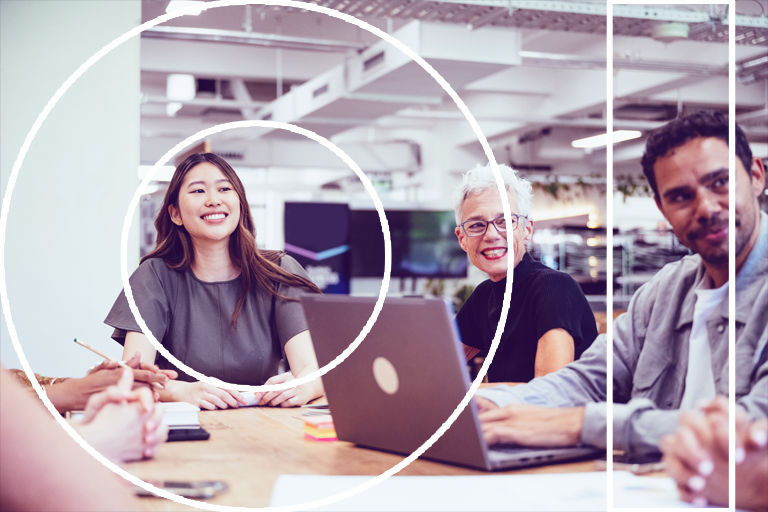
Mainframe Data Meets AI: Reducing Bias and Enhancing Predictive Power
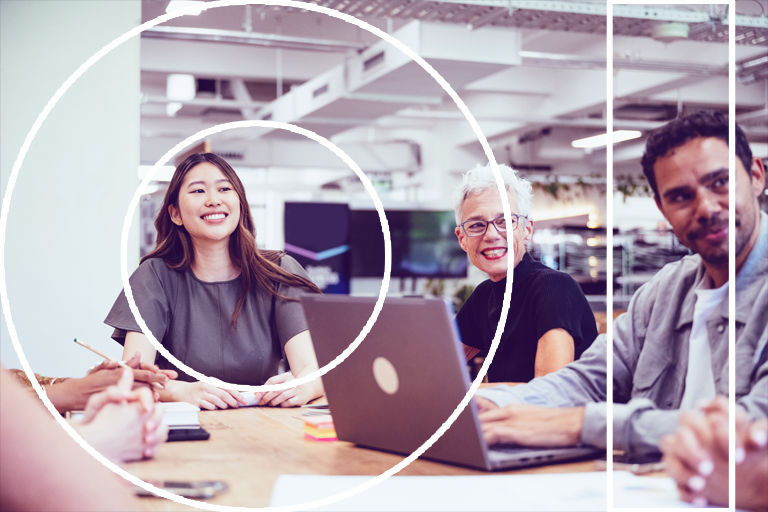
Key Takeaways:
- The significance of using legacy systems like mainframes in modern AI.
- How mainframe data helps reduce bias in AI models.
- The challenges and solutions involved in integrating legacy data with modern AI systems.
- The potential benefits of these integrations.
In today’s rapidly evolving technological landscape, businesses across industries are constantly looking for ways to harness the power of artificial intelligence (AI) to drive better decision-making, enhance customer experiences, and create efficiencies in operations. However, as with any innovation, there are significant challenges to be addressed. One critical issue is bias in AI models, which can lead to flawed outcomes and perpetuate existing inequities. Interestingly, a key solution to this problem lies in a rather unexpected place: the mainframe.
This article explores how organizations can tap into their mainframes to enhance their AI outcomes, eliminate biases, and keep up with the demands of the future.
The Importance of Mainframe Data in the AI Landscape
For decades, mainframes have been the backbone of enterprise IT systems, especially in industries such as banking, insurance, healthcare, and government. These systems store massive amounts of historical data—data that has been accumulated, processed, and secured over decades of operation.
Mainframe data is not just large in volume; it is also rich in context, containing a wide variety of transactional, demographic, and behavioral information that can provide invaluable insights when used effectively.
However, the rise of cloud computing and AI has prompted many organizations to question the role of mainframes in a modern tech stack. Yet, despite the perception that mainframes are outdated, they continue to play a critical role in business operations due to their robustness, reliability, and ability to process vast amounts of data efficiently.
When integrated with AI, mainframe data has the potential to serve as a key asset in addressing one of the most persistent challenges in AI—bias.
Understanding Bias in AI
Bias in AI arises when the data used to train machine learning models reflects historical inequalities, stereotypes, or inaccuracies. This bias can be introduced at various stages of the AI development process, from data collection to algorithm design, and it can have far-reaching consequences. AI models that are biased can produce skewed results that disproportionately affect certain groups of people, whether based on race, gender, socioeconomic status, or other factors.
For example, a biased AI algorithm used in hiring might favor certain demographics over others, perpetuating inequalities in employment opportunities. Similarly, biased AI in financial services might lead to unfair loan approvals or denials. Addressing this bias is critical for ensuring that AI models produce fair, ethical, and reliable outcomes.
One of the root causes of bias in AI is the limited and incomplete data sets used to train models. In many cases, organizations rely on recent, readily available data that may not accurately reflect the full diversity of experiences or historical context needed to make informed decisions. This is where mainframe data can make a transformative impact.
