eBook
Bringing Insights to Life with AI and Data Enrichment
Introduction:
The Power of Data Enrichment
In today’s data-driven world, businesses generate vast amounts of raw data daily. However, data without context is like a puzzle with missing pieces—hard to understand and even harder to act upon. This is where data enrichment comes in, transforming scattered data into actionable insights by adding context, relevance, and depth. Whether it’s enhancing customer profiles, streamlining operations, or empowering AI models, enriched data brings clarity and precision to decision-making.
Discover how these technologies can elevate your organization’s ability to make informed, strategic decisions.
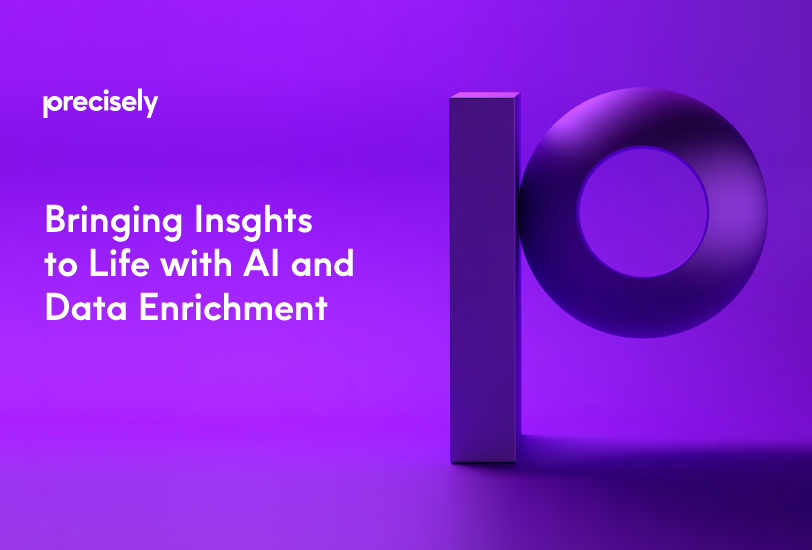
Chapter 1:
What is Data Enrichment?
Data enrichment is the process of enhancing raw data with additional information from external sources. It adds layers of context to help businesses better understand their data. This context could include demographic data, geographic location, business information, or environmental risks, allowing organizations to create more detailed profiles, optimize their operations, and make smarter decisions.
For example, a business may have a list of customer addresses. By enriching this list with demographic and purchasing data, it gains a clearer understanding of its customer base, enabling more targeted marketing efforts.
Chapter 2:
Real-World Examples of Data Enrichment
Improving Insurance Underwriting with Location Data
An insurance company wants to assess property risk when underwriting a new policy. With basic data, they can evaluate the property’s size, location, and owner. However, by enriching this information with geospatial data—such as flood zones, crime rates, and historical weather data—the insurer gains a more accurate risk profile.
With this enriched dataset, the company can offer more precise policy premiums, reducing the risk of underpricing or overpricing a policy. This is made possible through tools like the Data Graph API, which allows access to a variety of location-based datasets, integrating geospatial intelligence with internal data.
Enhancing Customer Profiles for Targeted Marketing
A retail company stores customer data such as names, addresses, and purchase histories. By enriching this with aggregate demographic data like—age, income levels, and interests in geographical zones—they can create more detailed customer personas. This enables them to launch hyper-targeted marketing campaigns that resonate with specific customer segments.
Optimizing Network Planning with Boundary Data
A telecommunications company is planning to expand its network, but its location data alone doesn’t enable it to conduct a sufficiently detailed analysis of where service area borders and infrastructure should be positioned. By enriching its location data with data that defines boundaries for key areas of interest, such as neighborhoods and metro areas, it’s able to ensure the expansion best captures local market demand, provides high-quality service, and deploys new infrastructure in a cost-effective manner.
Across industries and use cases, enrichment data provides a new layer of information that is critical to business decisions.
Chapter 3:
AI and Data Enrichment—A Perfect Match
Artificial intelligence (AI) relies on high-quality, well-structured data to deliver accurate predictions and insights. Data enrichment enhances the quality of input data, making AI systems more effective. By providing more complete datasets, enriched with meaningful information, AI can deliver results that are more precise, personalized, and actionable.
Consider an e-commerce company using AI to recommend products. If the customer data is enriched with details like shopping habits, geographic location, and purchasing power, the AI can offer personalized recommendations that resonate more with each individual, boosting customer engagement and sales.
Moreover, data enrichment can help to mitigate potential bias in AI systems by enhancing the comprehensiveness and diversity of model training data. With additional context provided by integrating trusted, third-party data, organizations can be confident that AI outputs are ready for real-world use and don’t reflect biases that commonly arise from using limited or siloed training datasets.
Chapter 4:
AI-Driven Insights with Enriched Data
When AI is applied to enriched datasets, the results are transformative. AI systems can quickly spot trends, correlations, and outliers that might otherwise be missed. For example, in urban planning, AI can analyze enriched data to predict population growth, traffic patterns, and infrastructure needs, helping city planners make more informed decisions.
Supply chain optimization is another compelling use case. Companies can enrich their supply chain data with external information about weather conditions, political events, or local road obstructions, enabling AI to forecast disruptions and suggest alternative routes or suppliers in real-time.
Chapter 5:
How to Implement Data Enrichment in Your Business
To leverage the full potential of enriched data, businesses must integrate it into their workflows and AI models effectively. The following is a practical, simple roadmap for streamlining data enrichment and unlocking the value it provides:
Identify Data Gaps: Start by analyzing your existing datasets to determine what’s missing. Are there opportunities to add demographic, geographic, or market data?
Choose the Right Data Provider: Select a provider that offers high-quality, curated datasets relevant to your industry. Precisely, for example, offers the Data Integrity Suite, which includes tools to enrich data with precise geospatial and demographic information.
Use APIs for Seamless Integration: APIs like the Data Graph API enable businesses to enrich their data efficiently. They allow you to pull in relevant data on-demand, without the need to store massive datasets locally.
Incorporate AI for Deeper Insights: Once your data is enriched, integrate AI tools to unlock predictive analytics and pattern recognition. This will help your business make more informed, proactive decisions.
Chapter 6:
The Future of Data Enrichment and AI
As AI and data enrichment technologies continue to evolve, their potential applications will only expand. Future advancements will focus on creating more automated and contextually aware systems, where data is enriched and processed in real-time to provide instant insights.
For instance, smart cities will use AI and enriched geospatial data to improve everything from traffic management to emergency responses. Healthcare providers will enhance patient records with environmental and behavioral data, leading to more personalized care.
The combination of AI and data enrichment holds the key to unlocking a more intelligent, connected world where businesses can thrive on data-driven decisions.
Conclusion:
Bringing Insights to Life
Data enrichment is a critical process for transforming raw data into valuable insights. Combined with AI, enriched data helps businesses gain a competitive edge by enabling smarter decisions, better customer experiences, and more efficient operations.
By embracing these technologies, organizations can bring their data to life, using it not just as a historical record but as a predictive tool that drives innovation and growth. Now is the time to explore the possibilities of data enrichment and AI to unlock the full potential of your data.
This eBook offers just a glimpse of the potential that enriched data holds. For businesses ready to dive deeper, the Precisely Data Integrity Suite provides a comprehensive solution to start precturning raw data into actionable insights today.