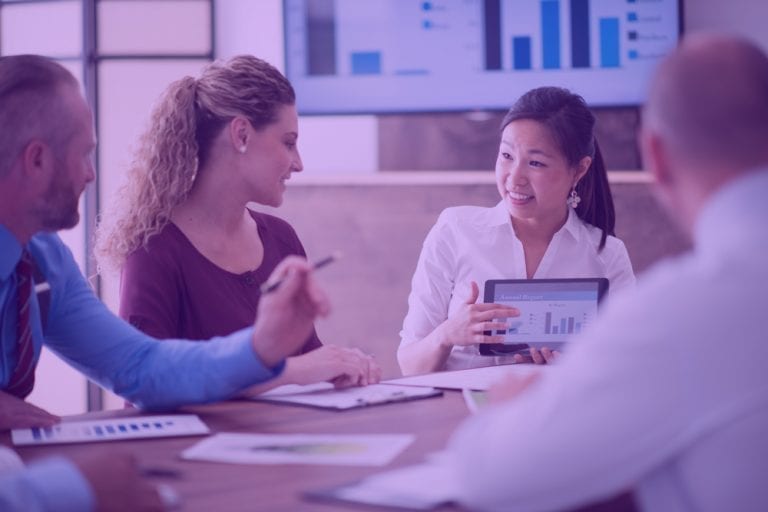
Top Challenges in Global Enterprise Data Management
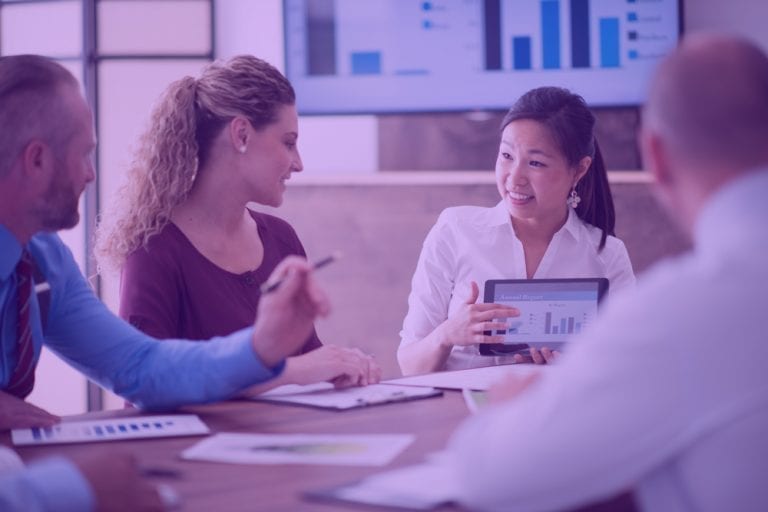
Large enterprises (and even many small and midsize companies) are increasingly dealing with highly complex IT systems, often spread across multiple geographies and systems. In addition to core ERP, they are using specialized software to manage pricing, demand planning, customer relationship management, human resources, point-of-sale, and a multitude of other mission-critical functions within the business. Data management has become more and more challenging, as IT systems have grown to include specialized, domain-specific software.
Companies that operate multiple subsidiaries or business units usually have even more complexity to manage. A subsidiary based in Brazil, for example, may have specialized tax reporting obligations that make it necessary to run specialized ERP software to accommodate those requirements. A recent acquisition might be running on legacy software that cannot be easily changed due to extensive customization. Finally, different business units may be running separate instances of a common software package. Data consistency must be maintained across all of those systems.
Why is data management so important
At face value, it seems obvious that the quality and consistency of data is important. If you can’t get the fundamentals right, such as where your customers live, how much inventory you have, and how much you sold last month, then it is hard to imagine that you will stay in business for very long.
Data quality and consistency are essential to understanding your business at a deeper level, though. Big data and analytics have come a long way in recent years, and companies that want to tap into the hidden assets within their corporate data must be capable of seeing the whole picture. They must be able to do an apples-to-apples comparison of information across multiple geographies, subsidiaries, and software systems.
When a company is running multiple systems that model data very differently, it is essential that the data be harmonized before analytics can do its job. Let’s look at a few examples of how data from multiple software systems can be problematic.
eBook
4 Ways to Measure Data Quality
Assessing data quality on an ongoing basis is necessary to know how well the organization is doing at maximizing data quality. There are a variety of data and metrics that organizations can use to measure data quality. We review of few of them in this eBook.
Staying in sync with data management
The first data management challenge is simply to keep information consistent across disparate systems as information is updated. If a customer does business with you at one subsidiary or location and they update their address information, that information should automatically be updated across your entire business, even if you’re running distinct software systems.
As consumers, many of us have experienced this when shopping online at a business that also operates brick-and-mortar stores. If we buy a product online and want to return it, the local store needs to have visibility for that transaction. Most “click and mortar” businesses have addressed this problem by now, but many of us have experienced this problem as consumers in the past.
The problem of keeping systems in sync often necessitates real-time communication between those systems. Most financial services companies are still running their core business processes on mainframe computers, for example. Those companies also need to support mobile and web applications. Customers expect up-to-the-minute accuracy and consistency across all of their accounts, regardless of how they are accessing that information.
That calls for real-time streaming of data. For legacy mainframe systems in particular, that presents some challenges. Legacy systems often employ data formats and data types that must be deciphered and transformed on-the-fly. All the while, data security and strong data governance practices must be observed. Enterprise-grade integration tools from Precisely are designed to handle these kinds of challenges, operating in conjunction with Precisely’s data quality tools to provide a complete solution to data quality and consistency.
Apples and oranges
Additional data management challenges arise out of the vast differences in the ways that business applications model data. Some ERP systems, for example, maintain a list of vendors distinct from customers or prospects. Others maintain a single table containing all “business partners” including customers, vendors, and prospects. CRM systems often distinguish between prospects and leads.
In addition, some systems view customers as hierarchical entities, with multiple locations or subsidiaries having a distinct master data record, but belonging to a larger parent organization or buying group.
Inventory items, likewise, may be more complex than one would initially imagine. Items with multiple size or color variations may be recognized in some systems as a single SKU, whereas other systems might treat each variant as an individual SKU.
Product formulations might differ slightly across geographies to accommodate regional consumer buyer preferences. Packaging, likewise, might vary across business units based on language, legal requirements, or regional preferences.
More apples and oranges
Even more data management challenges arise simply through small differences in the way data is recorded. One salesperson might enter a new customer record as “ABC, Inc”, whereas another might enter “Associated Buying Company, Inc.”, and another might simply enter “ABC”. Not only does this give you an inaccurate picture of the customer, but it generates inconsistencies (for example, when the customer’s change of address only gets recorded in one location). Enterprise-grade data quality tools are designed to recognize potential variations and trigger corrective action.
Consistency vs. external sources
Finally, companies need to synchronize and validate data against external sources. A simple example would be validating a customer address. Just as variations might arise in customer nomenclature, a single address may be expressed in many different ways. As part of its suite of data quality products, Precisely offers tools to validate and standardize addresses so that they are clear, consistent, and up-to-date.
Getting a clear picture of your business
It is often said that “data is the new oil”. In other words, there is a great deal of value in the various information systems across your entire IT landscape, but it must be extracted and refined if its full value is to be realized. Effective analytics are predicated on having consistent, harmonized data from across the entire business.
The complexity of modern IT systems introduces substantial challenges for global enterprise data management. Fortunately, there are tools that can effectively address those challenges and help enterprises master the complexity of disparate systems while ensuring security, reliability, and good data governance practices.
Read our eBook: 4 Ways to Measure Data Quality to learn more about how to assess your data quality needs.