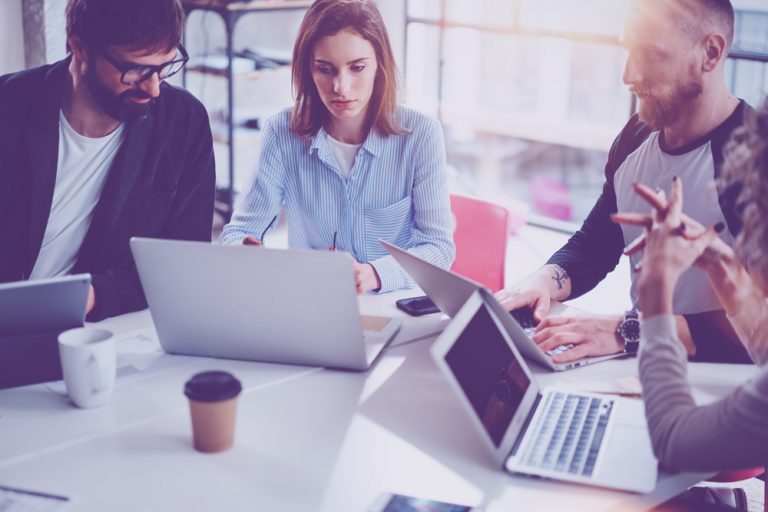
Data Quality Standards Have Evolved: Is Your Strategy Keeping Up?
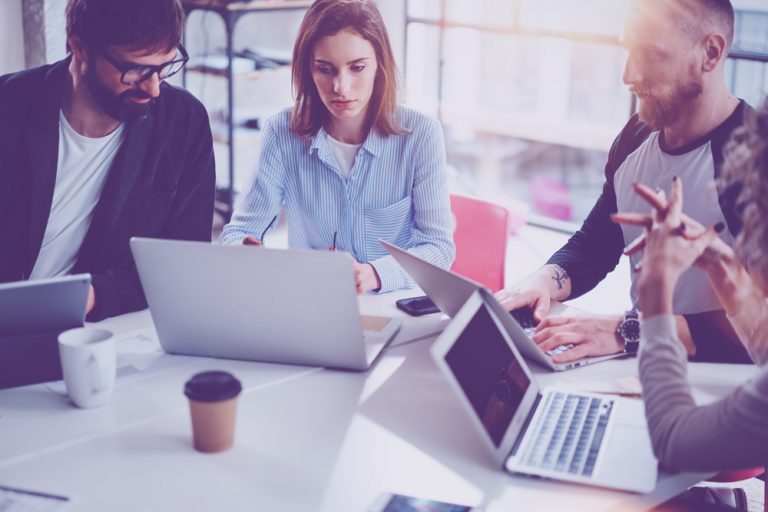
Chances are, you can remember a time when one of your colleagues commented about the need to “clean up” the data in the CRM database or the ERP system. After some period, those systems always seem to accumulate duplicate records, and some portion of the data becomes obsolete. That need hasn’t gone away, but today’s data quality challenges encompass far more than just the need to “clean up the data.”
Today’s organizations are dealing with a multitude of different software systems and data sources. In some cases, those are large-scale transaction processing systems like ERP or e-commerce platforms. In other cases, they house critical customer information, driving sales pipeline activity and marketing automation. Sometimes they are simple ad hoc solutions to everyday problems, such as spreadsheets or homegrown databases.
In addition to this proliferation of systems, organizations are seeing a higher volume of data than ever before. Email analytics, clickstream analysis, and mobile and IoT data add even more complexity to the mix. When you think about the challenges of dealing with so many systems, whether or not they are connected to one another, the focus shifts away from “cleaning up the data” to gaining a more consistent, accurate, and holistic view of the enterprise and its critical data.
Over the past two decades, a dramatic change in the way organizations understand data quality has occurred. Consider some of the facets of this change.
From batch mode to real-time
Data is the lifeblood of modern businesses. It follows, therefore, that their data must reflect what is happening in real time. It’s no longer sufficient to operate on a 24-hour delay (or longer). That’s certainly true of business intelligence, which provides visibility to events and conditions on the ground, but it’s also true for transactional integrity. Airline customers expect that when they make a reservation over the phone, it will show up in their online frequent-flier account right away and that their mobile app will show the update as well.
In a world where real-time data must flow seamlessly from one place to another, data quality cannot be a periodic undertaking. It must be integrated into the day-to-day activities of the business, ensuring that information is consistent, complete, accurate, and available at all times.
Read our eBook
4 Ways to Measure Data Quality
See what data quality assessment looks like in practice. Review four key metrics organizations can use to measure data quality
From reactive to proactive
Today’s organizations simply cannot afford to wait until data quality becomes a problem. Given the volume and velocity of data coming from multiple systems, problems are inevitable. Customer databases decay at a fairly predictable rate, as new consumers enter the market, and others pass away. People move to new residences, or to different countries altogether. Similar forces affect B2B data quality. Businesses close up shop. New businesses open. Personnel leave and are later hired at other companies.
Organizations must take a proactive approach to data quality strategy, or they risk ending up with more and more meaningless data that wastes money and undermines business results.
From manual data cleansing to automated data re-engineering
The real-time nature of today’s businesses, combined with the need for a proactive approach, calls for automated processes that are geared toward data engineering rather than a more simplistic data cleansing approach. An effective data quality initiative is predicated on a comprehensive discovery process in which an organization’s data assets are first cataloged and profiled. That must be followed by a rules-based approach to detecting and fixing data quality issues as soon as they arise. While automation cannot fix every issue that emerges, enterprises must consider how data quality processes can scale effectively. By automating as much of the process as possible, scale can be achieved.
From silos to a holistic enterprise approach
As most enterprises have moved from large and relatively comprehensive information systems to a more diversified collection of applications and systems, there is a vastly higher risk that data across those various systems will be increasingly out of sync. Even if those systems are reasonably well integrated, the data spread across various databases will eventually lack consistency, unless data quality is understood from a holistic, enterprise-wide perspective.
It is no longer reasonable to treat data quality as an application-specific undertaking. It must be part of a broader strategy for ensuring that the information stored within various platforms is complete, consistent, and accurate throughout the enterprise.
The bigger picture: Enterprise data integrity
This holistic view of data comprises part of an even larger picture, that is, data integrity. At Precisely, we view data integrity as having four key pillars:
- Enterprise-wide data integration breaks down silos and establishes reliable, manageable, scalable mechanisms that enable data to flow seamlessly between systems and applications throughout the organization.
- Data quality ensures that information is consistent across all those systems and applications and that stakeholders can trust that data and the business insights that it provides to them.
- Data enrichment incorporates trusted third-party data to increase the value of an organization’s existing data and add further to the insights that can be drawn from it. Data enrichment supports enterprises in gaining a multifaceted 360° view of the customer.
- Location intelligence adds geospatial context, providing rich detail about the places associated with data elements, the activities that take place there, the people who visit or live in those locations, weather and traffic patterns, long-term trends, and much more.
When all four of these pillars are in place, an enterprise can achieve a myriad of different benefits. They save money by avoiding waste. They gain a deeper understanding of customers, supply chains, competitors, and the wider world in which they operate. They spot trends before the competition sees them. They can act quickly; they gain agility.
The most important thing is to have some kind of data quality assessment plan in place, whatever its details may be. For a deeper dive into data quality measurement, read our eBook: 4 Ways to Meaure Data Quality.