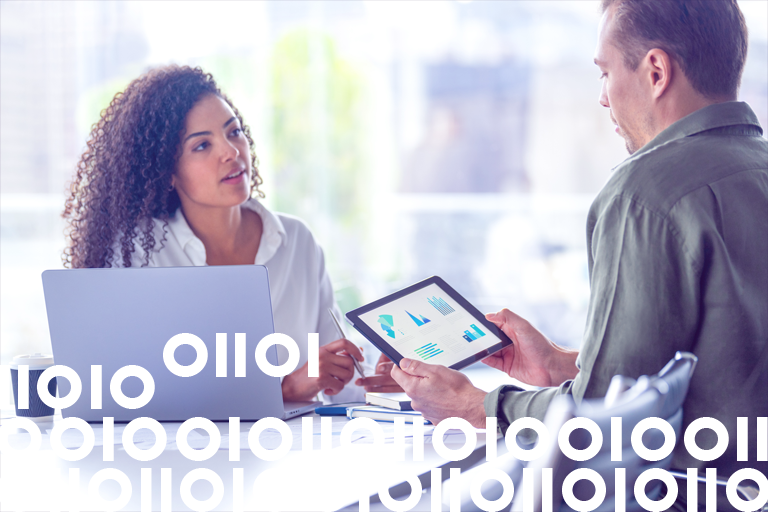
AI Success – Powered by Data Governance and Quality
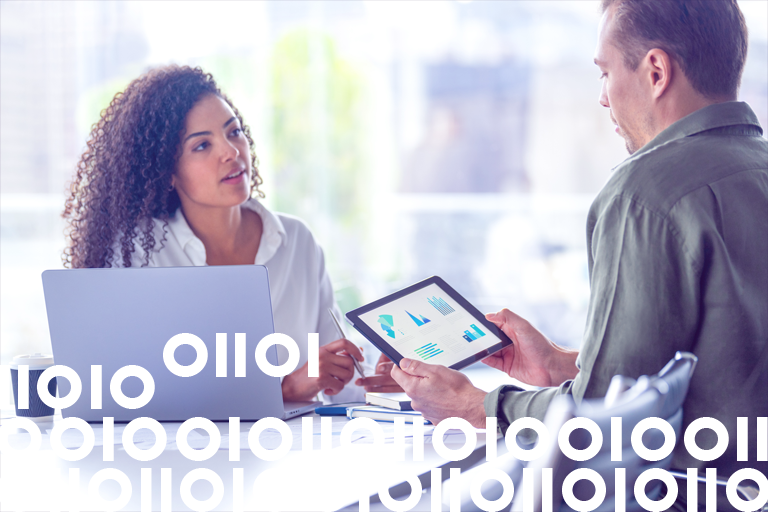
Key Takeaways:
- Data integrity is essential for AI success and reliability – helping you prevent harmful biases and inaccuracies in AI models.
- Robust data governance for AI ensures data privacy, compliance, and ethical AI use.
- Proactive data quality measures are critical, especially in AI applications. Using AI systems to analyze and improve data quality both benefits and contributes to the generation of high-quality data.
Everyone’s eager to unlock the potential of artificial intelligence (AI) in their business – but the question you need to answer first is: is your data ready?
There’s no question about the unprecedented opportunities that AI presents for innovation and competitive advantage. But achieving AI mastery comes with its own unique hurdles – the most significant being ensuring the quality and governance of the data that fuels your AI systems.
Precisely experts gathered for a panel hosted by Dataversity to discuss this topic more in-depth. The session featured:
- Ana-Maria Badulescu, Senior Director, AI Lab
- Sachin Bapat, VP of Value Engineering and Business Architecture
- Matt Vandevere, VP of Strategic Services
Watch the full session for all their insights into why robust data quality and governance frameworks are crucial for reliable AI outcomes, and how you can transform your data into a powerful asset for AI success.
Let’s explore some of the biggest takeaways.
The Importance of Data Integrity for AI
Data integrity – data with maximum accuracy, consistency, and context – forms the backbone of trustworthy AI models. When data integrity is compromised, AI outputs can be biased, inaccurate, or even harmful.
In practical terms, data integrity ensures that the data feeding AI systems is complete, trusted, and contextual. For AI to deliver reliable results, the underlying data must be high quality. This means addressing common issues including data inaccuracies, inconsistencies, and lack of relevant context that can skew AI outputs.
“AI can only produce an answer as good as the data it can consume in order to render a result,” says Matt Vandevere, VP of Strategic Services at Precisely. “Therefore, because of the potential risk involved in acting on a flawed AI result, organizations need a robust data management program to ensure that the data being leveraged by the AI models is as good as it can be.”
Read eBook
Trusted Data, Powerful AI: Driving Better AI Outcomes through Data Quality and Governance
In this eBook, you’ll learn that when AI models are given quality data, businesses enjoy increased efficiencies, cost savings, improved regulatory compliance, customer engagement and satisfaction, and reduced output bias.
Challenges in Ensuring Data Quality for AI
Data quality is an ongoing challenge for organizations. Traditional approaches to data quality are reactive, addressing issues as they arise. But in the AI context, proactive data quality management is essential.
