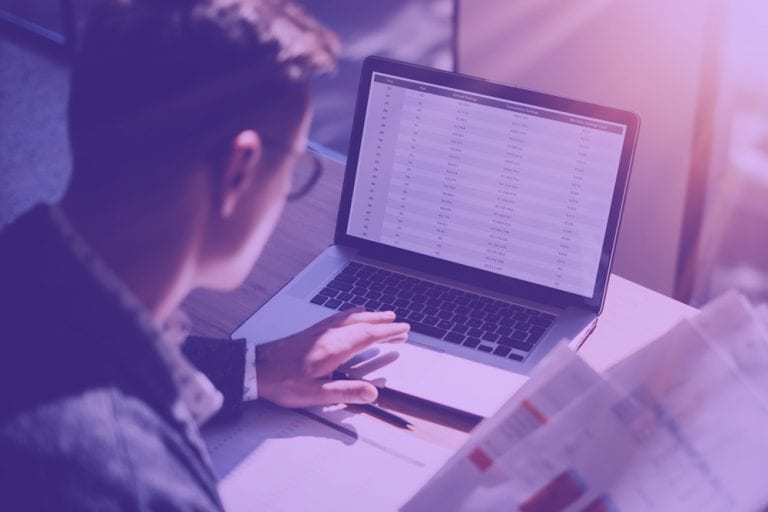
5 Use Cases for Enriched Data in the Insurance Industry
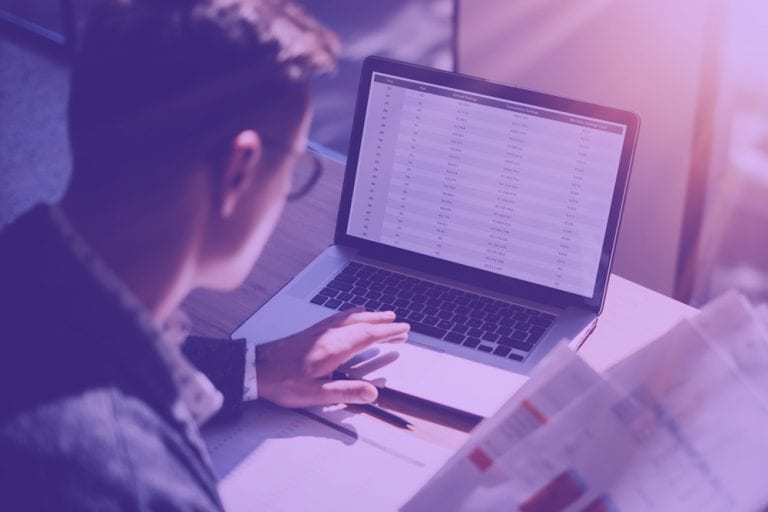
At its core, the insurance industry is about accurately predicting risk, pricing policies to sufficiently cover that risk, and minimizing fraud. Enriched data such as geospatial data adds richness and location context and accurate insurance characteristics to existing information about policyholders, insured properties, and more.
For data-driven insurance companies, data about properties and locations and the physical characteristics of those properties and locations provide a competitive advantage. Here are some examples of how this type of enriched data can improve profitability in the insurance industry.
1. Improve accurate insurance of underwriting and pricing decisions
For many years, the insurance industry has treated location as a coarse-grained data point. Insurance policies, for example, have traditionally factored in information about the city, town, or zip code in which an individual policyholder lives and works. When every insurance company in the market is using that same approach, it serves as what many have used, a “good enough” data point to access a policyholder’s risk and intelligence on how a policy should be priced.
Yet today, insurers have access to so much more location context to make better and more informed underwriting decisions. Take for example two policy holders that live on the same road in the same place. Now, let’s say they live on the longest continuous street in America, Chicago’s Western Avenue which goes about 23.5 miles all the way from the city’s most northern street Howard Street all the way down to the far south side of the city. One home is located near a dangerous intersection in a very congested area. It has a high crime rating and is located in close proximity to a series of abandoned buildings. The other home is in a reduced speed area, near to a school with a lower crime rating and high-end stores within walking distance. Should those policies be priced the same? If you want a highly accurate risk assessment for the policyholder who lives there, the difference in those two scenarios is material. The ability to add enriched data with location context to better understand a specific location matters.
