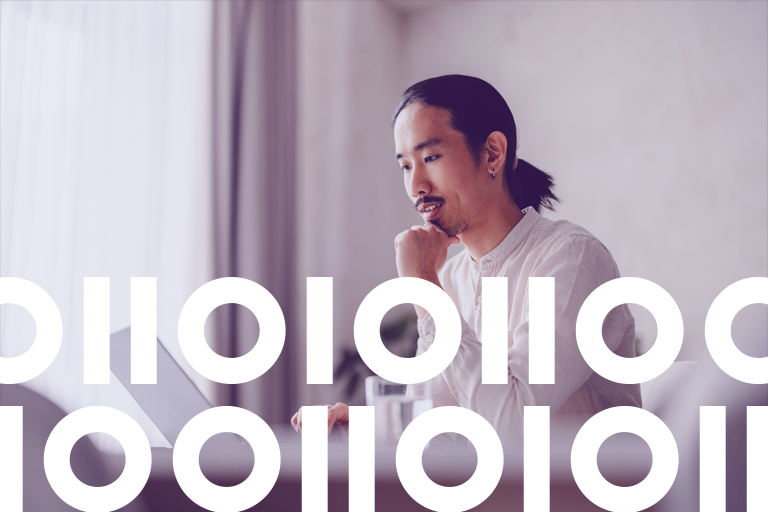
Modern Data Management Essentials: Exploring Data Fabric
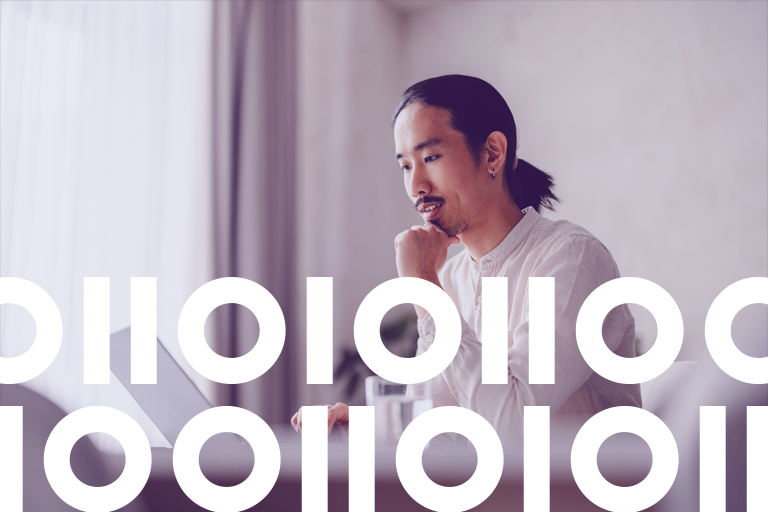
Key Takeaways
- Data Fabric is a modern data architecture that facilitates seamless data access, sharing, and management across an organization.
- Data management recommendations and data products emerge dynamically from the fabric through automation, activation, and AI/ML analysis of metadata.
- While data fabric is not a standalone solution, critical capabilities that you can address today to prepare for a data fabric include automated data integration, metadata management, centralized data governance, and self-service access by consumers.
As data grows exponentially, so do the complexities of managing and leveraging it to fuel AI and analytics.
In most organizations, data is accumulated in various systems and formats, leading to data isolation and inaccessibility. This hinders efficient data access and management and impedes actionable insights.
And when businesses face significant challenges in ensuring data consistency, security, and compliance across their diverse data landscapes, it often results in delayed decision-making and missed opportunities.
The untapped potential in this ocean of data is undeniable, yet most organizations don’t have a strategy to unlock its full value. A data fabric architecture is one solution that helps you meet these challenges head-on.
What is Data Fabric?
Data fabric is a modern data architecture that facilitates seamless data access, sharing, and management across your organization. Data fabric isn’t a stand-alone solution, but components that work together to deliver data management recommendations.
Using a combination of data integration techniques, metadata management practices, and other specialized data management tools, recommendations and data products emerge dynamically from the fabric through automation, activation, and AI/ML analysis of metadata.
A data product is a reusable set of data, metadata, semantics, and templates that are curated and maintained to be immediately useful for specific business objectives.