Read the eBook
Preparing Your Data for AI with Data Integration
Read our eBook which provides a check list and quick overview of the key steps and considerations for data integration.
How Mainframe Data Helps Eliminate AI Bias
Mainframe data is often more comprehensive and diverse than newer data sources. It reflects a wider range of experiences, spanning long periods of time and covering a broad spectrum of transactions and interactions. By incorporating this data into AI models, organizations can reduce the risk of bias and create more accurate and representative algorithms.
Here are several ways mainframe data can help eliminate bias in AI:
1. Diverse and Rich Historical Data
Mainframes store decades’ worth of transactional data. This data captures historical trends and behaviors across different demographics, markets, and socioeconomic conditions. By training AI models on such a broad dataset, organizations can create more balanced models that account for a variety of outcomes rather than reinforcing recent trends that might be biased.
2. Inclusion of Underrepresented Groups
Bias in AI often results from the underrepresentation of certain groups in the training data. Mainframes, particularly in sectors like finance or healthcare, contain detailed records that include a diverse range of customers or clients, from different ethnic backgrounds, age groups, and income brackets. By including this historical data, organizations can ensure that these groups are better represented in their AI models, resulting in more equitable outcomes.
3. Contextual Insights
Historical data from mainframes provides context that is often missing in newer datasets. For instance, a mainframe system might contain data on how financial markets behaved during past economic downturns, or how consumer behavior shifted in response to specific regulatory changes. This type of context is invaluable when training AI models to avoid oversimplifying patterns or making decisions based on recent data that could be skewed.
4. Correcting Imbalances in Real-Time Data
Real-time data can sometimes introduce biases because it reflects current societal inequities or short-term trends. Mainframe data, which encompasses long-term trends and stable historical data, can help correct these imbalances. By using historical data as a baseline, AI systems can better understand the long-term behaviors and patterns that should inform decision-making, reducing the reliance on skewed real-time data.
Challenges in Integrating Mainframe Data with AI Systems
While the potential for using mainframe data to reduce AI bias is significant, it is not without challenges. One of the primary hurdles is the complexity of integrating legacy systems with modern AI frameworks.
Mainframes were not designed to interact with AI systems that rely on cloud infrastructure and modern data processing techniques. As a result, organizations often face difficulties in extracting, cleaning, and transforming mainframe data into a format that can be used by AI algorithms. Additionally, mainframe data can be stored in proprietary formats that require specialized knowledge to access and interpret.
Here are a few common challenges organizations encounter when integrating mainframe data with AI systems:
1. Data Silos
Mainframe data often exists in a silo, separated from other enterprise data. This can make it difficult to integrate with modern AI systems that rely on a centralized data platform. Overcoming this challenge requires a comprehensive data integration strategy that brings together data from different sources into a unified platform.
2. Data Formatting and Compatibility
Mainframe data may be stored in legacy formats that are incompatible with modern AI tools. This requires data transformation processes that can convert the data into usable formats. Additionally, organizations may need to address issues such as data duplication, inconsistency, and quality, which can arise when working with legacy systems.
3. Security and Compliance Concerns
Mainframes are often used to store sensitive data, especially in regulated industries such as healthcare and finance. When extracting and using this data for AI purposes, organizations need to ensure they are compliant with data privacy regulations such as GDPR or HIPAA. Implementing secure data transfer processes and ensuring that AI models do not inadvertently expose sensitive information are critical considerations.
4. Cost and Resource Constraints
Maintaining mainframes and integrating them with AI systems can be expensive and resource intensive. Organizations need to invest in infrastructure, tools, and talent to effectively manage the integration process. However, the long-term benefits of leveraging mainframe data for AI—such as improved decision-making, reduced bias, and enhanced competitiveness—often justify the investment.
Solutions for Seamless Integration of Mainframe Data
Despite the challenges, there are several strategies and solutions that can help organizations successfully integrate mainframe data with AI systems:
1. Mainframe Modernization
Many organizations are modernizing their mainframes by moving them to the cloud or adopting hybrid cloud solutions. This not only extends the life of their legacy systems but also makes it easier to integrate with modern AI frameworks. By leveraging cloud-based mainframe environments, organizations can ensure that their mainframe data is accessible to AI systems and other enterprise applications.
2. Data Virtualization
Data virtualization tools enable organizations to access and analyze mainframe data without physically moving it. These tools create a virtual layer that allows AI systems to query mainframe data in real-time, without the need for extensive data migration processes. This approach reduces the complexity and cost associated with data integration.
3. API Integration
Organizations can use APIs (Application Programming Interfaces) to create connections between mainframes and AI platforms. APIs allow different systems to communicate with each other, enabling real-time data sharing and integration. This approach can simplify the process of extracting and using mainframe data for AI model training.
The Future of Mainframe Data in AI
As AI continues to advance and become more embedded in critical decision-making processes, the importance of reducing bias and ensuring fair outcomes will only grow. Mainframe data, with its rich historical context and diverse demographic representation, will play an increasingly important role in this effort.
By overcoming the challenges of integrating legacy systems with modern AI, organizations can unlock the full potential of their mainframe data. This not only helps to eliminate bias in AI models but also enables businesses to create more accurate, equitable, and trustworthy AI systems.
Read our eBook Preparing Your Data for AI with Data Integration which provides a check list and quick overview of the key steps and considerations for data integration.