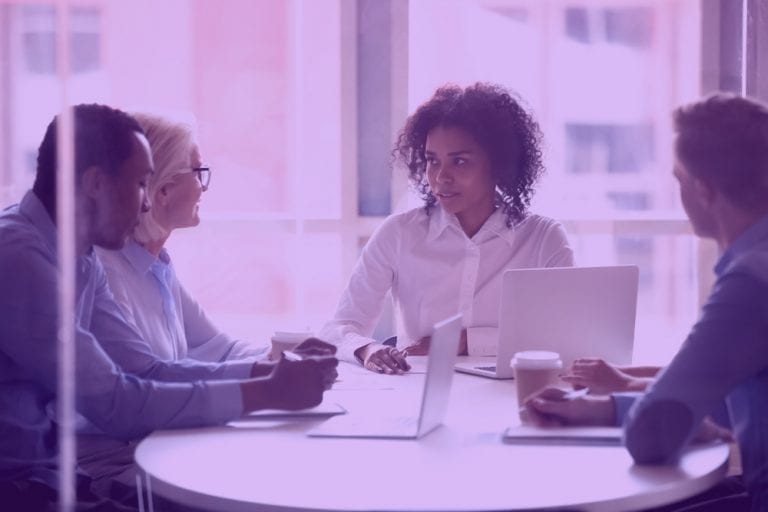
Data Quality: A Financial Services Industry Imperative
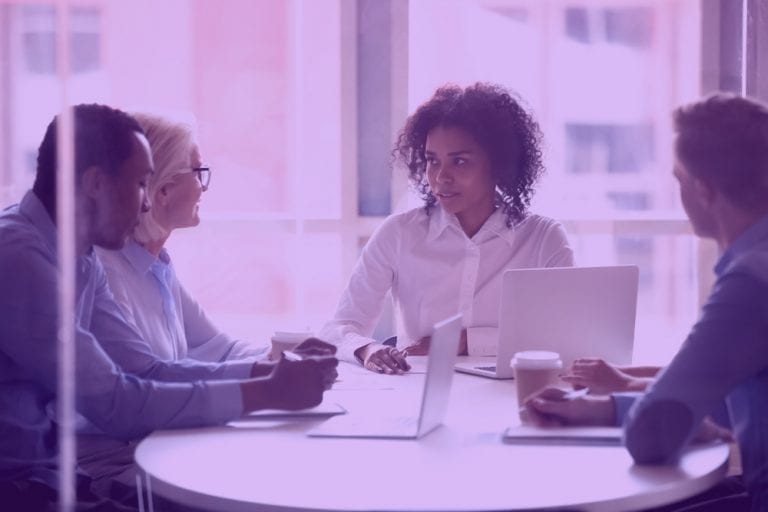
Trust is the keystone of the financial system. Over the past two decades, global financial regulations have established a system of governance intended to ensure and safeguard that trust. Dodd-Frank, Basel III, MiFiD, EU Solvency II, and other major regulatory initiatives have mandated that financial institutions understand where their data is coming from and can attest to its accuracy and validity, as well as keeping it secure. Financial services data quality and security must be proactively maintained to comply with good data governance standards for which regulations are constantly evolving.
To ensure good financial services data quality, business and IT leaders must work together. Multiple departments within the organization have a stake in the outcome; finance, marketing, risk, and compliance all need to know that they can trust the data. That requires a clear vision that defines the elements of good data quality and establishes a working framework and a body of best practices to achieve that vision.
Begin with the “Why”
The business case for financial services data quality initiatives is overwhelmingly clear. It begins with compliance. Meeting regulatory mandates is a priority for all organizations in the financial sector, as governing bodies around the world continue to seek transparency and fend off crisis situations before they happen. The penalties for non-compliance can be severe, and the repercussions for customer trust can be even worse. In this respect, data quality is a “must-have” function rather than a driver of business growth or innovation.
Risk management is next on the list of priorities and is closely related to compliance. Finance obviously also has a stake, as its reliance on data is core to the function of any financial services business.
The impetus for good care quality management does not stop there, however. A company that understands its customers will be better positioned to anticipate their needs and deliver products and services that address those needs, so marketing likewise needs to know that the organization’s data is trustworthy. Let’s look at some of the key elements of data quality.
Read the eBook
Creating an Agile Data Quality Strategy for Effective Regulatory Compliance
Explore how an agile, iterative data quality strategy can help your organization streamline compliance and proactively face new regulations with confidence.
Accuracy
Good financial services data quality practices necessitate a clear set of rules for ensuring consistency and accuracy across datasets. Business logic that dictates conditional or dependent values should be automated. Finance institutions must be capable of validating the consistency of that data across all of their internal systems, as well as with external data sources.
It is estimated that customer data has a half-life of about one year. Financial institutions cannot afford to let their information fall out of step with the world in which their customers live. If a customer moves to a new location, for example, the financial institution should be able to establish consistency across all of that customer’s records. Furthermore, they should be able to cross-reference the customer’s new address to externally sourced address data.
Completeness
Missing information needs to be identified and resolved. Automated processes should be used to identify cases where a default value or dummy value has been entered and to trigger workflows aimed at resolving those missing data elements. However, challenges may arise when such logic is added after the fact. Effective data quality tools can proactively identify incomplete or inconsistent data elements and can route those to the appropriate personnel for resolution.
Changes throughout the customer lifecycle can likewise lead to inaccurate or incomplete data. Mergers and acquisitions can lead to deficiencies in customer information. In the case of individual customers, life events such as marriage or death may likewise trigger problems with data that need to be systematically discovered and resolved.
Standardization
Something as simple as a postal address can take many different forms. Breaking down an address into its constituent parts sounds simple until you consider some of the anomalies that can arise. A road may be referred to by multiple names, such as “Route 101A” and “Amherst Street”. The same road name may be spelled in multiple ways, such as “Route 101A”, “Route 101-A”, or “Rte. 101A”, or “NH State Route 101A”.
If you add multiple countries, languages, and address formats to this picture, you can quickly see just how many variations might arise from a single physical address. Multiply that by the thousands of data elements that make up customer and transaction records, and it quickly becomes apparent just how important standardization can be for financial institutions. Standardization of data models is a necessary precursor to data consistency.
Availability
Good data governance ensures that vital business information is secure and that there is a clear audit trail to determine who accessed or changed information, and when. High profile security incidents have generated intense negative publicity for multiple companies.
However, data must also be available to the right people within the organization at the right time. As self-service applications such as web portals and mobile apps have gone mainstream, the challenge of ensuring availability of data alongside security has never been higher.
Trustworthiness
Ultimately, all of these quality requirements lead to the same endpoint; for financial institutions, trustworthiness is a non-negotiable requirement. With this as a priority, an aggressive data quality program should be a foregone conclusion. For financial services firms, it is imperative.
Good data quality practices dictate that business leaders work together to define clear outcomes. That includes cross-functional collaboration across multiple departments. It is impossible to govern everything, so subject matter experts from across the organization need to work together to establish a shared list of priorities around risk, compliance, finance, and marketing objectives.
Good data governance practices also imply a sound strategy for using technology to automate data quality. That includes the use of tools that help companies to cleanse, validate, de-duplicate, and standardize their critical data. Data Quality tools can detect problems of which personnel might not be aware, and then provide dashboards and automated workflows that help staff members to identify and resolve data quality problems quickly and easily.
Is your data quality all it could be? To learn more, read our eBook: Creating an Agile Data Quality Strategy for Effective Regulatory Compliance