A data fabric offers several key benefits that transform your data management:
- Accelerates analytics and decision-making processes by enhancing data accessibility through seamless data integration and retrieval across diverse environments.
- Ensures consistent, high-quality data is readily available to foster innovation and enable you to drive competitive advantage in your markets through advanced analytics and machine learning.
- Significantly improves data governance and security through a unified framework for managing data policies, compliance, and quality across all data points.
2025 Outlook: Essential Data Integrity Insights
What’s trending in trusted data and AI readiness for 2025? The results are in!
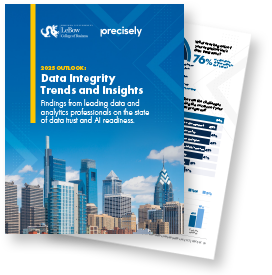
Data Fabric Challenges
A data fabric enables you to respond more quickly to market changes and customer needs, fostering greater business agility.
However, Gartner reports that less than 10-15% of organizations have the metadata maturity to implement an architecture successfully.
Let’s look at some of the challenges that must be addressed to accelerate the success of a data fabric architecture:
Lack of automated data integration
To build a modern data fabric architecture, you need access to data from across your landscape, including data from on-premises legacy systems and data stored in a logical data warehouse or data lake.
Without access to all critical and relevant data, the data that emerges from a data fabric will have gaps that delay business insights required to innovate, mitigate risk, or improve operational efficiencies.
Lack of metadata maturity
Metadata is how humans talk to machines and how machines talk to machines. Every time data is touched, it creates more metadata, making it difficult to manage in a timely and comprehensive fashion across a complex data ecosystem.
Unlike traditional metadata, which typically describes static attributes and properties of data (such as data type, size, and creation date), active metadata goes further by actively participating in and influencing the behavior of data management processes and workflows – providing real-time insights and influencing operational decisions.
Without an automated and AI-driven approach to harvesting, profiling, tagging, and observing metadata, metadata activation will yield incomplete, out-of-date, or incorrect data recommendations – leading to a lack of trust by data consumers and a lack of adoption and confidence in the approach itself.
Lack of governance
Because it’s driven by metadata, it can be difficult to implement a data fabric successfully without a business-friendly framework that defines meaning, ownership, and responsibilities, and clearly identifies data policies and processes.
Without this understanding, responsibility is unclear for ‘human in the loop’ processes related to the health, quality, and maintenance of the data in the data fabric. To avoid the risk of exposing sensitive data or violating regulations or agreements that would be costly and damaging to your company, you must closely monitor data usage and compliance.
Lack of self-service access to data
A data fabric facilitates comprehensive, consistent data access across your organization – regardless of where the data resides. This satisfies the needs of data owners, who require a simple way to make data products available to users and keep them up to date, and data users who demand user-friendly, self-service methods for finding and accessing trusted data.
Preparing for Data Fabric Success
To achieve the level of data integrity required for a successful data fabric architecture, you must comprehensively assess your current data landscape.
Here are four aspects of a data management approach that you should consider to increase the success of an architecture:
- Break down data silos by automating the integration of essential data – from legacy mainframes and midrange systems, databases, apps, and more – into your logical data warehouse or data lake.
- Increase metadata maturity. You must be able to continuously catalog, profile, and identify the most frequently used data. Implementing data observability tools that use machine learning intelligence and proactive alerts to inform you of metadata changes that could impact data insights or outcomes is essential.
- Establish robust data governance policies and practices to ensure data quality, security, and compliance. You must establish visibility, ownership, and accountability around data access, policies, quality, and advanced business metadata to ensure that your data is accurate, consistent, and fit for purpose.
- Create a user-friendly way to make data and data products available to users and keep them up to date. And if a goal of your data fabric is to support the creation of relevant data products, users must have an easy way to find trusted data for actionable insights and data-driven decision-making.
With these critical capabilities in place, you are on the path towards a successful data fabric architecture that fosters a data-centric culture in your organization and enables them to drive greater business value.
Precisely the global leader in data integrity, in partnership with the Center for Applied AI and Business Analytics at Drexel University’s LeBow College of Business, surveyed 565 data and analytics professionals on their organizations’ data strategies, priorities, challenges and the state of data trust and AI readiness. The results are in, read the report today! 2025 Outlook: Essential Data Integrity Insights