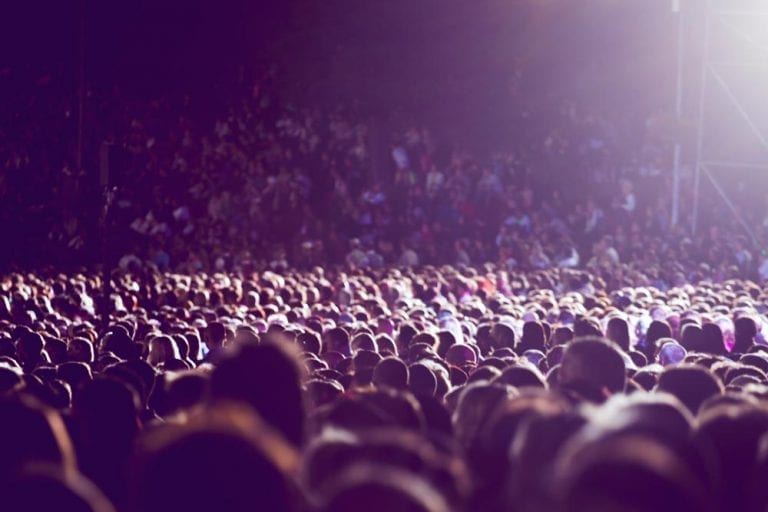
Key Takeaways from Spark+AI Summit
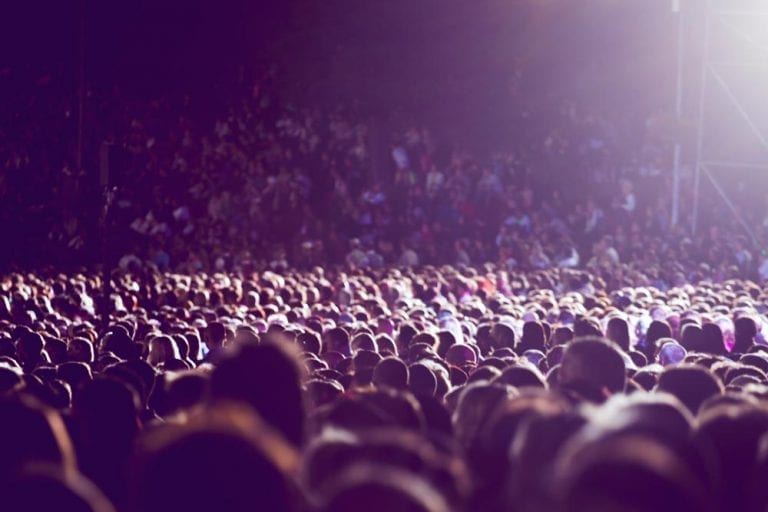
The 2020 Spark+AI Summit 2020 organized by Databricks hosted 60,000 virtual attendees. The keynotes focused on technical updates such as data integration and quality, and included multiple live demos, and use cases.
Spark turns 10 years old
Ali Ghodsi, CEO and Co-founder at Databricks and Matei Zaharia, co-founder and Chief Technologist at Databricks, celebrated Spark’s 10th anniversary by announcing Spark 3.0. The new highlights of Spark 3.0 include query performance enhancements and another step towards ANSI SQL support.
Enabling analytics and data integration
Ali started the keynotes with a call to unite around data. He mentioned that data and artificial intelligence (AI) is a team sport. The goal at Databricks is to unify SQL Analytics with data warehouses to make it easy to explore data and deploy AI models in production.
The theme of enabling analytics resonates with what we hear from our customers at Precisely. In her recent Forbes article, Precisely CTO Tendü Yoğurtçu highlighted the business imperative of managing data integrity to enable trusted business decisions. Complete data, accuracy, and context are vital to the success of analytics projects.
Eliminating data silos with data integration
While most organizations are striving to improve their analytics output, they face many challenges, including siloed, fractured, and incomplete data. Ali shared that Databricks often sees organizations hitting these walls when trying to bring the right people together, with the right data, as quickly as possible.
At Precisely, we often work with our customers to help solve the data silo problem. We see organizations wanting to incorporate a large percentage of business transaction data such as ATM transactions, credit card swipes, insurance claims, and sales transactions into their data strategies. However, this data lives on hard to reach legacy systems such as mainframe and IBM i. To maximize business results, making this data available for analytics is a business imperative.
Read our white paper
A Practical Guide to Analytics and AI in the Cloud with Legacy Data
Businesses that use legacy data sources such as mainframe and IBM i have invested heavily in building a reliable data platform. Lean how you can access legacy data in the cloud for analytics, data science and machine learning.
Moving to the data lakehouse
Ali talked about Delta Lake as a data lakehouse concept. The data lakehouse paradigm combines the structured and reporting capabilities of a traditional data warehouse with the data science, real time, and machine learning capabilities of data lakes. Databricks’ goal with the data lakehouse will be to supply a structured transaction layer in which to enable improved data management across different teams.
Improving data integration and quality
The hardest challenge when building a data lakehouse, according to Ali, is around data quality issues. Manual data entry and integration with third-party data sources can cause information to be missing, duplicated, or incorrect. Machine learning models need clean, trusted data to produce reliable and fair outcomes.
Rohan Kumar, Corporate Vice President of Azure Data at Microsoft, unveiled Fairlearn and InterpretML. These tools help assess fairness in Machine Learning models and expose features that affect model outcomes.
Unlocking successful analytics
To address the main challenges of successful analytics, Precisely has partnered with Databricks – even presenting on our partnership during the Summit. Precisely Connect helps organizations to liberate data from its silos to deploy to the ‘one cloud massive scale’ of Databricks. In addition to Connect, Precisely offers market-leading data integration, data quality, location intelligence, and data enrichment capabilities to deliver trusted, complete data to Delta Lakes.
Precisely’s design once deploy anywhere solution enables customers to integrate data from all sources, including streaming data, traditional data warehouses, and legacy data on mainframe or IBM i. Data can then be cleaned and enriched with location and demographics data. The data pipeline can be easily deployed on-premise, or on a cloud environment like Databricks. Native integration with Delta Lakes enables data scientists to focus their efforts on deriving business insights.
To learn more, read A Practical Guide to Analytics and AI in the Cloud with Legacy Data, a white paper co-sponsored by Databricks and Precisely.