Ana-Maria Badulescu, Senior Director of AI Lab at Precisely, emphasizes, “A key data challenge for data quality is transitioning from being reactive to having a proactive approach … at scale, especially given the growing volumes of data like never before. AI can help you, AI is not just the beneficiary of high-quality data, it’s also the contributor and the producer of high-quality data.”
Badulescu cites two examples:
- Quality rule recommendations: AI systems can analyze existing data to understand data ranges, anomalies, relationships, and more. Then, this information can be used to suggest new quality rules that will help prevent data issues proactively.
- Data observability: Continuously monitoring your data using AI-driven solutions to track data quality metrics and provide real-time feedback. This ensures that data remains accurate, consistent, and up to date – which prevents data issues from impacting downstream AI applications and maintains the performance of those applications over time.
The Role of Data Governance in AI
“It’s imperative that in this world of GenAI especially, and AI broadly, data strategy should be at the forefront of executive’s minds and in the execution plan of company strategy,” says Sachin Bapat, VP of Value Engineering and Business Architecture at Precisely.
And within those data strategies, data governance is non-negotiable. Badulescu adds, “Data governance has to be thought of as being embedded in the entire development lifecycle of AI systems from day one; it cannot be an afterthought.”
It’s important to note that in the AI age, your data governance practices must evolve ensure the ethical use, privacy, and compliance of these technologies. Let’s examine these measures more closely:
- Data privacy: Implement strict access control policies and mask personally identifiable information (PII) data to protect sensitive information. Ensuring data privacy involves using automated tools to detect and secure PII.
- Compliance: Review legal agreements on data usage and address intellectual property concerns with generative artificial intelligence (GenAI) outputs. Compliance measures also involve security risk assessments to identify potential gaps and ensure data isn’t compromised.
- Ethical AI: Establish an AI ethics strategy to document data and model governance, and ensure company-wide awareness and education on AI ethics. Ethical AI practices include transparency in data and model management, as well as involving diverse teams to oversee ethical considerations.
Vandevere adds that data catalogs also have an important role to play: “Establishing your data catalog along with the classification to that catalog is critical. Understanding what data you’re getting from a lineage perspective and where it’s coming from – how are the handoffs being managed? How is the transformation being understood?
All of that is critical to understand that you are treating the data ethically and that you’re actually presenting to the AI model a dataset that is fit for purpose.”
Overcoming Bias in AI
AI bias can have significant negative impacts across various sectors. For example, healthcare AI systems may produce biased diagnostics if training data underrepresents minority groups; search engine biases can reinforce job role gender bias – showing higher-level positions to males more than females.
So how do you avoid these harmful challenges?
“To minimize bias, you need to ensure a balanced, diverse, and representative training dataset,” explains Budalescu. “Essentially, you want to ensure that the dataset you’re using accurately reflects the real-world scenario that you’re trying to solve.”
To mitigate bias, organizations must take steps to ensure data quality and data governance:
- Data profiling is a data quality capability that helps you gain insight into the data select appropriate data subsets for training. Ensure training date sets are balanced diverse and representative to minimize bias.
- Data discoverability is a key part of data governance. You need to curate metadata with descriptive names and relationships to make data easily searchable and usable to ensure the best data is included in your AI model.
“Bias is a very critical topic in AI,” notes Bapat. “Being aware and fixing this ahead of time is critical to using these systems for treatment.”
Real-World Examples of Data Quality and Governance in AI
High-quality data and strong data governance can lead to remarkable AI outcomes – and we’re seeing those results firsthand. Here are just two examples of those success stories from Precisely customers:
- A mortgage financing company increased revenue by $7 billion by taking steps to clean and govern their data to feed their machine learning models. The company used data quality solutions to standardize, verify, and geocode their addresses and gain a better understanding of the demographics and geographies they market to.
- A national financial services company reduced the time to prep data for AI models from 13 hours to 3.2 hours by implementing a robust data governance and quality strategy.
“Doing data governance and data quality right can have a significant impact on businesses and their efficacy,” remarks Bapat.
Future Trends in Data Governance for AI
Looking ahead, data governance practices must evolve to keep pace with AI advancements. Key trends include:
- Certified, fit-for-purpose datasets: shifting focus from curating only critical data elements to certifying complete datasets for clearly defined purposes, ensuring thorough data lineage and quality measures are in place. Understanding the transformations data undergoes and the quality and enrichment steps applied is essential before leveraging datasets in AI models.
- Metadata curation: turning raw data into knowledge through meaningful names, descriptions, and relationships.
- Automation in governance: leveraging AI-assisted features to scale data curation and governance efforts. Automation tools can help manage large volumes of data, ensuring it remains accurate, consistent, and up to date.
Data-Driven AI Success Awaits
Data quality and governance are crucial for mastering AI. As businesses continue to adopt AI, a solid data strategy will be essential to achieving accurate, ethical, and impactful outcomes – and prioritizing data integrity, implementing robust governance practices, and proactively addressing data quality challenges is what will help you maximize your results.
Empower your AI initiatives by prioritizing data quality and governance. For more on the foundational elements of trusted AI, the top challenges, and how to overcome them, read our eBook Trusted Data, Powerful AI: Driving Better AI Outcomes Through Data Quality and Governance.