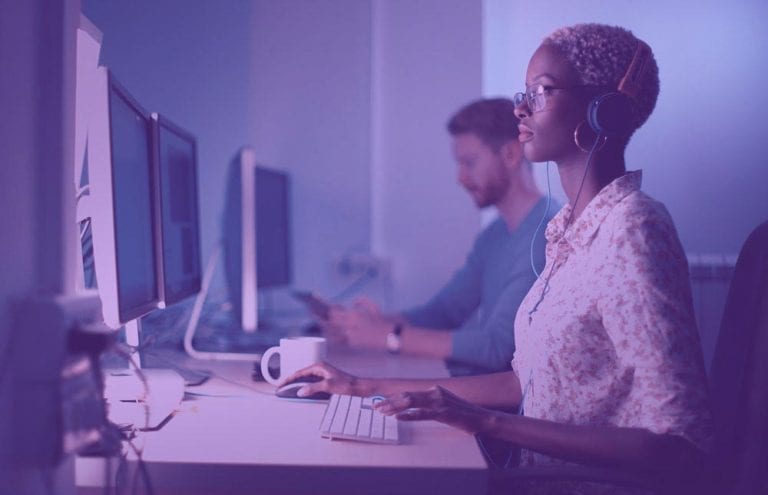
Your Data Governance Solution Essential to AI and Machine Learning
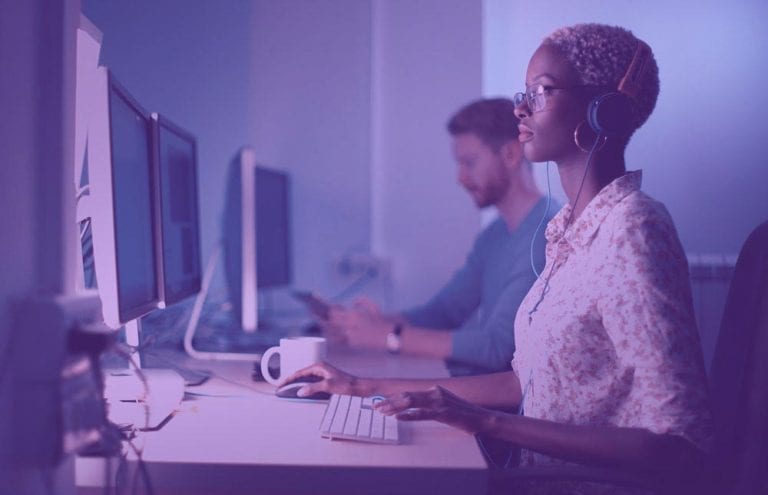
Enterprise use of Artificial Intelligence (AI) and machine learning is growing rapidly. A 2019 report by Gartner reveals that over the previous four years, the number of enterprises employing AI grew by 270 percent. It’s easy to understand why. With good data governance, AI and machine learning technologies provide an unprecedented ability to extract useful and actionable insights from corporate data.
AI and machine learning algorithms work by sensing hidden patterns in data that are often so subtle that human beings can’t even detect them, let alone understand or apply them. To accomplish this feat, AI algorithms must be “trained” using large amounts of pre-selected data with characteristics similar to the datasets of interest. In a sense, an AI algorithm effectively defines itself based on the training data to which it is exposed. For this reason, the effectiveness of an AI implementation is directly dependent on the quality of its data.
The danger of hidden biases in AI
A major issue for AI use arises when training or production data is not properly assessed and cleansed with respect to the potential for hidden biases.
Here’s a good example of how unintended biases can creep into seemingly objective data. The city of Boston distributed a smartphone app designed to help locate and repair potholes. What wasn’t initially taken into account was the fact that smartphone use was not evenly distributed across the city, but was much less among low-income and elderly inhabitants. As a result, until that oversight was corrected, those populations didn’t receive the level of services they should have.
As this example illustrates, unwary AI users may be vulnerable to making unwarranted predictions or taking inappropriate actions because of unsuspected biases in AI training or production data. That’s why it’s critical for companies making use of AI to have in place a comprehensive data governance regime that allows them to understand and, as necessary, correct the data used by their AI engines.
How Precisely Trillium enables good data governance for AI
Precisely Trillium gives you control over the quality of your data to ensure your data governance standards are met. It allows you to track the lineage of data from various sources that ends up in your corporate data lake or other repository. With that information, you can assess the accuracy and completeness of data based on insight into the parameters recorded (or left out) at the original source.
For example, completeness is an important dimension of data quality. In assessing the completeness of the information being ingested by your AI platform, it’s important to be able to trace particular datasets back to their original sources to verify not only that all relevant fields are populated, but as importantly, that all the fields required to fully represent the target use case are present. In other words, does the smartphone data fed into your pothole AI engine include information regarding the distribution of smartphones among various population groups? Without such scrutiny and cleansing of the data at its source, the introduction of unintended and unnoticed bias is highly probable.
Using Precisely Trillium for Data Governance allows you to evaluate the relationships between data from different sources, and to standardize and cleanse those data streams as necessary. By providing a comprehensive view of your data, Precisely Trillium gives you the tools you need to identify data that is inaccurate, incomplete, inconsistent, or duplicated and make needed corrections before that information is presented to your AI solution.
To learn more about how Precisely Trillium can help you put in place a comprehensive data governance regime for your AI and machine learning initiatives, read our white paper: Six Steps to Overcoming Data Pitfalls Impacting Your AI and Machine Learning Success.