eBook
Unlocking AI Success through Data Integration - Transform your AI initiatives
Introduction
AI is fundamentally transforming businesses across industries. However, for AI to deliver benefits such as accurate and actionable insights, cost savings, and improved customer service, the data it uses must be complete, accessible, and integrated.
When AI training models are given inconsistent, biased, redundant, incomplete, outdated, or inaccurate data, it will produce incorrect results. Those results can expose the business to risk, damage customer relationships, delay decision-making, and decrease revenue.
For all the potential it holds, AI is the ultimate garbage in / garbage out analogy. As soon as an AI model is fed incomplete data, the damage is done; you’ll simply get wrong answers faster. However, when AI models are fed enterprise data, businesses can enjoy the benefits AI has to offer, including improved efficiencies, customer satisfaction, cost savings, and improved regulatory compliance.
Data integration is a keystone of success with AI. By consolidating data from diverse sources and formats into a unified view, organizations can seamlessly access and analyze their data, regardless of its origin or structure. This process also ensures that the data is accurate, complete, and reliable – key attributes of enterprise data necessary for successful AI implementations.
There’s no one solution for data integration, and different AI use cases may require different approaches. Let’s look at some of the challenges data integration for AI can help businesses solve and some of the use cases where data integration can help an organization succeed in its AI initiatives.
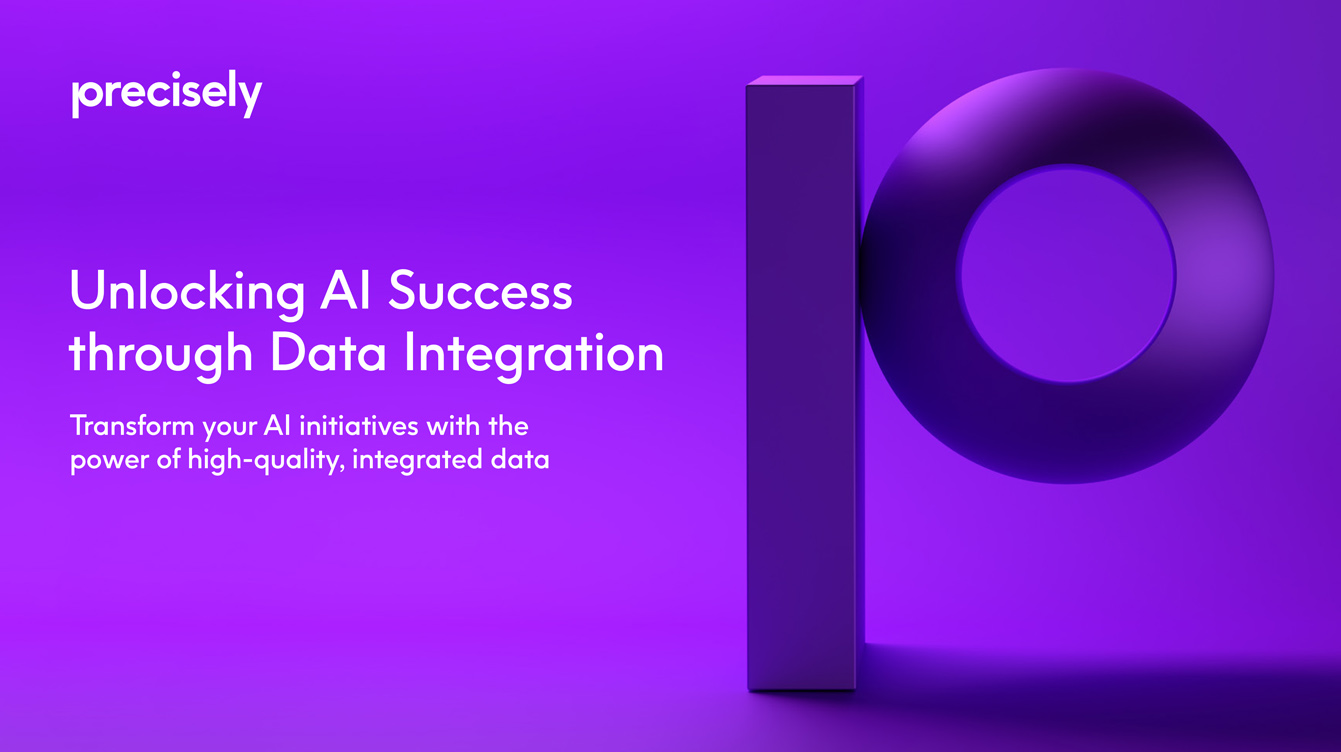
Business Challenge:
Slow Decisions in a Fast Market
In today’s rapidly changing markets, if a business can’t use predictive analytics, it risks falling behind competitors who can. Delayed decision making can cause missed opportunities, slow responses to market shifts, and an inability to capitalize on emerging trends.