Read the Forbes Insights report
Pinpointing the Issue: Why Hyper-Accurate Location Data Can’t Be Overlooked in Insurance
If you are an insurance professional interested in exploring data enrichment as a path to increased competitiveness, we invite you to download this Forbes Insights report
2. Telematics and the Internet of Things (IoT)
Commercial fleets are equipped with telematics devices that track location, measure driver performance, and assess the operational health of the vehicle. Those kinds of devices are making their way into the consumer market as well, particularly as mobile phones have gained widespread adoption, making it easier to collect and communicate information about a policyholder’s location and driving habits.
One major insurer has even rolled out a usage-based pricing program that relies on the policyholder’s smart phone to track mileage, location, and driving behavior. While drivers may have privacy concerns that preclude them from using such a service, it nevertheless underscores the value of collecting and analyzing highly detailed information about the various factors that impact risk.
In the medical field, wearable sensors and at-home diagnostic devices are capable of providing detailed information about physical activity, vital signs, and alerts to potential emergency situations. As in the case of the telematics example above, many people will prefer to opt out of sharing such information, but to the extent that insurers are able to incentivize data sharing, subprograms can be presented as a win-win scenario. Policyholders who are willing to share that information with insurers may be extended discounts or other benefits in return for their participation.
3. Risk mitigation
Major weather events account for billions of dollars in claims every year. By carefully assessing historical weather patterns and the resulting damage they cause, insurers can predict the likely impact of impending weather events with a reasonably high degree of accuracy. That enriched data helps insurers understand the relative damage done by tornadoes, floods, hailstorms, and other severe weather events in specific areas.
When insurers have a reasonable estimate of where the damage is most likely to occur, they can warn customers in advance–encouraging policyholders to move parked cars into a garage or other protective structure in advance of hailstorm, for example. They can encourage actions that will prevent losses before they occur.
When insurers can predict where the damage will be most severe, they can expedite claims settlement for affected policyholders in those areas. They can also pre-position claims adjusters in the areas that require closer attention and on-the-ground assessment.
4. Fraud detection
With detailed information about risk scenarios and likely damaging impact, it becomes easier to detect fraud with greater accuracy. If predictive models tell us that a particular geographical area suffered a little or no damage, then catastrophic claims located in that area may be singled out as anomalies worthy of additional investigation.
Given the amount of data that is now available about customers, locations, and movement in time and space, data scientists are better equipped to identify anomalies and pinpoint potential cases of fraud than ever before. In addition to the scenario described above, purchasing patterns, economic conditions, and a customer’s past history can be factored in as well.
5. Improve marketing spend
Customer databases have long been plagued with inconsistencies and inaccuracies. Duplicate records are common. In the simplest of scenarios, that means money wasted on duplicate mailings and other customer outreach efforts. But the value of adding context to customer data goes much further than simply deduplicating and updating customer information.
For insurance companies that sell to both consumer and commercial markets, for example, it can be useful to understand the relationships (if any) between a home and auto policyholder and a small business owner who might be in the market for commercial property and liability, workers comp, or other business policies. Traditional customer databases rarely draw a connection between the consumer policyholder who also happens to be the owner of a small business.
Data enrichment also brings context to customer information in the form of major life events such as high school or college graduations, job changes, new home purchases, new children in the family, and more. Each of those events implies some major shifts in a person’s needs for insurance and other financial services.
While differentiated service or product offerings may help win customers, it’s the ability of an insurer to accurately price its premiums that is critical to sustainable, long-term profitability. Accurate insurance in pricing depends on enriched data, the accurate location data used to determine risk.
If you are an insurance professional interested in exploring data enrichment as a path to increased competitiveness, be sure to check out our insurance datasets. We also invite you to read this Forbes Insights report: Why Hyper-Accurate Location Data Can’t Be Overlooked in Insurance.