ebook
Trusted AI 101:
Tips for Getting Your Data AI-Ready
Successful AI initiatives rely on trusted data
With competition fiercer than ever, it’s easy to understand why businesses are racing to harness the power of artificial intelligence (AI) to increase productivity and efficiency, attract and retain customers with personalized service, and create new ideas that provide a competitive edge.
However, there are risks to rushing into AI without the proper preparations. In 2023, we saw AI failures ranging from AI-written briefs containing fake citations, to renowned consulting firms implicated in non-existent scandals, and many others.
In each case, the model that produced the bogus results had inadequate training data for the intended purpose, leading to skewed and flawed outputs, underscoring the need for AI powered by trusted data.
And trusted data requires data integrity – or data with maximum accuracy, consistency, and context. Think of it this way: your AI outputs will only be as strong as the data feeding them.
However, organizations often face data integrity challenges, like struggling to integrate data fast enough, understand and govern its responsible use, observe and improve its quality, enrich it for deeper context, and guarantee security and privacy.
The benefits are limitless if your AI applications learn from trusted, AI-ready data. And yet, the current reality for many organizations is sobering.
In the 2023 Gartner IT Symposium Research Super Focus Group, only 4% say their data is AI-ready.
With all this in mind, let’s explore valuable AI use cases and the data integrity fundamentals you need to ensure trust and success in your results.
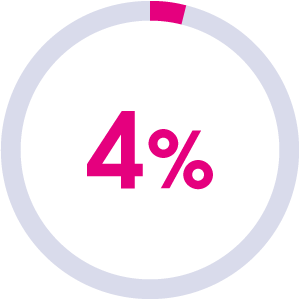
The sobering reality:
Only 4% say their data is AI-ready
AI in action: Six use cases that succeed
with data integrity
AI applications were previously reserved for automation and predictive analytics. With generative AI (GenAI), they push the boundaries of new, imaginative use cases, enabling the creation of content, ideas, and data that can significantly enhance your competitive advantage. In an October 2023 study by Fortune/Deloitte, 79% of CEOs said, “Accelerating innovation is one of the top use cases for implementing GenAI.”
A wealth of new possibilities can finally be realized through intuitive, natural language-based access to corporate data – making previously unattainable use cases a reality.
Browse the six use cases
Solve Top AI Challenges with Data Integrity
Incomplete data, compliance struggles, a lack of context for AI outputs … do these challenges and others like them feel familiar to you? If so, the good news is that they’re all solvable.
To reap the many benefits of AI, like the ones we’ve covered above, you can take a proactive approach by investing in robust, tailored data integrity capabilities. Let’s explore common challenges in more detail.
The challenge
Narrow, biased results
Incomplete datasets and separate data infrastructure stacks limit an AI’s understanding of the questions it’s asked and produce biased, unreliable results.
Because enterprise data is generated and stored in various legacy systems, not all relevant and critical data may be available on the (cloud) platform where AI is run. Lack of access to a complete view of data can result in bias.
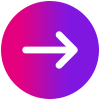
The solution
Data integration
Integrating siloed data and bringing it to where your AI applications run is key to getting unbiased and trustworthy results. This ensures all relevant data is available where AI needs it in a comprehensive, complete, and timely fashion.
The challenge
Untrustworthy results
Inaccurate predictions and recommendations from your AI lead to a lack of trust and can potentially prevent further adoption of these technologies. These are some of the consequences of poor data quality.
The demand for data that’s accurate, consistent, and fit for purpose by AI applications is driving a rethinking of the data quality domain. Without reliable data, advanced AI models are of little use. The stakes are high, and overcoming data quality roadblocks has become a priority.
Additionally, governments across the globe have been stepping up efforts to ensure compliance with regulations aimed at protecting data privacy, which puts more pressure on businesses to comply with data sovereignty laws.
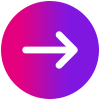
The solution
Data quality and governance
AI initiatives require a new approach to data quality to ensure they’re using data that’s accurate, consistent, and fit for purpose. This will often come in the form of core data quality and business rules, automated validation and cleansing, and integration with data observability and data governance solutions.
Proactive data quality tools can monitor data pipelines, use advanced ML techniques to quickly identify anomalies and outliers, and employ AI to recommend or create rules that ensure issues are remediated before they reach downstream systems.
Data governance provides a clear understanding of how the data you use in AI applications is:
- Collected: what are the data types?
- Stored: where is it located?
- Used: who has access to it?
This unified understanding helps you enforce policies and procedures that protect your data.
You need a proactive approach to data security and compliance to protect sensitive data, safeguard your reputation, and get the most out of AI applications. Implementing robust data governance measures and staying current with the latest regulations is critical.
The challenge
Lack of contextual relevance
Without context into the nuances and dependencies of a given real-world scenario, the AI bases its inference or recommendations on only a small portion of the bigger picture. This can lead to incomplete, inaccurate, or contextually irrelevant results with potentially dangerous downstream impacts.
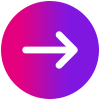
The solution
Spatial analysis and data enrichment
For more accurate and contextualized AI outputs, you must enrich the data that fuels them with trusted third-party data and spatial insights. For instance, you may want to combine address details and environmental risk factors with your property portfolio data for more accurate predictive modeling and natural catastrophe insights.