Solution
Data integration consolidates data stored in different formats and environments throughout the organization and unifies it into a consolidated view that AI models can use.
When AI models have access to comprehensive data, they can produce reliable real-time insights and predictions, automate decisions, foster innovations that lead to new products and services, and help businesses adapt quickly to emerging trends.
Business Challenge:
Unreliable AI Results
Advanced AI models are ineffective without reliable data, and the stakes are high when results are wrong. Basing decisions on incorrect AI outcomes can damage customer relationships, expose the business to risk and penalties of non-compliance, and waste money and time. Using inaccurate predictions and recommendations can also damage internal trust and obstruct further adoption of AI technologies.
Solution
Data integration that ensures the most comprehensive datasets are available for use in AI initiatives allows organizations to fully leverage the power of all the valuable data they’ve collected and saved. When AI uses high-quality, integrated data, it can make decisions on a full spectrum of correct and relevant information, which leads to reliable decision-making.
Business Challenge:
Pressure to Reduce Costs and Increase Efficiencies
As competition intensifies in a global market, businesses face pressure to reduce costs and improve efficiency. To stay competitive, they must find innovative ways to streamline operations, cut expenses, and optimize resources. AI can help by automating routine and time-consuming tasks, identifying inefficiencies and negative patterns, and flagging anomalies that may lead to expensive mistakes.
Solution
Businesses can reduce costs and improve efficiencies by building data integration pipelines that inform AI outcomes. Companies using integrated data to feed AI models can identify previously undiscovered inefficiencies and redundancies across business functions. When data is effectively integrated, AI models have the information needed to streamline workflows, reduce manual tasks, minimize errors, and deliver more precise insights for forecasting and data-driven decisions.
Use Cases
Integrated data allows AI models to deliver powerful insights across industries. Let’s look at some common scenarios.
When given integrated data from a variety of sources, AI processes can deliver faster, more personalized recommendations, enhance customer experience, improve retention, and increase revenue.
For example, companies might use data integration to combine customer browsing, purchase history, and product reviews to create AI-tailored recommendations for retailers. Streaming services might use data integration to combine viewing history, rating, and user preferences to intelligently suggest what to watch, read, or listen to next.
Before using AI for personalized recommendations, an organization’s data must be integrated, accurate, complete, and reliable. Data can provide valuable behavioral information so businesses can better understand their customers and quickly identify emerging trends so businesses can adjust strategies and offerings.
AI models can quickly analyze vast amounts of data to determine patterns or anomalies that indicate fraud, even in real time. Intelligent processes can significantly reduce financial losses, prevent or alert about unauthorized access, and reduce costs associated with manual fraud detection. When a business can be proactive instead of reactive in its approach to fraud detection, it protects company assets and compliance and boosts consumer trust.
When AI can use aggregated transaction data, behavioral patterns, and external data sources to identify and prevent fraudulent activities, subtle anomalies, and fraud patterns can be difficult or impossible to identify when data is siloed. Data integration enhances fraud detection and prevention by consolidating data in multiple formats
and from various sources into a unified source for AI consumption and real-time monitoring.
As organizations look for ways to reduce costs and risks, they face the problem of aging technology that still provides relevant data to those systems. AI and data integration can reduce the need for direct interaction between organization members and the technology, allowing the platforms to operate independently and concentrate on their intended functions. Meanwhile, the data generated by these systems can be utilized by other teams, uncovering insights that might otherwise be overlooked.
With data integration, data from legacy systems can be accessed and used to build more intelligence in the AI output for more comprehensive business intelligence (BI). With real-time or near-real-time BI, organizations can see a holistic view, identify problems and anomalies, and improve forecasting.
AI can help businesses reduce costs by automating time-consuming tasks such as handling routine customer inquiries. Chatbots reduce the need for a large customer service team and can handle many interactions simultaneously, improving response times. Chatbots can also provide consistent answers to frequently asked questions without ongoing training.
To be useful, AI models behind chatbots must be trained on large volumes of integrated data such as complete customer profiles, purchase histories, previous interactions, and support tickets. Integrated data ensures chatbots have the latest information to adapt in real-time, ensuring the interaction is relevant and helpful.
Summary
Data integration is pivotal in enhancing AI’s effectiveness in addressing modern business challenges. By consolidating information from multiple sources into a unified view, data integration empowers AI systems to deliver timely and accurate insights essential for making fast decisions in an ever-changing market.
By ensuring data is complete, consistent, and accurate, data integration improves the reliability of AI results, reducing the risk of errors and biases. It also plays a crucial role in reducing costs and risks, optimizing resources, automating processes, and enabling predictive analysis.
Integrated data enhances AI’s ability to provide personalized recommendations and improve customer service by offering a comprehensive view of customer interactions and preferences. Data integration enables AI to identify anomalies and patterns in real time for fraud detection and prevention and supports the modernization of IT systems by streamlining operations and facilitating the adoption of advanced technologies.