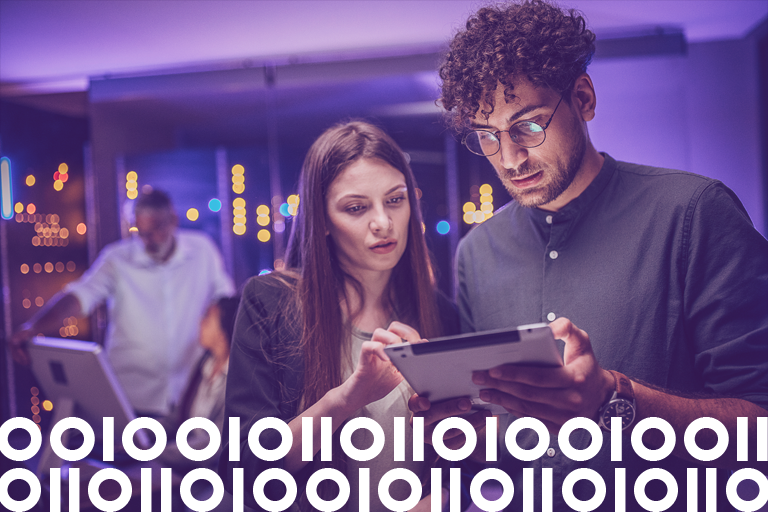
What if every decision, recommendation, and prediction made by artificial intelligence (AI) was as reliable as your most trusted team members?
This isn’t a distant dream – it’s a tangible reality with trusted AI. From enhancing decision-making and productivity to delivering personalized customer experiences and sparking innovation, trusted AI is your key to gaining a competitive edge in today’s fast-paced market.
But how can you make sure your AI can be trusted? That’s where it gets more complicated.
For all its transformative power, there are crucial steps you need to take first to prevent AI biases, inaccuracies, and more.
As you navigate the complexities of integrating AI into your operations, it’s essential to understand data integrity – data with maximum accuracy, consistency, and context – and its pivotal role in ensuring AI reliability. Let’s explore how to harness the full potential of AI technologies by making your data AI-ready.
The Risks of Rushing Without Readiness
The power of AI isn’t just in its ability to process information at an unprecedented scale but in doing so with accuracy and integrity that you can rely on.
While the temptation to dive headfirst into AI is understandably strong, there are risks to doing so without laying the proper groundwork– something that proves easier said than done.
A striking revelation from the 2023 Gartner IT Symposium Research Super Focus Group showed that only 4% of businesses considered their data AI-ready. This big wake-up call emphasizes the urgent need for organizations to enhance their data management practices to pave the way for trusted AI applications.
Remember some of the newsworthy AI mishaps of 2023? From AI-generated briefs filled with inaccuracies to scandals that never were, these incidents highlight how easily inadequate data can create flawed results with significant business implications – while simultaneously demonstrating the importance of feeding your AI with trusted, high-quality data.
The lesson here is clear: your AI outputs will only be as strong as the quality of the data feeding them. Next, you’ll see valuable AI use cases and how data integrity powers success.
eBook
Trusted AI 101: Tips for Getting Your Data AI-Ready
Future-proof your AI today with data integrityIt’s time to maximize the potential of your artificial intelligence (AI) initiatives. Get inspired by valuable AI use cases, and find out how to overcome bias, inaccurate results, and other top challenges. Technology-driven insights and capabilities depend on trusted data.
AI Success in Action: Powered by Data Integrity
From enhancing customer experiences with AI-powered chatbots to optimizing operations through AI-assisted workflows, the possibilities with AI are nearly limitless. While use cases may vary, each success story shares a common foundation: data integrity.
Whether it’s making personalized recommendations, automating complex workflows, or training machine learning models, the accuracy, consistency, and context of your data determine the effectiveness of your AI solutions. Let’s dive deeper into two of the countless examples:
1. AI recommendations
The benefits: Faster, more personalized recommendations.
How it’s done: An AI recommender system is a sophisticated technology that leverages AI and vast amounts of user data – like past preferences, behaviors, and interactions – to suggest tailored products, content, or services.
Why data integrity is essential: Recommendations will only be accurate and relevant if the data has integrity, especially the critical element of data quality. Data integrity also powers more effective training of models, resulting in improved recommendation performance, increased user satisfaction, and higher engagement levels.
2. Chatbots
The benefits: Efficient and personalized assistance that increases user engagement.
How it’s done: Chatbots built on large language models (LLMs) can deliver natural, contextually rich responses to user prompts. An LLM is a type of foundation model (FM) trained on vast amounts of textual data to understand and generate human-like language. A chatbot’s ability to dynamically generate responses based on the ongoing conversation sets them apart, enhancing user engagement across multiple industries and use cases like customer support.
GenAI’s impact on customer service is already being felt. The National Bureau of Economic Research surveyed 5 5,179 customer support agents and found an average productivity increase of 14% when exposed to AI tools. This number goes up to 34% for novice workers.
Why data integrity is essential: High-quality chatbot responses require LLMs trained on high-quality, complete data. An FM will take the data as-is to generate a response – it won’t correct issues. Data integrity is essential to bringing together all relevant data for the GenAI model and ensuring it’s accurate, consistent, and contextualized.
Feeling inspired yet? Then, let’s move on and discuss the most important considerations to remember as you begin to tackle the challenges that hold you back.
Overcoming AI Challenges: Three Key Considerations
Facing hurdles like compliance issues, lack of contextually relevant AI outputs, and the ever-present risk of AI bias? You’re not alone.
The journey to trusted AI involves navigating these challenges with strategic data integration, quality management, and governance practices. Adopting a holistic approach to data integrity ensures your AI initiatives deliver trustworthy and unbiased results, fostering trust and driving adoption across your organization.
To achieve trusted AI outcomes, you need to ground your approach in three crucial considerations related to data’s completeness, quality, and context. Here’s a snapshot of how you can address each:
- A more complete dataset helps you realize the full potential of your AI. You need to break down data silos and integrate critical data from all relevant sources. This ensures your AI models can access a comprehensive dataset, minimizing bias and enhancing accuracy.
- Fuel your AI applications with trusted data to power reliable results. Implement robust data quality measures to ensure your data is accurate, consistent, and standardized and a governance framework to maintain its quality over time. High-quality data is the fuel for reliable AI predictions and decisions.
- Add context to your data for more relevant and nuanced responses. Enrich your data with spatial insights and third-party information to ensure accuracy, depth, and relevance for your AI applications.
As you start navigating these data integrity considerations for AI, it might feel overwhelming – but it’s important to remember that you don’t need to do it alone. Leverage the power of trusted data to fuel your AI applications by combining capabilities from Precisely and Amazon Web Services (AWS).
From modern data pipelines to cloud-native solutions for data quality and governance, you’ll have the tools and resources to make your AI initiatives successful.
The Bottom Line: There’s No Trusted AI Without Data Integrity
AI’s possibilities are as vast as the data it learns from. That means prioritizing data integrity isn’t just beneficial – it’s essential. You unlock the full potential of trusted AI that drives innovation, enhances efficiency, delivers unparalleled customer experiences, and more by ensuring your data is accurate, consistent, and contextualized,
What are your top use cases for AI? How can trusted AI transform your business?
Remember, the journey to trusted AI begins with a single step: ensuring your data is ready for the challenge. Future-proof your AI initiatives by embarking on the data integrity journey today.
For a deeper dive, including practical tips and best practices, read the full eBook: Trusted AI 101: Tips for Getting Your Data AI-Ready