This process requires a thorough spatial analytics and data enrichment strategy to map your data to real-world scenarios accurately. With your AI and ML models trained on accurate and relevant data, you’ll be ready to produce bigger and better outcomes.
For AI success, these challenges must be addressed to ensure data integrity. We’ll explore that next by discussing three key data integrity considerations for trusted AI.
Three Data Integrity for AI Considerations with Precisely and AWS
Many executives consider integrating new technologies into their business models a top strategic priority – and the focus is on transforming their infrastructure through AI.
However, AI applications based on probability tend to hallucinate, with varying results based on how the prompt was engineered. Comprehensive data integrity can go a long way in building trust.
Training your AI applications with accurate, consistent, and contextualized data is key to reliable results that fuel success. It comes down to three primary data integrity considerations for AI. Here’s what you need to know and how to achieve your desired results with the combined capabilities of Precisely and Amazon Web Services (AWS).
A more complete dataset helps you realize the full potential of your AI
What you achieve with data integrity
Minimize bias, improve accuracy and reliability, and enhance understanding by training AI models with all relevant critical data on-premises, in the cloud, and in hybrid environments. That includes complex data residing on your mainframe.
How you achieve it
Break down data silos and bring fresh data to AWS services – fast – with modern data pipelines from Precisely. Tap into the broadest and deepest functionality for compute and scalable cloud storage with AWS instances optimized for training and inference. By providing your team with cloud resources to derive value from massive datasets, you make AI work for your business.
Fuel your AI applications with trusted data to power reliable results
What you achieve with data integrity
Ensure AI outcomes you can trust. For accurate predictions, recommendations, and effective process automation, models must be trained by data with integrity delivered via cloud-native solutions.
For trusted AI outcomes, your data needs to meet rigorous quality metrics; it needs to be accurate, complete, validly structured, standardized, and free of duplicates. High-integrity data should also be timely, governed using a robust framework, and observed for changes and anomalies.
How you achieve it
When you have transparency into the data lineage in your pipelines, you can improve and observe its quality and govern your data and AI models. You can streamline this process using the Precisely Data Integrity Suite, which runs data integrity processes natively within AWS services across operational and analytical systems like Amazon Redshift. Using data with integrity for model training, deployment, inference, and monitoring makes it easier to build trust in your AI results.
Using trusted data to train and fine-tune your ML and GenAI models in Amazon SageMaker and Amazon Bedrock is essential. With an automated data integrity program, you can understand your data better and automate steps needed to improve its quality. The services of the Precisely Data Integrity Suite provide the required tools to achieve this goal.
When dealing with the high volume of data required to train models, you need a cloud-native approach that scales elastically and cost-effectively. And to ensure continuous data quality, monitoring and proactive anomaly detection are necessary to provide visibility into your entire pipeline. For example, automated alerts can prevent bad data from being sent to Amazon Bedrock or other services for training.
Add context to your data for more relevant and nuanced responses
What you achieve with data integrity
Boost your AI applications’ accuracy and contextual relevance by enriching the data that fuels them with trusted third-party data and spatial insights.
How you achieve it
To accelerate the development of AI applications and increase their adoption, add context to your data that allows the AI to grasp nuances, maintain coherence, and generate contextually relevant responses.
You can achieve this by bringing third-party data and spatial insights from Precisely to your Amazon SageMaker and Amazon Bedrock environments. Curated, authoritative datasets like this add more detail to what you and your AI know about places, people, properties, businesses, and environmental risk factors.
AWS provides a comprehensive set of AI and machine learning (ML) services, infrastructure, and implementation resources to help you at every stage of your AI adoption journey. By building AI applications using high-integrity data and these services, you increase your AI adoption and ensure that your applications are accurate and relevant.
Make your data AI-ready and maximize the potential of your AI-based solutions using the Precisely Data Integrity Suite in tandem with services from Amazon Web Services (AWS).
Precisely and AWS help you gain trusted AI outcomes by bringing together critical data and ensuring it’s of optimal quality — governed by a robust framework, observed to detect degradation, and enriched with essential context derived from spatial insights and third-party datasets.
These considerations ensure your data is of high integrity and that the resulting AI applications can be delivered with complete trust and reliability. Focus on increasing data integrity will accelerate the development and adoption of AI within your organization.
Summary
With the rise of GenAI applications, prioritizing data integrity has never been more crucial.
For AI initiatives that are high-performing, reliable, and produce quality outputs, your data must be complete and accurate, trusted and consistent, and contextualized. This helps you overcome the barriers standing in your way, making it easier to foster trust and adoption, and accelerate AI development and innovation.
What are your top use cases for AI? What could you achieve if you unlock its full potential? Whatever your unique goals, remember that trusted AI starts with trusted data. Future-proof your AI by starting the data integrity journey today.
Data integrity for AI resources
Products
The Precisely Data Integrity Suite
Webinar
AI You Can Trust: Embracing Data Integrity Throughout the Development Lifecycle
Blogs
Trusted Generative AI using Precisely and AWS
Use Data Enrichment to Supercharge AI
The Power of AI in Precisely Software
Tackling Top Data Issues with the Precisely Data Integrity Suite