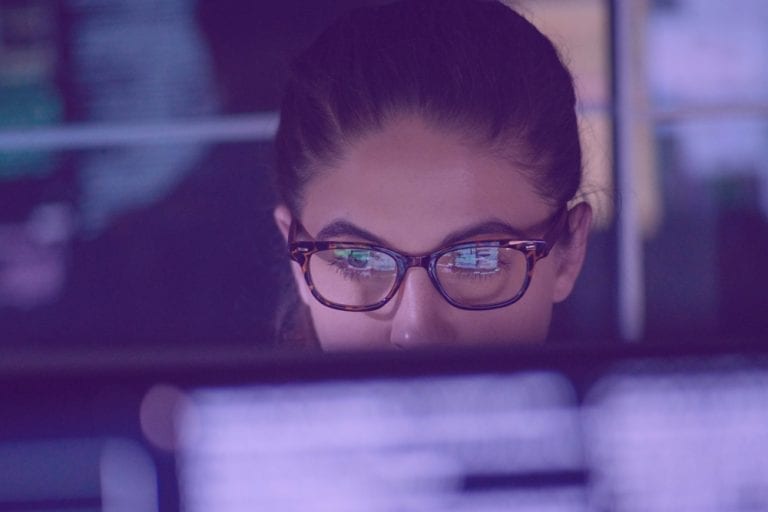
Three Important Considerations for Data Integrity
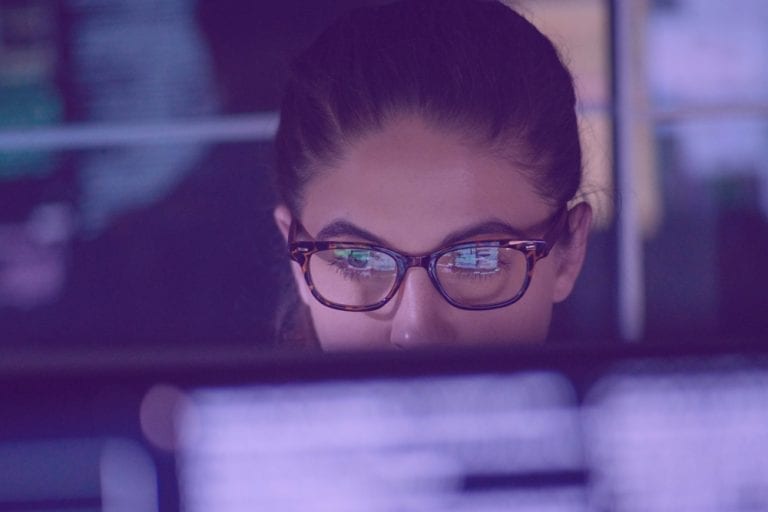
According to Gartner Research, the average financial impact of poor data quality on organizations is $9.7 million per year. Enterprises are putting a greater focus on data integrity than ever before. The volume and velocity of the data available to decision-makers continue to rapidly increase. Data management – and particularly data integrity – are evolving and maturing to keep pace.
Precisely is reshaping the way businesses approach data integrity by helping its customers to address the four key pillars of data integrity: data integration, data quality, data enrichment, and location intelligence.
Let’s look at three trends driving data integrity initiatives.
Context is king
Historically, data integrity initiatives have revolved around accuracy and consistency. As the need for trusted data has become a business imperative, it is now clear that context is also a critical dimension of data integrity. Context is about expanding your understanding of your data with location details, related consumer demographics, points of interest, and so on. It is about understanding the relationships, trends, and patterns in the data.
Financial institutions, for example, need to assess the performance of each branch against a benchmark of some sort. The simple fact that a particular location attracted $5 million in new deposits last year is important, but it is hard to understand the impact of that number or to evaluate its true meaning without a broader context.
If you begin to compare that branch with others throughout the region, then that $5 million number takes on a bit more meaning. Even so, that comparison is limited. After all, the comparison includes branches with different demographics. For example, one might be a small drive-through branch near the city center, whereas another might be a larger full-service facility located near an office park in a shopping mall. The comparison might include locations with vastly different competitive market dynamics as well.
A single data point provides you with a metric that might not be very meaningful, but when you begin to add information about location, branch characteristics, prior-year performance, demographics, traffic patterns, competitive dynamics, and more, you have context.
Context also implies managing all of an organization’s various data sets as a coherent whole. According to IDC, 39 percent of organizations are buying overlapping data sets because they lack a clear strategy for contextualizing the data they have across the enterprise. Multiple conflicting data sets inevitably produce multiple versions of the truth. That, in turn, erodes confidence in the organization’s data assets.
With increased cloud adoption, the number and diversity of data sources continue to increase for most organizations. Hybrid cloud/on-premise scenarios are quite common. The result, all too frequently, is a proliferation of siloed data sets. Data is getting bigger, faster, and more dynamic. That also means it’s getting harder to manage and understand that data in terms of the broader reality in which it operates.
In the era of big data, context is essential. Precisely sees context as a critical driver of value for the enterprise.
2023 Data Integrity Trends & Insights
Results from a Survey of Data and Analytics Professionals
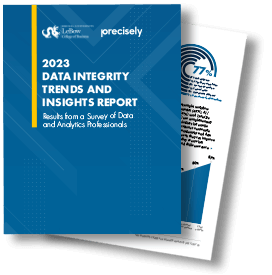
From data governance to data intelligence
Context is just one element of data integrity. Business leaders are shifting from a perspective that calls for better data governance toward a new approach that is centered around driving strategic value in the organization.
The result is that organizations can produce faster and better outcomes with their data than ever before.
It is critical that people throughout the organization can trust that the data they are using is accurate, consistent, and contextually rich such that it can be relied upon when making important business decisions. As trust in the data increases, stakeholders throughout the organization can make the shift from gut-instinct decisions to data-driven results.
AI/ML and the GIGO problem
Artificial intelligence and machine learning have finally come of age. Precisely is leveraging these technologies to automate data integrity and drive better results and higher value for its customers. But AI and machine learning also present significant challenges that call for an aggressive approach to data integrity.
Big data is getting bigger. Unstructured data is taking on a more significant role. Schema-on-read is changing the way we think about data storage and retrieval and the business rules that govern information.
The classic IT conundrum, “garbage in, garbage out” (GIGO), takes on an even greater significance as AI and machine learning continue to gain momentum. Given the volume of data, the complexity of managing data sets from disconnected silos, and the rise of unstructured data, companies that deploy AI/ML technologies without first establishing a clear strategy for data integrity risk producing invalid or misleading results with that technology.
Precisely partnered with Drexel University’s LeBow College of Business to survey more than 450 data and analytics professionals worldwide about the state of their data programs. Now, we’re sharing the ground-breaking results in the 2023 Data Integrity Trends and Insights Report.