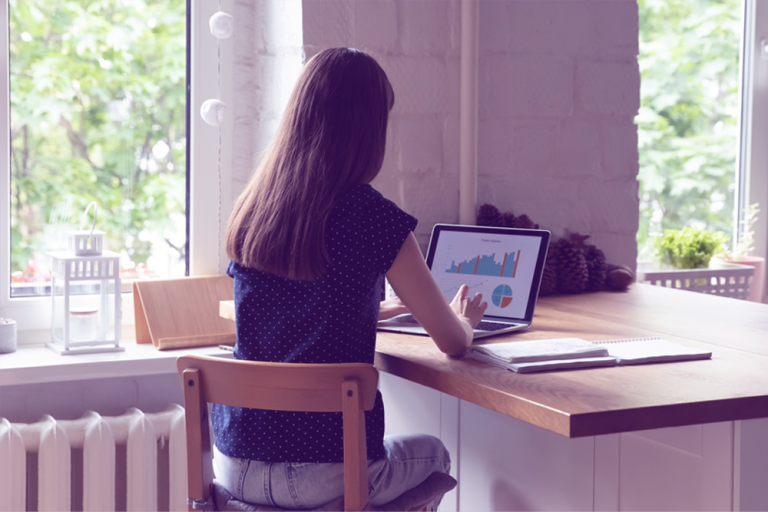
Data Governance vs Data Quality: Which Comes First?
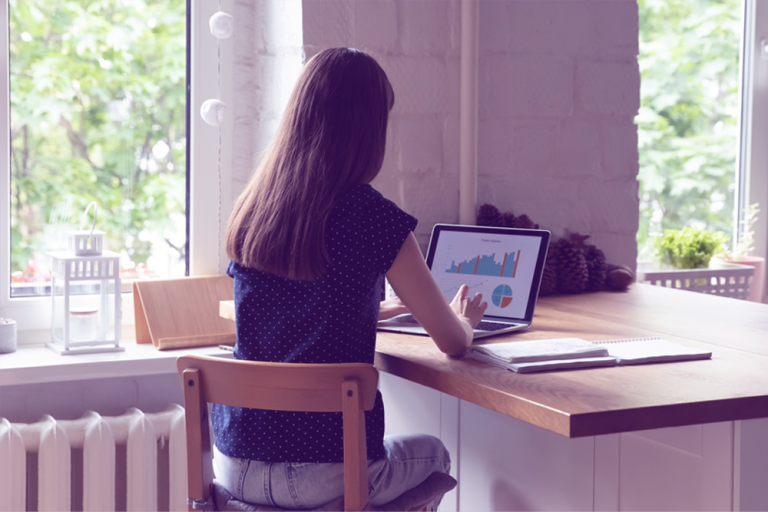
Data governance vs data quality: both are essential to the other’s success, and to making bigger, better decisions based on data you can trust.
A solid data governance program is the foundation for enterprise-scale data quality, providing a framework that aligns people, processes, and technology. Quality data is complete, verified, and validated – and it also underpins any data integrity plan.
In today’s connected world, organizations are generating astronomical volumes of data at accelerated rates.
But whatever the size of the data environment that houses all this data – be it a traditional data warehouse or a big data environment like a data lake – the common denominator is raw data, ingested from multiple internal and external sources, and typically of unknown quality.
As organizations prioritize data management tasks and deliverables, a great debate often ensues regarding the first step to tackle: data governance vs. data quality.
In the realm of data management, there are many terms that may be used interchangeably among employees, typically due to similarities in function and usage within the ecosystem.
Data quality and data governance certainly aren’t synonymous, but can sometimes be confused – and for good reason. They’re both essential to the other’s success, and a solid data governance program is the foundation for enterprise-scale data quality. Without governance, the quality of data is often misunderstood and therefore mistrusted.
If your organization is tackling data management initiatives, you need to ensure solid data governance in order to take on the challenge of data quality.
Data quality relies on good data governance
When data quality is improved within the framework of a solid data governance program, it not only assures data’s accuracy, completeness and relevance, but also tackles data credibility. Data quality tools may include parsing and standardization, cleansing, profiling, and monitoring to improve data quality, but they need to occur within a solid data governance framework.
Here’s what you need to consider as you start the journey:
- First, establish a clear understanding and ownership of data assets, including where that data came from and how it’s used across all facets of the enterprise.
- Identify sensitive data and handle it in accordance with regulatory and compliance rules. Responsibility and accountability must also be established.
- Identify data stewards, owners, and stakeholders who can define usage and act as resources for business users. Data assets are valuable, and that value is only rivaled by the expertise of your organization’s human capital. It’s critical to leverage these together.
All of this builds a culture of collaboration and improves data understanding and utilization.
Data governance serves many functions: establishing a business glossary and data dictionaries, tracking data lineage, ensuring compliance, preventing data breaches and protecting data assets, and creating uniform policies and procedures.
Fundamentally, though, it’s about laying the foundation for data understanding. Data understanding ensures that data is used appropriately to maximize value and mitigate risk, while encouraging business users to increasingly leverage those data assets for analytics that can lead to bigger, better decisions.
To tie this all back to our question of data governance vs. data quality: business users will only increase utilization when they not only understand those assets, but trust their quality as well – and that’s where data quality comes in. Data quality can be tracked, scored, and monitored as part of a comprehensive data governance solution.
Read our report
Fueling Enterprise Data Governance with Data Quality
The time to start the journey is now. To learn more about how your organization can achieve excellence in data governance, download our free report.
Data governance protects the data supply chain
Data governance provides data organization and understanding in a clear-cut framework of responsibilities, policies, and standards. But data assets need to be continuously managed throughout the data supply chain, as data transforms and use cases evolve over time. Data lineage and definitions are not static, and as data moves from sources through systems, the quality can often be impacted.
“Protecting” data assets refers not just to data security, but to a commitment to preserve and improve data integrity. A comprehensive governance program with data controls and quality monitoring can prevent many downstream data-related problems and help reduce data misunderstanding and misuse. Clearly defined workflows and user-friendly visualizations will also increase accountability and provide for smooth issue escalation and resolution.
How your organization should approach data governance
The right approach to data governance requires a business-centric, enterprise-wide strategy to maximize both the quality of an organization’s data and the insights it provides.
The solution should facilitate a comprehensive understanding of an organization’s data landscape, enabling data owners, data stewards and data consumers to effectively manage, share, and utilize data to extract maximum business value. The solution should also incorporate analytics and apply machine learning algorithms to identify data quality gaps across the enterprise.
Data governance vs. data quality: the final word
So what’s the key takeaway from the data governance vs. data quality debate, in short?
Ultimately, improved data quality is a key benefit from the implementation of a comprehensive data governance framework.
Data governance comprises the broader, strategic, enterprise vision of recognizing and managing data as a valued enterprise asset – and this framework needs to be established first in order to boost data quality, understanding, and collaboration throughout your organization.
To learn more about how your organization can achieve excellence in data governance, read our eBook “Fueling Enterprise Data Governance with Data Quality”.