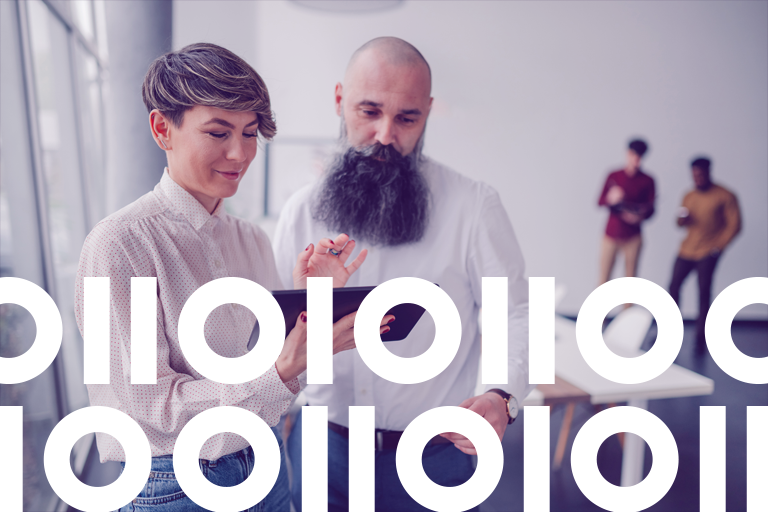
Mastering AI Data Observability: Top Trends and Best Practices for Data Leaders
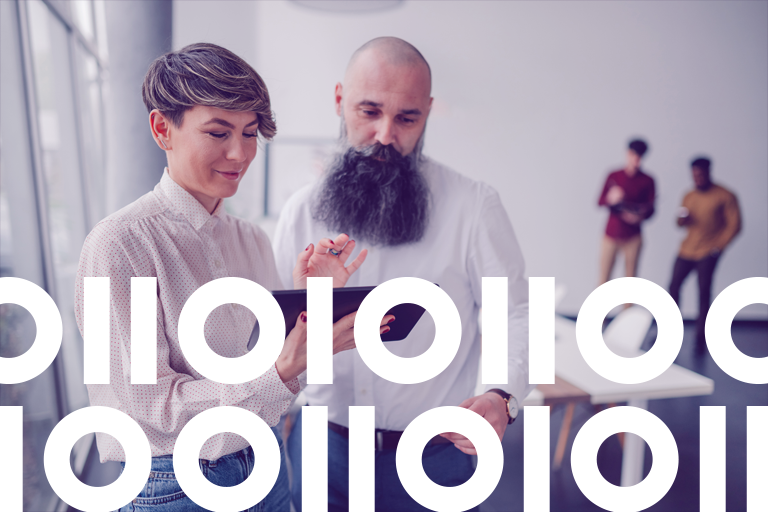
Key Takeaways:
- Observability is essential for trusted AI – Yet most organizations lack the structured programs, tools, and cross-team collaboration needed to make it effective.
- North America is pulling ahead – U.S. organizations show significantly higher observability maturity, trust in AI outputs, and use of diverse data types compared to Europe.
- Leaders must act now – Addressing skills gaps, investing in dedicated tools, and aligning governance practices are critical steps to ensure AI success and mitigate risk.
Artificial intelligence (AI) and machine learning (ML) are transforming businesses at an unprecedented pace. And yet, many data leaders struggle to trust their AI-driven insights due to poor data observability.
In fact, only 59% of organizations trust their AI/ML model inputs and outputs, according to the latest BARC Data Observability Survey: Observability for AI Innovation.
If you’re a data leader grappling with trust, transparency, and governance in AI data pipelines, you’re not alone. This survey of 264 data and AI stakeholders uncovers why organizations struggle with data observability – and what leading teams are doing to solve that problem head-on.
Let’s dive into some of the key findings from the survey. For more, be sure to get your copy of the full report.
Data Observability: A Critical Pillar of AI Success
Data observability isn’t just about monitoring systems – it’s about ensuring that data quality, data pipelines, and AI/ML models produce accurate, reliable insights. The survey found that:
✅ 76% of organizations have implemented formal observability programs for data quality and pipelines.
✅ Fewer organizations (though growing in number) have tackled AI/ML model observability, leading to trust issues in AI-generated insights.
✅ Generative AI (GenAI) adoption is accelerating, requiring new observability approaches for unstructured data, vector databases, and AI-generated content.
For AI to be effective, data leaders must establish strong observability practices that ensure high-quality inputs and transparent, accountable outputs.
Your Top Challenges – and How to Overcome Them
Despite increasing adoption, data observability remains one of the biggest obstacles to AI maturity. The survey pinpoints four core challenges that data leaders must tackle:
1️. The skills gap: lacking expertise in AI data observability
🔴 The #1 barrier to observability is the lack of trained professionals who understand how to monitor AI data pipelines and model performance.
