White Paper
Data Quality is a Hard-to-Pin-Down Metric
At a time when data is viewed as one of an organization’s most valuable assets, it’s no surprise that companies are consuming huge volumes of data. Yet data has no intrinsic value. It only has potential value. While that potential value is vast, it is largely contingent on three main data quality metrics:
- Data quality—the foundation of data trust and reliability
- Data governance—to ensure data is easily understood, readily found, appropriately accessed and correctly used
- Data analysis—to turn raw data into business insights
To realize data value, companies must generate actionable business intelligence from quality data to increase innovation, competitive advantage, growth and revenue. When organizations use flawed data for analysis, the inaccurate and unreliable output creates user distrust and low data utilization. Conversely, integrated data quality and data quality-powered data governance build user trust by, helping organizations realize the data value they want and need across every critical system, process and environment.
In this whitepaper, we’ll examine the critical parameters for safeguarding and measuring data quality, including critical quality rules you should implement across the data supply chain. Data quality framework Data governance objectives such as increasing data user understanding and awareness, enabling optimal use of data to the enterprise and ensuring regulatory compliance can only be accomplished by providing a complete view of the data.
The following image provides a complete view of the data based upon three categories of data quality parameters which will be explored in further detail:
- Data representation
- Data value usage
- Data context
This whitepaper examines how companies must generate actionable insights using data quality metrics to increase innovation, competitive advantage, growth and revenue.
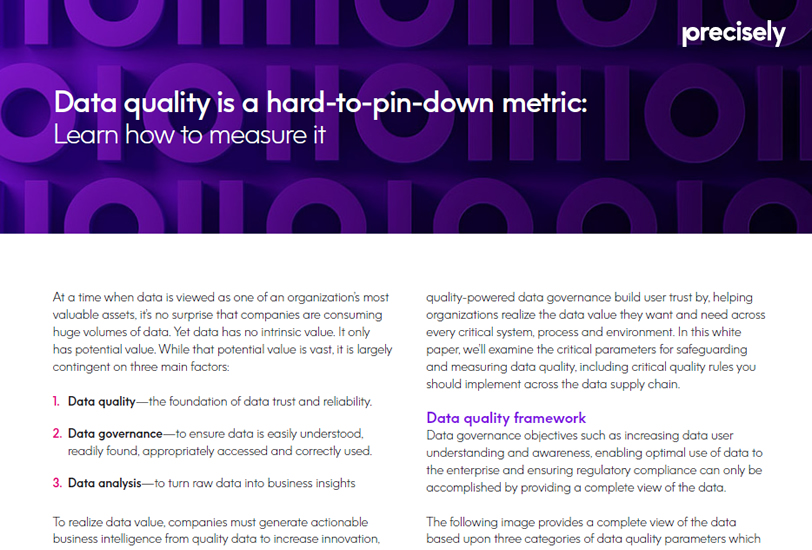