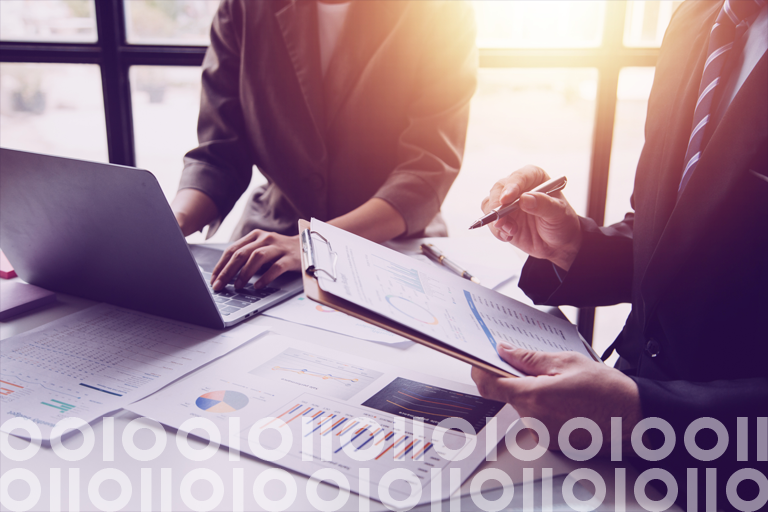
How Data Observability Helps to Build Trusted Data
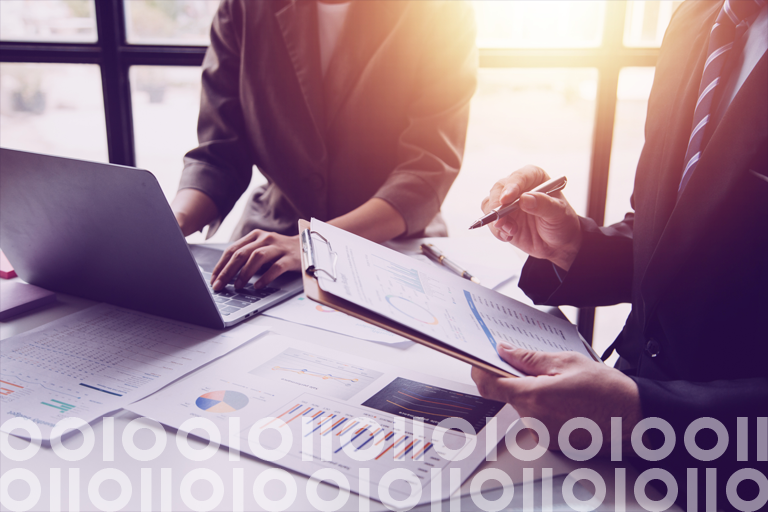
Author’s note: this article about data observability and its role in building trusted data has been adapted from an article originally published in Enterprise Management 360.
Is your data ready to use?
If we look by the numbers, it doesn’t seem like a promising outlook for many:
- Nearly half of newly created data records contain at least one critical error (Harvard Business Review)
- Only 46% of data and analytics professionals have “high” or “very high” trust in the data they use for decision-making (2023 Data Integrity Trends and Insights Report)
At Precisely, we often hear from our customers about the damaging downstream impacts of overlooking even the smallest upstream data anomalies. That’s what makes this a critical element of a robust data integrity strategy.
In a recent piece for Enterprise Management 360, I explored the important role of data observability in gaining – and maintaining – the trusted data you need for powerful decision-making. Below, I’ll recap what you need to know.
What is Data Observability?
There’s more data being created than ever before – approximately 328.77 million terabytes per day. And the connectivity between systems and data sources is also at an all-time high, meaning we need to be more cautious and aware of even small and simple changes to any of those sources.
Let’s think back again to the question I posed above: is the data flowing through your organization ready to use?
Regardless of your industry or role in the business, data has a massive role to play – from operations managers who rely on downstream analytics for important business decisions, to executives who want an overview of how the company is performing for key stakeholders. Trusted data is crucial, and data observability makes it possible.
Data observability is a key element of data operations (DataOps).
It enables a big-picture understanding of the health of your organization’s data through continuous AI/ML-enabled monitoring – detecting anomalies throughout the data pipeline and preventing data downtime.
At its core, data observability can be broken down into three primary components:
- Discovery: collecting information about your data assets using a variety of techniques and tools
- Analysis: identifying any events that have the potential to adversely affect data integrity
- Action: proactively resolving data issues to maintain and improve data integrity at scale. The best data observability tools incorporate artificial intelligence (AI) to identify and prioritize potential issues.
Why is data observability so important?
Simply put: traditional methods of managing data quality no longer work in today’s digital age. The longer a data issue goes undetected, the greater the impact and costs for the business.
Manually identifying and solving problems is too risky and time-consuming, but observability helps you be more proactive – reducing risk and saving countless hours, dollars, and headaches.
Read our Report
TDWI Checklist Report: Succeeding with Data Observability
For even more data observability insights, read our report which covers the five best practices for using observability tools to monitor, manage, and optimize operational data pipelines.
Data Observability vs. Data Quality
Given they share similar aims, it might be easy to conflate the idea of data observability with data quality. But while they do complement each other, it’s important to note their key differences:
Data quality focuses on clearly defined business rules and analyzing individual records and datasets to determine whether they conform to said rules. For example, customer records should be consistent across all systems and databases, especially if they hold sensitive or personal information.
Data observability focuses on anomaly detection before data quality rules are applied. Drastic sudden changes in the volume of data values, for example, could indicate an upstream issue with the data. Longer-term data trends also require attention. These patterns enable the recommendation of targeted data quality business rules for ongoing data integrity actions.
Is Data Observability Right for You?
How do you know if data observability is right for your business? Here are a few questions to ask yourself to get started:
- Are data-driven decisions becoming even more important in your organization?
- Have you ever had a report with inaccurate data get to your executives before anyone realized there was an issue?
- Is your organization relying more on advanced analytics for recommendations?
If your answer to any (or all) of these questions is “yes,” then it’s time to adopt data observability as a critical element of your overall data integrity strategy, and start building more trust in your data.
Data Observability with an integrated data catalog:
- often provides a single searchable inventory of data assets and allows technical users to easily search, explore, and understand their data
- enables users to easily understand data lineage and visualize the relationships among various datasets
- enhances collaboration by providing tools like commenting capabilities, monitoring, auditing, certifying, and tracking data across its entire lifecycle
Ready to Begin?
As businesses increasingly take steps towards cloud transformation – modernizing data environments to support advanced analytics and drive more powerful decision-making – a company’s ability to trust its data becomes paramount.
Data observability helps you understand the overall health of your data, reduce the risks associated with bad analytics, and proactively solve problems by addressing their root causes – all of which contribute to the confident decision-making you need to thrive.
Find out how the Data Observability service of the Precisely Data Integrity Suite helps you proactively identify and resolve data issues to boost data reliability and minimize disruptions.
For even more insights, read the TDWI Checklist Report: Succeeding with Data Observability – covering the five best practices for using observability tools to monitor, manage, and optimize operational data pipelines.