🔴 Many organizations rely on manual processes due to a shortage of automation skills.
✅ How to fix it:
- Upskill your data teams with AI-specific observability training.
- Invest in tools that automate observability processes, reducing reliance on manual monitoring.
- Consider leveraging AI-powered observability assistants to improve efficiency.
2. AI data trust issues: can you trust your AI/ML models?
🔴 Only 59% of organizations trust their AI-generated outputs – a major problem for decision-making.
🔴 Data drift, model bias, and unstructured data quality remain unaddressed in many AI observability programs.
✅ How to fix it:
- Implement real-time monitoring for AI/ML pipelines to detect drift, bias, and anomalies before they impact decisions.
- Prioritize unstructured data observability – over 60% of organizations now recognize its role in AI success.
- Strengthen governance frameworks to ensure AI models operate with full transparency and accountability.
3. Tooling gaps: relying on legacy solutions instead of AI-focused observability tools
🔴 Most organizations still depend on traditional BI, data warehouses, and data integration tools for observability.
🔴 Only 8% use dedicated observability platforms, limiting automation and scalability.
✅ How to fix it:
- Move beyond basic monitoring tools and adopt dedicated AI observability platforms that provide:
✔️ Automated anomaly detection
✔️ AI model drift monitoring
✔️ Full lifecycle observability for structured & unstructured data - Avoid patchwork solutions – integrate observability directly into your AI governance strategy.
4️. AI observability maturity: North America leads, Europe lags behind
🔴 88% of North American organizations have formalized observability programs vs. only 47% in Europe.
🔴 North American firms also prioritize regulatory compliance and model accuracy more than their European counterparts.
✅ How to fix it:
- Benchmark your observability maturity: where do you fall on the spectrum?
- Focus on closing AI governance gaps to align with North America’s best practices.
- Establish clear KPIs for observability success, including model accuracy, data integrity, and compliance adherence.
Report
BARC Research Study: Observability for AI Innovation
This research study examines three distinct observability disciplines: data quality, data pipeline and AI/ML model. In each case observability refers to the measurement, monitoring and optimization of these elements.
The Rise of GenAI: How Observability Must Evolve
As GenAI adoption rises among companies, new requirements come into play that necessitate a modernized approach to observability.
AI observability is no longer just about structured data – modern AI models require observability across all data types, including:
🔹 Unstructured data (documents, images, video, audio)
🔹 Vector databases for GenAI applications
🔹 AI-generated content governance
Today, 40% of organizations already recognize that GenAI observability is a priority – is your organization one of them?
If so, there are some essential best practices you need to put into action:
🔹Foster AI trust through transparency: improve collaboration between data scientists, engineers, and business leaders.
🔹Ensure AI model governance: track data lineage and accuracy from input to output.
🔹Expand observability beyond structured data: monitor documents, streaming data, and vector embeddings.
Take Action: BARC’s Observability Playbook for Data Leaders
With all of this in mind, the report outlines key recommendations for those ready to advance in their data observability journey. Here’s a preview:
🔹Formalize and optimize observability programs
Move away from ad hoc monitoring – instead, implement structured programs covering data quality, pipeline integrity, and AI model trustworthiness.
🔹Strengthen data governance and compliance
Ensure observability aligns with privacy laws, auditability standards, and AI governance frameworks to maintain regulatory compliance.
🔹Establish metrics for success
Define quantitative and qualitative KPIs for measuring observability effectiveness – like model accuracy, bias detection, and data drift monitoring.
🔹Invest in AI-specific observability tools
Move beyond traditional BI and data integration platforms. Adopt solutions that offer automated AI model monitoring, root cause analysis, and cross-system observability.
🔹Strengthen skills and cross-team collaboration
AI success depends on multi-disciplinary collaboration. Establish structured workflows between data scientists, engineers, business process owners, and AI governance teams.
Are You Ready for the Future of AI Observability?
The BARC survey makes one thing clear: organizations that invest in AI data observability today will build more trustworthy, scalable, and successful AI systems in the future.
That means that data leaders who proactively address observability challenges – particularly around AI observability – will set their organizations apart from the competition in the long-term.
So, the question is: Are you leading, or lagging behind? Now is the time to elevate your observability strategy and ensure your AI models deliver real business value.
What’s your biggest AI observability challenge? Read the full Observability for AI Innovation report for an in-depth look at all of the impactful findings, and see how you can apply them to the obstacles you’re currently facing in your journey.
And, don’t hesitate to connect with our team to learn more – we’re here to be your partners in data observability success!